AI in Domain Search: Innovations and Implications
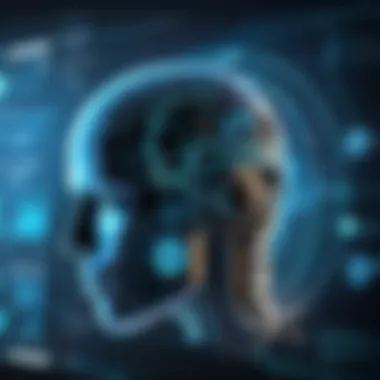
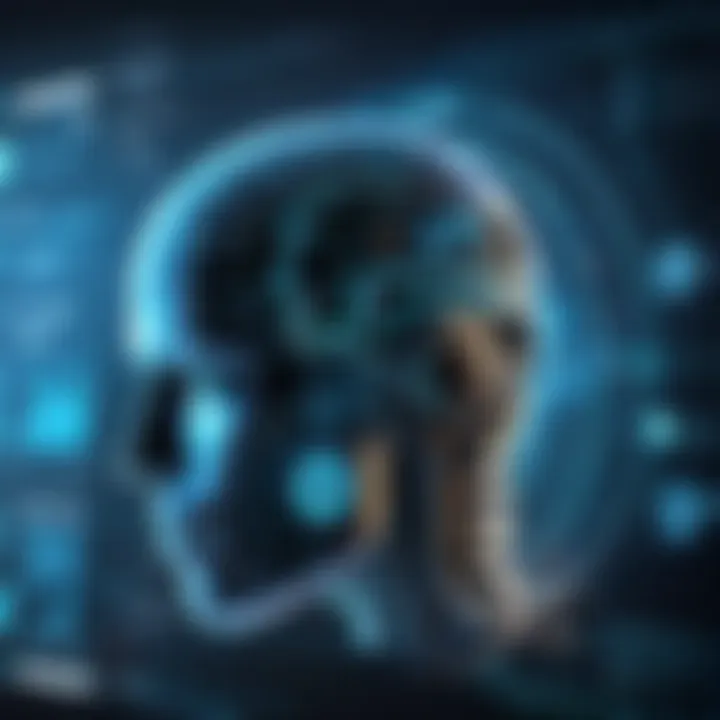
Intro
In todayâs fast-paced digital universe, search technology is more critical than ever. The introduction of artificial intelligence into the domain search landscape creates a complex layer of innovation and potential. Organizations are confronting a myriad of challenges as they integrate AI into their search strategies. This shift not only influences tech companies but also has far-reaching implications for startups looking to carve a niche in the competitive market.
The nuances of AI technology enhance the search experience, offering tailored solutions that promise to redefine how users navigate through vast oceans of information. Whether itâs understanding user intent or sifting through unstructured data, the importance of AI in domain search cannot be overstated. With this exploration, we aim to highlight significant advancements, spotlight emerging startups making waves in this space, and underscore trends and investment opportunities crucial to both entrepreneurs and investors.
Latest Technological Innovations
Overview of the newest high-tech advancements
The realm of AI domain search is evolving rapidly, with innovations transforming how businesses approach search algorithms. Recent breakthroughs include natural language processing and machine learning techniques. These advancements enable systems to interpret user queries with greater accuracy, making search results more relevant and personalized.
Key Innovations:
- Natural Language Processing (NLP): Allows for more intuitive interactions between users and search engines, enhancing the understanding of context.
- Predictive Analytics: AI predicts user behavior, enabling preemptive adjustments to search methodologies.
- Semantic Search: Focuses not just on keywords but also the underlying intent of queries.
Detailed analysis of futuristic technologies and their implications
The implications of these technologies stretch far beyond mere search capabilities. They impact user engagement, data management, and even cybersecurity. For instance, advancements in NLP have paved the way for smarter virtual assistants that can execute complex queries and deliver precise results without extensive user input. Moreover, these technologies foster greater transparency in data usage, crucial for compliance with regulations in an increasingly data-conscious world.
For startups, adopting these innovations might provide a substantial competitive edge. However, organizations must also stay aware of the ethical dimensions, ensuring that the technology does not perpetuate bias or misinformation.
Startup Spotlight
Featured startup company profile and introduction
One company that has positioned itself at the forefront of AI domain search innovation is Verity.ai. This startup focuses on integrating robust AI solutions into domain search applications, creating tools that allow businesses to sift through cloud-based data efficiently.
Startup journey and success story
Founded in 2021, Verity.ai started as a small team of passionate tech enthusiasts who recognized a gap in the market for intuitive search tools. Through strategic partnerships and relentless iterative development, they launched their flagship product last year, which quickly gained traction among several mid-sized companies aiming to enhance their data retrieval capabilities.
Challenges faced by the startup and how they overcame them
Like many startups, Verity.ai faced obstacles, particularly in securing funding and developing a reliable user base. The team navigated these issues by focusing on building a strong product-first approach, which resulted in a loyal customer base that advocated for their tools. Furthermore, their emphasis on transparent communication during the development process resonated well with potential investors, leading to critical funding rounds.
Tech Industry Trends
Examination of current trends shaping the high-tech sector
As AI technology advances, there is a clear shift toward automation and efficiency. The demand for refined search engines is greater than ever, propelling companies forward. Businesses are increasingly prioritizing user experience in their search functionalities, ensuring that users find what they need quickly and effortlessly.
Comparison of different companies' approaches to technological trends
Some companies, like Google and Microsoft, are investing heavily in AI for search optimization, while smaller firms focus on niche applications that cater to specific industries. This creates a landscape where innovation thrives due to a mix of established giants and agile startups.
Predictions for future trends and their potential impact
Looking forward, the rise of AI in domain search is expected to continue shaping how we interact with technology, with greater emphasis on voice search and multimodal search capabilities. The integration of these features will likely influence not only user behaviors but also the strategic directions of businesses engaged in this domain.
Investment Opportunities
Overview of investment prospects in high-tech and startup companies
The AI domain search space attracts various investors keen on capitalizing on groundbreaking technologies. Startups that demonstrate robust growth potential, coupled with innovative solutions, are often at the center of these investment conversations.
Analysis of key factors to consider when investing in the tech industry
Investing in tech requires a keen eye for detail and a deep understanding of market needs. Factors such as the scalability of the technology, the team behind the startup, and their vision make a significant difference in the likelihood of success. Moreover, being aware of ethical implications cannot be overlooked; understanding how a company handles data is vital for long-term viability.
Spotlight on promising startups seeking funding
There are new companies emerging almost daily, making it essential for investors to stay informed. Startups like Lexix.ai are addressing specific issues within the domain search ecosystem, suggesting that as technology advances, there are ample opportunities for investment within niche sectors of this market.
Entrepreneurship Insights
Strategies for navigating the challenges of starting a high-tech business
Starting a tech business comes with its unique challenges. Entrepreneurs should focus on creating a minimal viable product (MVP) that addresses a clear market need. Gathering feedback early and often from users can guide subsequent iterations and ultimately lead to a stronger final product.
Tips for aspiring entrepreneurs to succeed in the fast-paced tech industry
- Stay Informed: Keep up with industry trends and technology changes.
- Network Relentlessly: Build relationships with other entrepreneurs; their insights can be invaluable.
- Adapt Quickly: Be prepared to pivot your business model based on feedback and market demands.
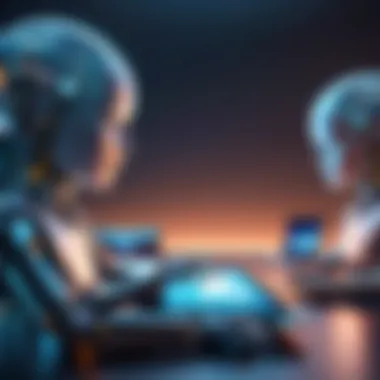
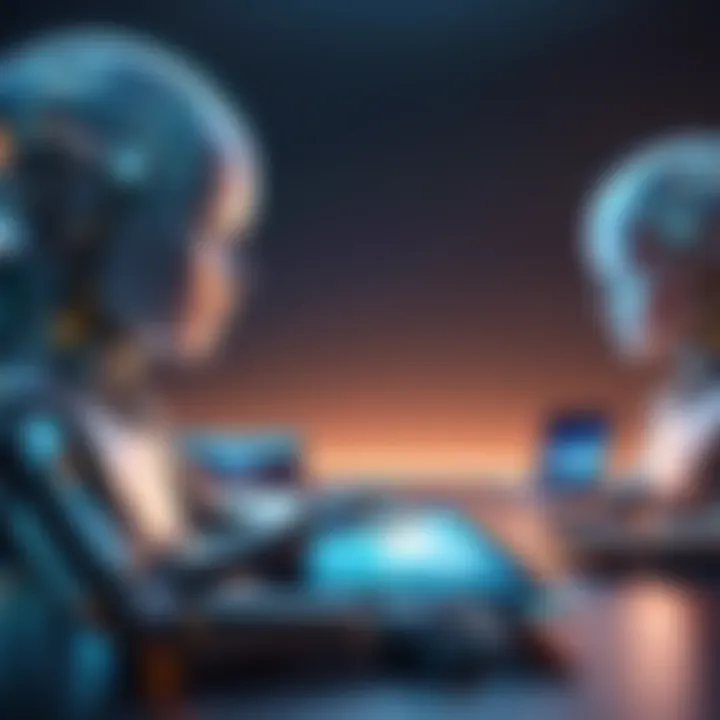
Prelims to AI Domain Search
AI domain search has emerged as a pivotal field, reshaping how technologies curate and provide information. The significance of understanding this area is not just an academic pursuit; it has tangible implications for a variety of industries, especially as businesses increasingly rely on effective data retrieval strategies. In a world characterized by an overabundance of data, having tools that can navigate through it efficiently is not just beneficialâit's essential.
Understanding Domain Search
Domain search refers to the process of identifying and accessing information within specific parameters. It's akin to searching for a needle in a haystack, but with the right tools, the task becomes manageable. Traditionally, search methods relied on keyword matching, which often led to irrelevant results. However, with the advent of AI technologies, particularly machine learning and natural language processing, domain search has evolved. These innovations enable systems to comprehend context, semantics, and even user intent, enhancing both the accuracy and relevance of search results.
Understanding domain search in the context of AI allows us to appreciate its versatility. For instance, in e-commerce, AI can analyze buying patterns and offer personalized recommendations. In knowledge management systems, AI algorithms can sift through documents to provide relevant insights quickly. These applications display not only the utility of AI in refining search capabilities but also its potential to transform business processes.
The Rise of AI in Search Technologies
The rise of AI in search technologies is a response to the increasing complexity of data environments. Organizations face mountains of data that traditional search technologies simply can't handle effectively. AI offers a new frontier, characterized by improved algorithms that continuously learn and adapt from user interactions. Such systems don't merely retrieve data; they understand and anticipate user needs.
As AI technologies have taken root, we have witnessed improvements in several key areas:
- Natural Language Processing: This aspect empowers search engines to interpret user queries in a way that resembles human understanding, thus providing more relevant responses.
- Personalization: AI can tailor search results based on individual preferences and past behaviors, creating a unique search experience for each user.
- Scalability and Efficiency: With the ability to handle vast amounts of data, AI-driven search solutions can operate efficiently across multiple platforms and applications.
"The integration of AI into search technologies is not just a trend; itâs a paradigm shift thatâs reshaping our interaction with information."
Technological Foundations of AI Domain Search
When we delve into the technological foundations of AI domain search, we uncover the core elements that underlie its capability to deliver insightful, relevant, and timely information. This section digs deep into the frameworks, methodologies, and processes that allow AI systems to transform abstract data into actionable insights, enriching search experiences across various domains. When thinking about AI and search, one can't overlook the integral technologies at play; they serve not just as building blocks but as enablers of efficiency and effectiveness.
Machine Learning Algorithms
Machine learning lies at the heart of AI domain search, allowing systems to learn from data and improve over time. Each type of machine learning algorithm approaches problems with unique perspectives and strengths.
Supervised Learning
Supervised learning is a fundamental component in the quest to refine search accuracy. This algorithm relies on labeled datasets, guiding the machine to recognize patterns and make predictions based on prior examples. Its key characteristic is the ability to learn from past outcomes to improve future results. This is particularly beneficial for AI domain search, as it helps tailor the search experience to user expectations.
The unique feature of supervised learning is its reliance on human-provided labels, which can offer precise guidance when training algorithms. However, it can be resource-intensive due to the need for extensive labeled data, which is often hard to gather, especially in niche markets.
Unsupervised Learning
Unsupervised learning provides a complementary angle, focusing on finding hidden patterns in unlabelled data. This method shines in scenarios where data is abundant, but explicit classification is scarce. The hallmark of this approach is its ability to perform clustering or association analysis, bringing forth insights that might have otherwise remained obscured. In AI domain search, unsupervised learning helps systems discover new trends in user behavior.
While unsupervised learning can reveal invaluable insights, its unique featureâlack of definitive outputâposes challenges in interpretability. Results can sometimes feel like a shot in the dark, leading to surprises that may not align with user needs or expectations.
Reinforcement Learning
Reinforcement learning takes a different path by simulating a trial-and-error approach. The algorithm learns to make decisions by receiving feedback through rewards or penalties based on its actions. Its key characteristic is the focus on optimizing long-term outcomes rather than just immediate success. This aspect makes reinforcement learning popular in domains where continuous improvement is possible.
The unique feature of reinforcement learning lies in its adaptability to new environments over time. However, its complexity can lead to longer training periods and significant computational resource requirements, which could be a barrier for some organizations.
Natural Language Processing
Natural Language Processing, or NLP, is central to making AI domain search more intuitive and user-friendly. This technology encompasses various language-based tasks, enabling machines to understand and respond to human language in a meaningful way.
Text Analytics
Text analytics is about extracting useful insights from unstructured data. It plays a vital role in identifying trends and sentiments, making it a key player in domains such as customer feedback and social media monitoring. The key characteristic of text analytics is its capacity to convert raw text into data that can be analyzed quantitatively. This feature makes it a powerful resource for AI domain search.
While text analytics excels in dealing with unstructured information, it often wrestles with contextual ambiguities, which could lead to misunderstandings in user queries.
Semantic Search
Semantic search elevates the search process by considering both the meaning and context of queries. This method goes beyond keyword matching, focusing on user intent and the relationships between concepts. Its key characteristic is this enhanced understanding of language, which leads to results that are not only relevant but also contextually appropriate. Semantic search is particularly advantageous in AI domain search, as it aligns better with how people search for information.
However, the major downside is that developing such complex models often requires substantial time and resources, which might stretch the capabilities of smaller operations.
Contextual Understanding
Contextual understanding further refines the search experience by accommodating the broader context in which a query is made. By analyzing previous interactions and current environment cues, it enables AI systems to offer more tailored results. The key characteristic here is this deeper comprehension of user behavior over time.
Despite its advantages, contextual understanding demands significant data and insights for effective application. If the data lacks representativity, you might find anomalies that skew the intended results.
Neural Networks and AI Models
Neural Networks are advanced learning models that mimic the human brain's architecture. They play a pivotal role in processing complex data structures, enabling AI systems to function more intelligently.
Convolutional Neural Networks
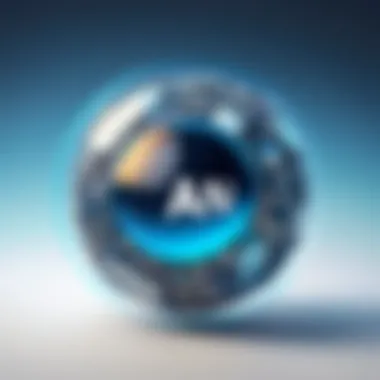
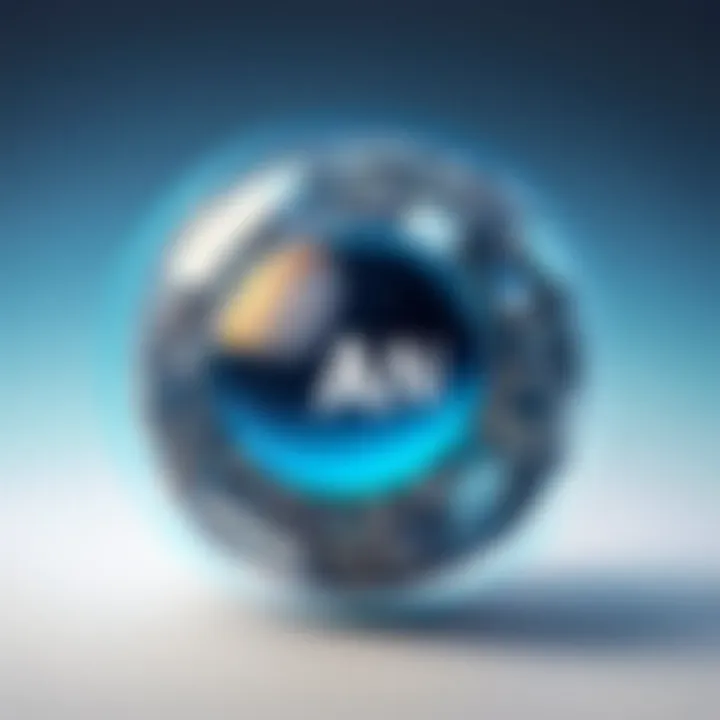
Convolutional Neural Networks (CNNs) are particularly adept at processing grid-like data, such as images. Their capacity to automatically detect patterns makes them a good fit for tasks that involve visual data. The key characteristic of CNNs is their hierarchy of layers, where simple patterns become increasingly complex as data flows through. They can provide substantial benefits in AI domain search where visual data is expected.
However, CNNs may struggle with tasks that require sequential analysis, which limits their use in other nuanced areas.
Recurrent Neural Networks
Recurrent Neural Networks (RNNs) are specifically designed to work with sequential data, which makes them particularly useful in areas like language processing. Their unique ability to maintain a hidden state allows them to remember previous inputs, giving them a sense of context over time. This is crucial for applications such as chatbots and language translation, enhancing the AI domain search's contextual accuracy.
Yet, RNNs can suffer from issues like vanishing gradients, which can hinder learning, especially in long sequences.
Transformers
Transformers represent a significant leap in AI modeling, particularly in processing sequences of data in parallel. They utilize a mechanism called attention that differentiates between relevant and non-relevant parts of the input sequence. Their key characteristic is efficiency in training on large datasets, making them a popular choice for tasks in language understanding and generation.
The primary advantage of transformers is their capability to handle longer contexts effectively. Nonetheless, they require dense computations, which might present challenges for systems with limited computational power.
In summary, each of these technologies and methodologies embodies distinct strengths and weaknesses, contributing to the rich tapestry of AI domain search. Their integration enhances the overall quality of search results, aligning with user expectations while pushing the boundaries of whatâs possible.
Applications of AI in Domain Search
The advent of AI technologies in domain search redefines how information is retrieved and utilized across various sectors. The significance of applying AI in this field cannot be overstated; it not only streamlines operations but also enriches the user experience. With ever-expanding data volumes, intelligent algorithms facilitate faster and more accurate results, making them invaluable in today's digital landscape. Let's delve into specific applications that showcase the extended capabilities of AI in domain search.
E-commerce Optimization
In e-commerce, AI domain search is a game changer. The ability to quickly sift through vast product catalogs and deliver personalized recommendations enhances these platforms. Think about itâwhen a user searches for a running shoe, AI algorithms analyze past behaviors, popular trends, and even seasonality to forecast what a customer might prefer. Moreover, AI can optimize pricing strategies by assessing competitors' prices and consumer demand.
- Personalized Shopping Experience: AI tailors suggestions to individual tastes, often increasing the likelihood of conversion.
- Dynamic Pricing Models: Real-time adjustments based on market trends can help enterprises stay competitive.
- Voice Search Optimization: With the rise of smart assistants, optimizing search capabilities for voice queries is crucial in modern e-commerce.
This application of AI not only drives sales but also informs inventory management and marketing strategies, resulting in a comprehensive approach to business growth in the e-commerce space.
Content Discovery and Recommendation Systems
When it comes to content, whether it's articles, videos, or music tracks, AI significantly enhances content discovery. Traditional search methods might provide generic results. However, AI-driven recommendation systems learn user preferences over time. This leads to a more engaging experience and keeps users returning.
- User Behavior Analysis: Leveraging algorithms to study how users interact with content helps tailor future recommendations.
- Contextual Recommendations: AI can understand the context of a user's search, providing suggestions that are not only relevant but also timely.
- Diverse Content Exposure: By avoiding filter bubbles, AI pushes users to explore varied content that they might never have found on their own.
The more engaging the content delivered, the higher the retention and satisfaction ratesâfactors that are crucial in todayâs online landscape.
Enterprise Knowledge Management
In large organizations, knowledge management becomes critical. AI domain search assists in curating and organizing an organization's intellectual assets. It goes beyond simply retrieving documents; it connects the dots between various pieces of informationâmaking it much easier for employees to locate what they need, when they need it.
- Centralized Knowledge Bases: AI facilitates the creation of a comprehensive knowledge repository that is easily navigable.
- Automatic Tagging and Categorization: By intelligently tagging documents based on content analysis, AI eliminates the tedious manual sorting.
- Enhanced Collaboration Tools: AI can improve tools that enable teams to share knowledge, making collaboration more fluid and fostering innovation.
Through these frameworks, enterprises can harness the extensive data at their disposal, transforming their operational approaches and enhancing organizational efficiency.
"AI-driven solutions are not merely enhancements; they are the linchpins in unlocking the future of domain search across multiple sectors."
Challenges and Limitations of AI Domain Search
In the fast-paced world of technology, understanding the challenges and limitations of AI domain search is crucial. This article helps spotlight these issues in the broader context of AI adoption, especially within startups aiming to take advantage of AI's impressive capabilities. While AI offers many benefits, it also comes with a variety of hurdles that can affect its efficacy. If organizations are aware of these challenges, they can better strategize approaches to mitigate them, leading to better outcomes and innovations.
Data Quality and Availability
One of the most pressing concerns in AI domain search is the quality and availability of data. Simply put, without quality data, the outcomes of AI models can be wildly inaccurate. Garbage in, garbage out is a phrase that perfectly encapsulates this issue. Low-quality or biased data can lead to skewed results in search algorithms. Moreover, if AI systems are trained on incomplete datasets, they may not perform well when faced with real-world data that falls outside the training parameters.
Organizations must prioritize gathering clean and well-structured data. This involves not only collecting vast amounts of data but also ensuring it is relevant and accurate. In many sectors, data is siloed, meaning necessary information is locked within different systems and could be hard to integrate. The effort required to gather and standardize data can delay implementation and reduce potential benefits.
Ethical Considerations in AI
The ethical implications of AI domain search merit closer examination as well. Ethical considerations often prioritize the broader societal impact, and two major aspects stand out: bias in algorithms and privacy concerns.
Bias in Algorithms
Bias in algorithms can lead to unfair or unethical outcomes. When AI systems reflect biases present in their training data, they can perpetuate these biases in output. For example, if an AI system used for job recruitment is trained mainly on data from a particular demographic, it may overlook or undervalue applicants from other groups. This isn't just detrimental to individuals; it could also lead to organizations missing out on diverse talent. The existence of bias symbolizes a considerable challenge in creating fair AI systems, emphasizing the importance of inclusive data collection.
Notably, addressing bias isnât only about correcting data; it also requires continued monitoring and adjustments of algorithms to ensure fairness. As a benefit, reducing bias can enhance the AIâs utility, helping organizations make more accurate and equitable decisions.
Privacy Concerns
In the age of big data, privacy concerns frequently intersect with AI domain search. Organizations harness considerable amounts of personal data to fine-tune their AI models. However, this practice often raises eyebrows regarding user consent and data security. Are consumers fully aware of how their data is being used? The pivotal question lies in balancing the benefits of data collection with the rights of individuals.
Such worries can lead to legal scrutiny and harm a brandâs reputation if not handled properly. Moreover, the integration of AI in search raises questions about transparency. Consumers may be skeptical about how their data is utilized or how search results are modified based on personal data. As a workaround, focusing on strong privacy protocols can bolster consumer trust and lead to more successful AI implementations.
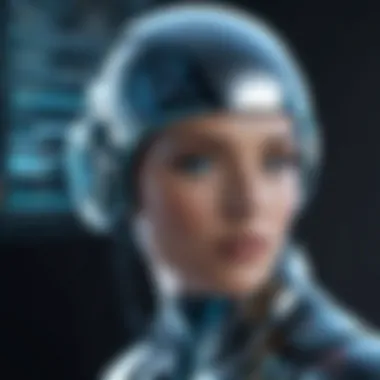
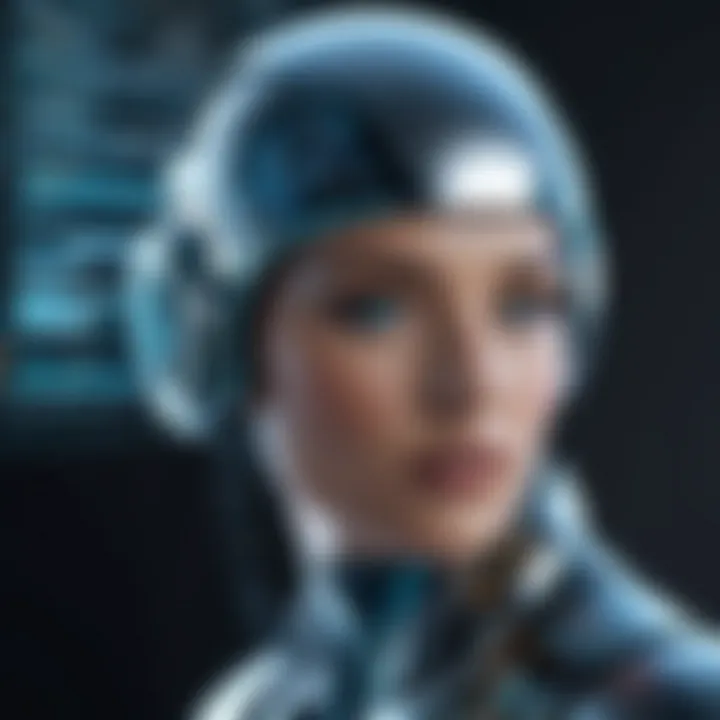
Integration with Legacy Systems
Integrating AI with legacy systems poses another obstacle for organizations. Many businesses have existing infrastructure that predates modern AI technologies. These systems can be outdated, cumbersome, and incompatible with advanced AI solutions, creating friction that could thwart innovation. Organizations that attempt to implement AI without addressing their legacy systemâs limitations may find significant hurdles in data transfer and operational efficiency.
A thoughtful approach to integration involves gradually transitioning from legacy systems while leveraging hybrid solutions. This may require additional investment and planning, but the goal is to ensure that the transition fosters a seamless flow of information and enhances overall system performance. By taking these steps, companies can utilize the best of both worlds â the robustness of established systems and the agility provided by AI technologies.
By recognizing and tackling these challenges head-on, organizations can set themselves up for success when navigating the evolving landscape of AI domain search.
Case Studies of Innovative Startups
The advent of artificial intelligence has been a game changer, particularly for startups aiming to carve out their space in a competitive landscape. By examining case studies of innovative startups, we get a first-hand look at practical applications of AI domain search and the transformative effects it holds not just for technology but for entire industries. Such case studies provide tangible evidence of success and reveal lessons that can guide future endeavors. They highlight the immediate benefits of harnessing advanced algorithms for enhancing search functionalities, personalized experiences, and operational efficiencies.
Success Stories in E-commerce
In the e-commerce sector, startups have massively embraced AI domain search to refine how consumers find and interact with products. A notable example is Stitch Fix, a personalized online clothing service that utilizes AI to match consumers with outfits tailored to their tastes and preferences. By analyzing user data and incorporating machine learning models, Stitch Fix predicts what items will resonate with each customer, driving higher conversion rates.
This process does not just involve basic keyword searches; it includes a nuanced understanding of user behavior and preferences. The algorithm interprets not only what users clicked on but also what they ignore. This dynamic capability results in a more targeted search experience that can significantly increase customer satisfaction and loyalty.
Pioneering Content Platforms
Turning to content discovery, the startup Medium exemplifies how AI domain search can redefine user engagement. Using sophisticated algorithms, Medium analyzes vast amounts of reading data to suggest tailored content that resonates with individual readers. The platform's AI algorithms sift through user interactions to recommend articles, improving user retention and making reading a more personalized experience.
Moreover, Mediumâs innovative tagging system integrates machine learning to categorize content in a way that enhances discoverability and relevance. Writers and readers alike benefit from precise search capabilities, ultimately fostering a thriving content ecosystem.
Disruptive Technologies in Search Solutions
Some startups are harnessing cutting-edge technologies to push the boundaries of traditional search solutions. Take Algolia, a search as a service startup that revolutionizes how developers implement search functionality on their platforms. Algoliaâs tool integrates AI-driven relevance algorithms with real-time analytics, offering lightning-fast responses to user queries.
Their platform stands out because it allows businesses to tailor search experiences with precision. By analyzing user interactions, businesses can adjust their content indexing dynamically. This means that the search experience can evolve based on user feedback, ensuring that the most relevant results are always at the forefront, thereby challenging conventional search methodologies.
Each of these examples showcases how innovative startups are leveraging AI domain search to not just meet consumer expectations, but to exceed them. By examining these case studies, entrepreneurs, investors, and tech enthusiasts can gather invaluable insights on the pathways to successful AI application in search technology.
Future Trends in AI Domain Search
The landscape of AI domain search is undergoing swift transformations. The significance of delving into the future trends in this area ultimately lies in the changing demands of digital information retrieval, coupled with the relentless march of technology. Understanding these future trends will equip organizations with the foresight needed to stay ahead of the curve, embracing new opportunities while mitigating potential risks. This segment paves the way for realizing how emerging technologies and shifts in various sectors can reshape AI-driven search capabilities.
Emerging Technologies to Watch
Quantum Computing
Quantum computing stands out as a game-changer within the realm of computational technology. Its main contribution involves the ability to process complex datasets significantly faster than classical computers. What sets quantum computing apart is its utilization of quantum bits or qubits, which can exist in multiple states at once, rather than being limited to the binary system of traditional bits. This unique characteristic allows quantum computers to perform computations at a scale and speed that were once thought to be infeasible.
The benefits of integrating quantum computing into AI domain search are manifold. For instance, it can enhance data processing speeds, making real-time data analysis not just a possibility but a reality. However, there are some drawbacks, such as the high cost of development and the current limitations of quantum hardware. Organizations must weigh these elements carefully as they explore the potential inclusion of quantum technologies in their AI strategies.
Explainable AI
Explainable AI, often referred to as XAI, plays a crucial role in demystifying complex AI algorithms. Its primary goal is to create transparency in AI decision-making processes. In a space where trust and accountability are paramount, this technology provides an essential avenue for ensuring that users can comprehend how and why specific outputs are generated.
The key characteristic of explainable AI is its focus on creating models that not only perform well but also allow stakeholders to understand the rationale behind outputs. This striking balance is what makes XAI particularly advantageous in sectors like finance or healthcare, where understanding AI decisions can have profound implications.
However, the implementation of explainable AI does come with its fair share of challenges. Crafting models that are both explainable and high-performing can be a delicate balancing act. Despite this, the advantages of deploying XAI, such as heightened user trust and improved compliance with regulations, underscore its importance in the evolving landscape of AI domain search.
Predictions for AI Adoption in Various Sectors
The predictions surrounding AI adoption in various sectors reveal a promising yet complex future. Industries such as finance, healthcare, and retail are increasingly embracing AI technologies to enhance their operational efficiencies and improve user experience.
- Finance: Expect automated risk assessment and advanced fraud detection models to take center stage. AI will help financial institutions analyze historical data and provide insights faster than ever.
- Healthcare: The integration of AI will pave the way for personalized medicine, leveraging vast amounts of patient data to tailor treatments to individual needs.
- Retail: AI-driven customer insights will refine inventory management, creating a personalized shopping experience that engages customers more effectively.
The possibilities are endless, but with them come considerations about ethics, data privacy, and the necessity for regulatory frameworks to guide AI integration.
Recognizing these trends enables businesses to prepare for a future where AI domain search not only evolves but also challenges conventions across industries.
Ending
In a rapidly changing technological landscape, understanding the nuances of AI domain search is crucial. This article has explored the juggernaut that AI has become within this space, raising awareness of its profound implications for various sectors. The intricate web of algorithms, neural networks, and natural language processing collectively owe their advancements to the persistent innovations and strategic applications emerging in startups and established organizations alike.
Summary of Findings
Throughout our exploration, several key highlights emerged:
- Technological Foundations: We comprehended the foundation laid by machine learning algorithms, natural language processing, and other technologies that make AI searches efficient and effective. Each component plays a unique role, from identifying patterns to understanding the context behind queries.
- Applications in Various Sectors: AI domain search is making ripples across many industries, particularly in e-commerce, where it helps optimize product search experiences, and in content discovery, where it enhances user engagement through personalized recommendations. The implications span beyond simple searches, reshaping user expectations and business strategies.
- Challenges Faced: Despite its advancements, the journey is not without bumps. Data quality, ethical considerationsâlike algorithmic biasâand integration hurdles remain daunting challenges that organizations face when diving headfirst into AI. Such challenges must be acknowledged and addressed for AI domain search to mature effectively.
- Innovative Case Studies: The article emphasized startups that have harnessed AI in disruptive and innovative ways. These case studies exemplify how new players are navigating the landscape, often setting the tone for future developments in the field.
Final Thoughts on the Future of AI Domain Search
The future of AI domain search looks promising yet complex. With emerging technologies such as quantum computing on the horizon, we might witness a seismic shift in speed and efficiency. Furthermore, the push for explainable AI ensures that the methods behind suggestions and results become more transparent, bridging gaps of trust among users.
Anticipating changes across sectors, an increasing number of enterprises will likely adopt AI tools to stay competitive. As these tools evolve, they will need to consider ethical ramifications seriously, ensuring that biases don't creep into their algorithms. Organizations that can deftly navigate these watersâbalancing innovation with responsibilityâwill not only thrive but will also set new benchmarks for what AI can achieve in domain search.
"Understanding AI in domain search is not just about technology; it's about re-envisioning user experience and setting a higher standard for what we expect from our digital interactions."