Unleashing the Potential of Amazon Time Series Database: A Guide for Tech Enthusiasts
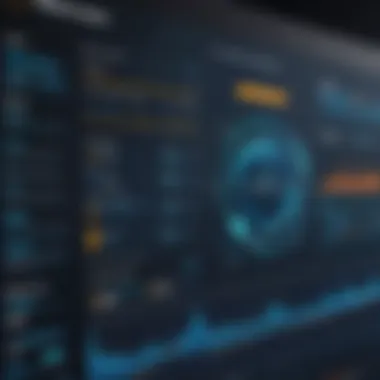
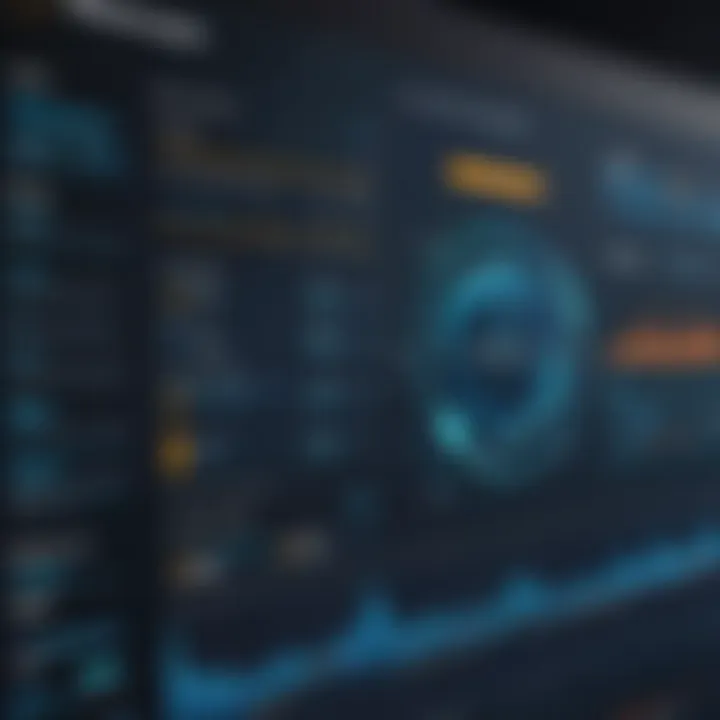
Latest Technological Advances
Delving into the world of Amazon Time Series Database (TSDB) unveils a landscape of cutting-edge technology, where innovation reigns supreme. This section aims to provide a detailed analysis of the latest technological advancements that underpin the high-tech industry. From machine learning algorithms to cloud computing solutions, the realm of high-tech enthusiasts is constantly evolving with new frontiers being explored.
Futuristic Technology Insights
T) devices to advanced data analytics tools, the implications of these technologies are vast. This subsection will delve into the intricate details of how these advancements are revolutionizing the high-tech landscape.
Startup Showcase
In the world of high-tech innovation, startups play a pivotal role in driving change and disruption. This section shines a spotlight on a featured startup that has embraced the potential of Amazon Time Series Database. From their humble beginnings to their remarkable success story, this subsection will offer a glimpse into the journey of the startup, including the challenges they faced and how they overcame them.
The Startup Journey
Exploring the startup's inception and growth trajectory provides valuable insights into the resilience and determination required to thrive in the high-tech sector. By showcasing their innovative approach and strategic decision-making processes, readers can gain a deeper understanding of the entrepreneurial spirit that drives these dynamic companies forward.
Tech Sector Trends
Keeping abreast of the latest trends in the tech industry is essential for high-tech enthusiasts seeking to stay ahead of the curve. This section offers an examination of the current trends shaping the sector, from artificial intelligence developments to cybersecurity protocols. By comparing different companies' approaches to these trends, readers can gain a broader perspective on the industry landscape.
Predicting Future Trends
Anticipating future trends and their potential impact is key to making informed decisions in the tech sector. By analyzing market projections and emerging technologies, this subsection aims to provide valuable insights into the direction in which the high-tech industry is headed. By forecasting upcoming trends, readers can better position themselves to capitalize on future opportunities.
Investment Insights
For investors looking to navigate the high-tech investment landscape, understanding the key factors driving investment decisions is paramount. This section offers an overview of investment prospects in high-tech and startup companies, detailing the risk and reward dynamics at play. By spotlighting promising startups seeking funding, readers can gain valuable insights into potential investment opportunities.
Evaluating Investment Factors
Analyzing the critical factors to consider when investing in the tech industry provides a comprehensive understanding of the risks and rewards involved. From market trends to competitive analysis, this subsection offers a nuanced perspective on the investment landscape. By delving into the intricacies of investment opportunities, readers can make informed decisions that align with their financial goals.
Entrepreneurial Guidance
Navigating the challenges of entrepreneurship in the high-tech industry requires strategic foresight and resilience. This section offers invaluable insights and strategies for aspiring entrepreneurs looking to make their mark in the fast-paced tech sector. By providing tips and guidance on overcoming obstacles and seizing opportunities, this subsection aims to empower readers with the knowledge and tools needed to succeed.
Strategies for Success
Offering practical advice and actionable strategies, this subsection equips aspiring entrepreneurs with the tools to navigate the complex and competitive landscape of the high-tech industry. From choosing the right co-founders to scaling a business effectively, readers will gain valuable insights into the entrepreneurial journey. By distilling key lessons and best practices, this section aims to inspire and guide the next generation of high-tech innovators.
Introduction to Amazon Time Series Database (TSDB)
Amazon Time Series Database (TSDB) holds a critical position in the realm of high-tech applications, where the continuous influx of time-stamped data is indispensable. In this article, we unravel the intricacies of TSDB, shedding light on its pivotal role in handling vast amounts of chronological data efficiently and effectively. Understanding the unique capabilities of Amazon TSDB is paramount for those seeking to harness the power of time series data in their high-tech endeavors.
Understanding Time Series Databases
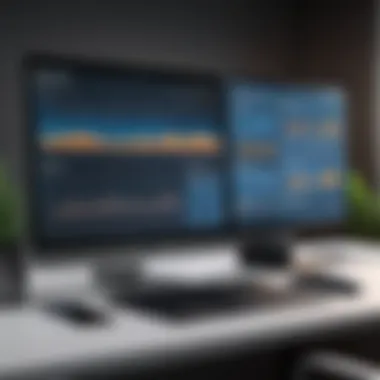
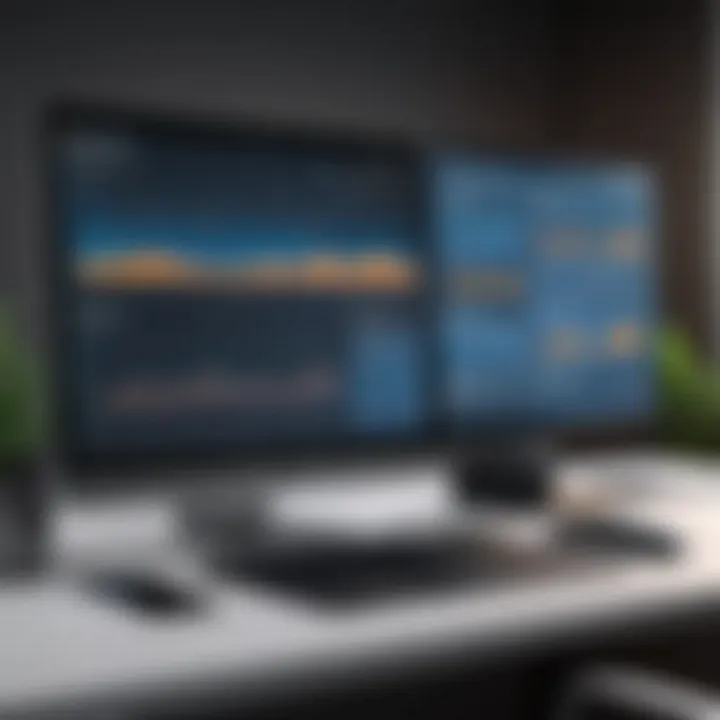
Definition and Concept
Time series databases such as Amazon TSDB are specifically designed to store and analyze data points indexed in chronological order. The inherent structure of time series data facilitates pattern recognition, trend analysis, and forecasting, making it an invaluable asset for high-tech applications. The capability of Amazon TSDB to handle massive amounts of sequential data sets it apart, offering streamlined data management and analysis for users.
Importance of Time Series Data in High-Tech
Time series data plays a pivotal role in high-tech industries by providing crucial insights into trends, anomalies, and performance metrics over time. Its significance lies in enabling organizations to make data-driven decisions based on historical patterns and real-time observations. Leveraging time series data in high-tech applications empowers businesses to optimize processes, enhance predictive capabilities, and drive innovation through deeper analytical insights.
Overview of Amazon Time Series Database
Key Features of Amazon TSDB
Amazon TSDB distinguishes itself with its high-performance data storage and retrieval capabilities, ensuring rapid access to large volumes of time-based data. The database's scalability and reliability make it a preferred choice for applications requiring real-time analytics and monitoring. Furthermore, Amazon TSDB's seamless integration with other AWS services enhances its versatility, allowing users to leverage the full potential of the cloud ecosystem.
Integration with AWS Ecosystem
The integration of Amazon TSDB with the extensive AWS ecosystem provides users with a comprehensive data management solution. By seamlessly connecting with services such as Amazon S3 and Amazon Cloud Watch, TSDB streamlines data processing workflows and simplifies monitoring and analysis tasks. This interoperability enhances the overall efficacy of time series data utilization within the AWS environment.
Advantages of Amazon TSDB
Scalability and Performance
Amazon TSDB's scalability and performance capabilities enable users to handle exponential data growth without compromising query speed or data integrity. The database's efficient indexing and compression techniques optimize storage utilization while ensuring rapid data retrieval. This scalability ensures that high-tech enthusiasts can seamlessly adapt to changing data demands and maintain superior performance levels.
Cost-Efficiency and Data Retention
The cost-efficiency of Amazon TSDB lies in its ability to streamline data storage and processing tasks, reducing operational overheads for high-tech organizations. Additionally, the database's advanced data retention policies enable users to manage data lifecycle effectively, ensuring compliance with retention regulations and optimizing storage costs. By balancing cost-effectiveness with data retention practices, Amazon TSDB offers a compelling solution for long-term data management strategies.
Functionalities and Use Cases
In the realm of Amazon Time Series Database for high-tech enthusiasts, the section on Functionalities and Use Cases serves as a vital exploration of the practical applications and benefits of this technology. Understanding the functionalities of Amazon TSDB is crucial for users to leverage its capabilities effectively. By delving into the various use cases, readers can grasp the diverse scenarios where Amazon TSDB proves invaluable.
Data Ingestion and Retrieval
Real-Time Data Ingestion
Real-Time Data Ingestion plays a pivotal role in ensuring that time-sensitive information is captured and processed instantaneously within Amazon TSDB. This aspect enables users to seamlessly stream data in real-time, ensuring up-to-the-moment accuracy and responsiveness. The key characteristic of Real-Time Data Ingestion lies in its ability to process incoming data without delay, making it a popular choice for applications requiring immediate insights and actions. Its unique feature of capturing and storing data as it is generated enhances decision-making processes, although maintaining real-time ingestion scalability can pose challenges.
Flexible Data Retrieval Options
Flexible Data Retrieval Options in Amazon TSDB empower users to tailor their data extraction methods according to specific requirements. This flexibility allows for customized data queries, facilitating nuanced analysis and insights. The key characteristic of Flexible Data Retrieval lies in providing varied retrieval mechanisms to accommodate diverse data needs seamlessly. Its unique feature of offering multiple retrieval options enhances user experience, yet striking a balance between flexibility and query optimization can present complexities.
Monitoring and Analytics
Visualizations and Dashboards
Visualizations and Dashboards within Amazon TSDB present data in a visually intuitive manner, aiding users in comprehending complex data sets efficiently. The key characteristic of Visualizations and Dashboards is their ability to transform raw data into interactive visuals, making trends and patterns more accessible. This feature is a popular choice as it enhances data interpretation and decision-making processes significantly. However, designing effective visualizations that convey precise insights without clutter can be a challenge.
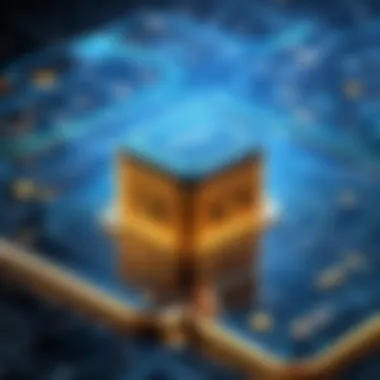
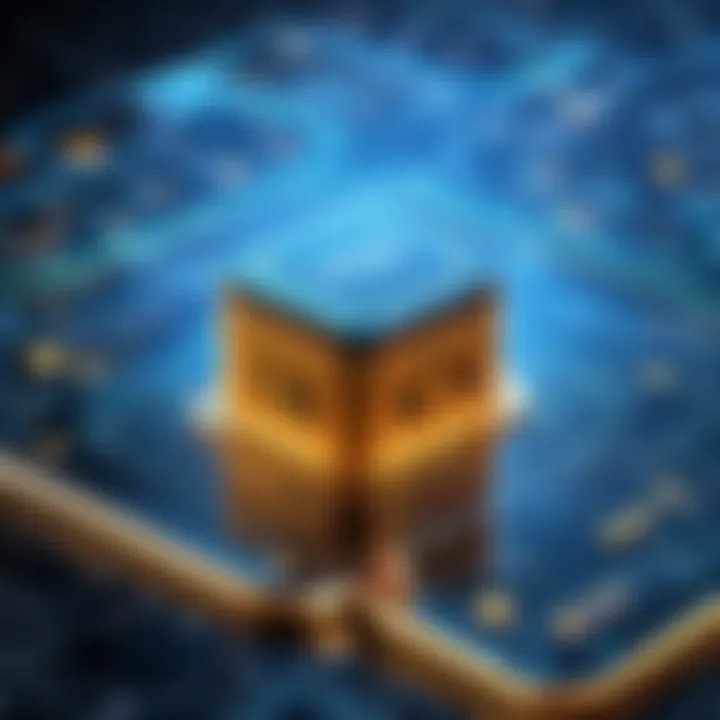
Anomaly Detection Capabilities
Anomaly Detection Capabilities in Amazon TSDB equip users with the capacity to detect deviations or abnormal occurrences within time series data. This functionality is crucial for identifying potential issues or irregularities promptly, minimizing operational disruptions. The key characteristic of Anomaly Detection is its real-time monitoring and alerting system, enabling proactive intervention in critical situations. Its unique feature of identifying anomalies automatically enhances operational efficiency, though fine-tuning detection thresholds for accuracy may require meticulous calibration.
Predictive Maintenance and Io
T Applications
Streamlining Io
T Data Processing
Streamlining Io T Data Processing in Amazon TSDB optimizes the handling of IoT-generated data, ensuring seamless integration and processing of vast volumes of information. The key characteristic of Streamlining IoT Data Processing lies in its ability to manage diverse data streams efficiently, enabling scalable IoT applications. This feature is a beneficial choice for organizations relying on IoT devices for real-time insights and decision-making. However, ensuring data accuracy and consistency across multiple IoT sources can present data integrity challenges.
Enhancing Predictive Analytics
Enhancing Predictive Analytics within Amazon TSDB enriches predictive modeling capabilities using time series data, enabling more accurate forecasting and decision support. The key characteristic of Enhancing Predictive Analytics is its ability to leverage historical data to predict future trends and outcomes with precision. This functionality is a popular choice for organizations seeking data-driven insights to enhance operational efficiency. Yet, ensuring the reliability and validity of predictive models through continuous data refinement requires rigorous analysis and validation processes.
Integration and Compatibility
Integration and compatibility are pivotal aspects when it comes to the utilization of Amazon Time Series Database (TSDB) in high-tech environments. By seamlessly integrating with other AWS services, Amazon TSDB ensures a cohesive ecosystem for data management and analysis. Compatibility with various tools and platforms further enhances its versatility and applicability, allowing for streamlined operations and efficient utilization of resources. The ability of Amazon TSDB to integrate with Amazon S3 and Cloud Watch presents notable advantages for scalability, performance monitoring, and data retention in this digital landscape.
AWS Services Integration
Seamless Integration with Amazon S3
Seamless Integration with Amazon S3 plays a crucial role in enabling efficient data storage and retrieval processes within the AWS framework. This feature allows Amazon TSDB to seamlessly interact with Amazon S3 buckets, facilitating secure and scalable storage solutions for time series data. The seamless integration ensures a smooth flow of data between Amazon TSDB and S3, contributing to enhanced data accessibility and reliability. Its ability to handle large volumes of data with low latency makes it a preferred choice for high-tech applications, underscoring its significance in this article.
Compatibility with Amazon Cloud
Watch Compatibility with Amazon Cloud Watch offers essential monitoring capabilities for Amazon TSDB users, empowering them to track performance metrics, set up alerts, and gain valuable insights into system behavior. This compatibility enables seamless collaboration between Amazon TSDB and CloudWatch, streamlining the monitoring process and ensuring timely identification of anomalies or issues. The real-time monitoring features provided by Amazon CloudWatch enhance the overall data management experience, making it a valuable asset for high-tech enthusiasts leveraging Amazon TSDB in their operations.
Third-Party Tool Integration
Utilizing Grafana for Visualization
Utilizing Grafana for Visualization amplifies the data visualization capabilities of Amazon TSDB, allowing users to create interactive and insightful dashboards for performance analysis. Grafana's user-friendly interface and extensive customization options make it a popular choice for visually representing time series data, catering to the diverse needs of high-tech users. The seamless integration of Grafana with Amazon TSDB enhances data visualization, offering a comprehensive view of critical metrics and facilitating informed decision-making in high-tech environments.
Integrating with Prometheus for Monitoring
Integrating with Prometheus for Monitoring extends the monitoring capabilities of Amazon TSDB, enabling users to track system health, performance trends, and infrastructure metrics effectively. Prometheus's robust monitoring features, including time series data collection and alerting mechanisms, complement the data management functionalities of Amazon TSDB. This integration provides a holistic approach to monitoring high-tech systems, ensuring proactive identification and resolution of potential issues. The seamless collaboration between Amazon TSDB and Prometheus enriches the monitoring landscape, offering advanced monitoring solutions for high-tech enthusiasts.
Security and Compliance
In the dynamic landscape of Amazon Time Series Database (TSDB), the aspect of Security and Compliance holds paramount importance. Security and Compliance are foundational pillars that ensure the integrity, confidentiality, and availability of data within the Amazon TSDB ecosystem. By adhering to stringent security protocols and regulatory compliance standards, users can mitigate risks and safeguard sensitive information. Security measures play a critical role in thwarting cyber threats and unauthorized access, bolstering the overall trust and reliability of the TSDB platform. Compliance, on the other hand, ensures alignment with industry regulations, enhancing transparency and accountability in data management practices.
Data Encryption and Access Control
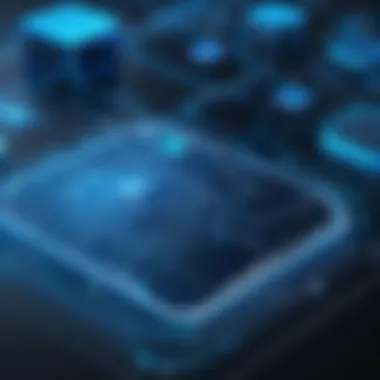
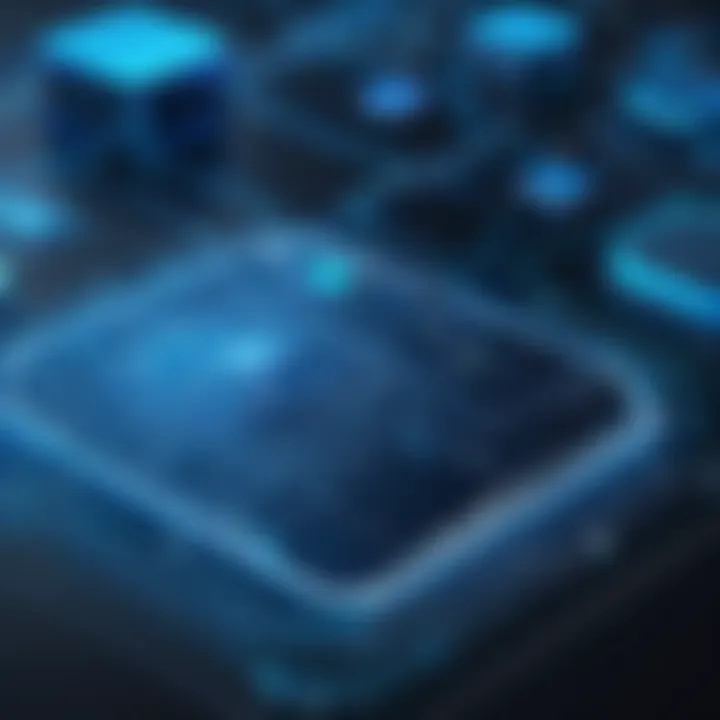
TLS Encryption for Data Transfer
In the realm of data encryption within Amazon TSDB, Transport Layer Security (TLS) Encryption for Data Transfer emerges as a linchpin of secured communication. TLS encryption functions as a cryptographic protocol that encrypts data during transmission, shielding it from interception or tampering. This robust encryption mechanism fortifies data-in-motion, guaranteeing confidentiality and integrity across communications within the TSDB infrastructure. TLS Encryption not only secures sensitive data transfers but also upholds data privacy standards mandated by stringent compliance regulations. Its distinctive attribute lies in its ability to establish secure communication channels, mitigating the risks of data breaches and unauthorized interceptions.
Fine-Grained Access Policies
When delving into access control mechanisms, Fine-Grained Access Policies stand out as a pivotal instrument for regulating data accessibility within Amazon TSDB. These policies offer granular control over user permissions, delineating precise levels of access based on roles and responsibilities. By defining intricate access privileges and restrictions, Fine-Grained Access Policies institute a robust security framework, curtailing unauthorized data exposure and manipulation. The nuanced nature of these policies empowers organizations to enforce strict data governance protocols, ensuring that only authorized users can interact with specific datasets. While enhancing data security, Fine-Grained Access Policies might necessitate meticulous configuration and maintenance, potentially increasing administrative overhead but yielding unparalleled data protection benefits.
Compliance Standards
GDPR Compliance Measures
Within the realm of data protection regulations, General Data Protection Regulation (GDPR) Compliance Measures play a pivotal role in upholding user privacy rights and data sovereignty. GDPR mandates stringent control over personal data processing, providing individuals with transparency and control over their information. By adhering to GDPR Compliance Measures, Amazon TSDB promotes data privacy and accountability, aligning its operations with European data protection standards. The core characteristic of GDPR lies in its emphasis on data subject rights and lawful processing criteria, enhancing user trust and data governance within the TSDB infrastructure.
HIPAA Regulations Adherence
In the domain of healthcare data management, Health Insurance Portability and Accountability Act (HIPAA) Regulations Adherence serves as a vital framework for safeguarding patients' medical information. HIPAA regulations impose strict guidelines on the handling of electronic protected health information (e PHI), ensuring confidentiality and integrity in healthcare data transactions. By complying with HIPAA Regulations, Amazon TSDB reinforces data security measures, particularly in managing time series data sourced from IoT devices in the healthcare sector. The key attribute of HIPAA lies in its focus on privacy, security, and breach notifications, fortifying data protection mechanisms and promoting trust among healthcare stakeholders.
Future Implications and Innovations
In this pivotal section of the article, we delve into the future implications and innovations surrounding Amazon Time Series Database (TSDB). In the ever-evolving landscape of technology, understanding the direction in which innovations are headed is crucial for high-tech enthusiasts. By exploring future implications, we navigate through the potential advancements and game-changing developments that Amazon TSDB can bring to the table.
One of the key elements to consider in future implications is how Amazon TSDB can integrate with machine learning (ML) models. By enhancing ML models with time series data, we open up a realm of possibilities for predictive analytics and deeper insights. This integration empowers high-tech enthusiasts to make more informed decisions based on real-time data trends and patterns. Leveraging the continuous streams of time-stamped data, ML integration can optimize processes, detect anomalies, and forecast future scenarios with precision.
Furthermore, enabling predictive insights through Amazon TSDB is another significant aspect to explore. By utilizing the rich historical data stored in TSDB, users can generate forecasts and predictions that drive strategic decision-making. This feature not only enhances operational efficiency but also provides a competitive edge in adapting to market trends and customer behaviors. Predictive insights derived from time series data amalgamated with advanced algorithms propel businesses towards proactive measures and futuristic strategies.
Machine Learning Integration
Enhancing Models with Time Series Data
Diving into the realm of enhancing ML models with time series data unveils a transformative approach to data analysis. By incorporating time series data into ML algorithms, high-tech enthusiasts can extract valuable nuances from temporal patterns and trends. This fusion optimizes model performance by enabling algorithms to learn from past data points dynamically. The ability to process time-stamped data empowers ML models to adapt to changing scenarios in real-time, enhancing predictive accuracy and decision-making processes.
Key characteristics of enhancing ML models with time series data involve the seamless integration of historical data series and predictive modeling. This integration facilitates the identification of correlations, seasonal variations, and trend forecasts, amplifying the predictive power of ML algorithms. The continuous flow of time series data improves model learning, leading to more accurate predictions and insightful interpretations. However, this approach requires robust data preprocessing techniques and adept model tuning to harness the full potential of time series insights.
Enabling Predictive Insights
Enabling predictive insights through ML integration marks a paradigm shift in harnessing the potential of time series data. By leveraging predictive analytics, high-tech enthusiasts can extrapolate future trends and patterns from historical time series data. This approach enables organizations to proactively address challenges, seize opportunities, and optimize resource allocation based on anticipated outcomes.
The key characteristic of enabling predictive insights is the forward-looking perspective it offers to decision-makers. By forecasting future scenarios and trends, businesses can strategize effectively, mitigate risks, and capitalize on emerging market dynamics. Predictive insights empower high-tech enthusiasts to stay ahead of the curve, anticipate consumer demands, and tailor servicesproducts accordingly. However, it is essential to validate predictive models regularly, monitor data quality, and adapt strategies based on evolving trends for sustained accuracy and relevance.
AI-Driven Decision-Making
Incorporating AI Algorithms for Analysis
Incorporating AI algorithms for analysis revolutionizes decision-making processes by infusing intelligence into data interpretation. High-tech enthusiasts can leverage AI-driven analysis to uncover hidden patterns, extract valuable insights, and optimize operational efficiencies. By integrating AI algorithms, organizations can automate analytical tasks, detect anomalies, and enhance data-driven decision-making capabilities.
The key characteristic of incorporating AI algorithms for analysis lies in the cognitive prowess they bring to data processing. AI algorithms sift through vast datasets, recognize complex patterns, and generate actionable recommendations, augmenting human decision-making with machine intelligence. This symbiotic relationship between AI and human cognition streamlines processes, reduces manual intervention, and accelerates insights delivery. However, maintaining data integrity, ensuring algorithm transparency, and validating AI outcomes are paramount for cultivating trust in automated decision-making processes.
Automating Decision Processes
Automating decision processes through AI integration streamlines workflows and enhances organizational agility. By automating routine decisions, high-tech enthusiasts can allocate resources efficiently, respond promptly to dynamic conditions, and optimize business operations. The autonomous nature of decision automation mitigates human bias, expedites response times, and scales decision-making capabilities across diverse functions.
The key characteristic of automating decision processes is the scalability and consistency it brings to operational frameworks. AI-driven decision automation maintains decision-making quality across repetitive tasks, minimizes errors, and frees up human capital for strategic initiatives. The precision and speed of automated decision processes empower organizations to adapt swiftly to market fluctuations, customer demands, and internal requirements. However, implementing robust governance frameworks, monitoring algorithm performance, and aligning automation with organizational goals are essential for ensuring successful deployment of AI in decision-making contexts.