Exploring Monte Carlo Valuation Techniques in Finance
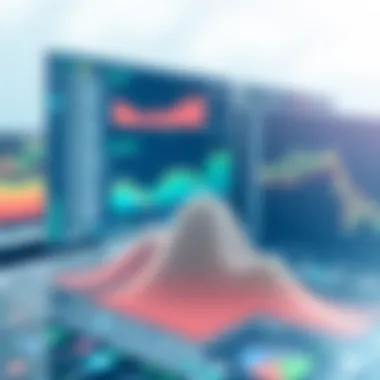
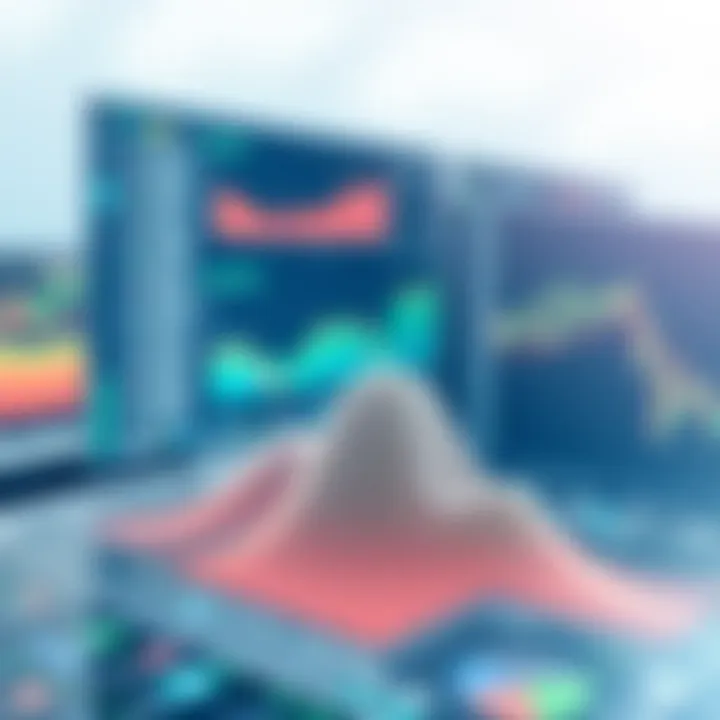
Intro
Monte Carlo valuation techniques have carved a notable niche in the landscape of financial modeling. These methods stand apart by simulating a multitude of potential outcomes through randomness and statistical modeling. At their core, Monte Carlo methods help practitioners quantify uncertainty in their financial forecasts, no small feat in a world where the only constant seems to be change.
Why is it that Monte Carlo techniques are capturing the attention of both startups and established tech firms alike? It comes down to their adaptability and accuracy, especially in complex environments where traditional valuation methodsâoften linear and deterministicâfall short. This article explores how these innovative techniques are reshaping financial analyses, particularly within high-tech industries and among nascent startups.
But before diving into the nitty-gritty, letâs grasp the broader picture of current advancements that make these techniques ever more relevant.
Latest Technological Innovations
In the fast-paced realm of finance, newer technologies continuously emerge, fundamentally altering how professionals approach valuations. Among the most significant advancements, machine learning algorithms and big data analytics have transformed how Monte Carlo methods are applied.
Overview of the newest high-tech advancements
- Cloud Computing: The power of cloud-based computing has rendered Monte Carlo simulations accessible to a broader audience. No longer confined to those with supercomputers, smaller startups leverage cloud platforms for running complex models at a fraction of traditional costs.
- Artificial Intelligence: AI is infusing a dose of intuition into statistical modeling. By optimizing the parameters and refining the models, AI enables better predictions and faster computations, enhancing the applicability of Monte Carlo techniques.
Detailed analysis of futuristic technologies and their implications
Now, consider the fusion of these high-tech tools. When machine learning and Monte Carlo simulations intertwine, they fuel a revolution. For instance, predictive analytics can help identify patterns in the vast oceans of data generated by consumers or marketsâan insight-rich stream often termed as big data. This dual approach not only enhances precision in forecasts but also tailors strategies that resonate with the evolving market landscape.
Quote: "The combination of Monte Carlo methods and AI is not just about more calculations; itâs about a paradigmatic shift in how we perceive risk and opportunity."
As we begin to unpack the depth of Monte Carlo valuation techniques, it is crucial to understand the driving forces behind their success.
Why Monte Carlo?
Unlike traditional models that often rely on a handful of assumptions, Monte Carlo valuation techniques embrace variability. They offer a roadmap through uncertain financial futures by leveraging random sampling, hence providing a spectrum of potential outcomes rather than a single output.
Understanding these foundational concepts provides a strong footing as we journey through the intricacies of how these valuation techniques apply in dynamic environments, from high-tech startups to established enterprises.
With context set, we can now briskly move into the complexities of how different sectors adopt and implement Monte Carlo valuation techniques.
Prolusion to Monte Carlo Valuation
Monte Carlo valuation techniques have revolutionized the landscape of financial modeling and risk assessment. Their importance cannot be overstated, especially in today's volatile markets where uncertainty reigns. Monte Carlo simulations allow analysts to embrace this uncertainty instead of shying away from it. By employing random sampling and statistical analysis, they create a realistic picture of potential outcomes, leading to more informed decision-making.
One could think of Monte Carlo valuation as a sophisticated crystal ball, where instead of peering into the future with blind optimism, analysts get a nuanced view of what could realistically happen. This approach is particularly critical when evaluating complex financial instruments or investments, where traditional methods may fall short.
It's also worth noting that the versatility of Monte Carlo methods extends beyond finance. They have found their way into various sectors, from insurance to pharmaceuticals, demonstrating their broad applicability and relevance.
Key Benefits of Monte Carlo Valuation:
- Risk Measurement: Provides a detailed understanding of the risks associated with a particular financial decision.
- Scenario Analysis: Enables the evaluation of multiple scenarios simultaneously, capturing a wide range of potential outcomes.
- Enhancement of Traditional Models: A powerful complement to established valuation techniques like Discounted Cash Flow (DCF), helping to bridge gaps in those methods.
However, it's important to approach Monte Carlo valuation with a clear framework. Analysts must carefully set parameters and assumptions to avoid misleading results. By doing so, they tap into the full potential of this method.
Thus, the following sections will delve deeper into the definition, historical context, and the bedrock principles of Monte Carlo valuations â because understanding these elements is crucial for anyone looking to leverage this mighty analytical tool.
Fundamental Principles of Monte Carlo Simulation
Monte Carlo Simulation serves as a cornerstone in the world of quantitative finance, offering a robust methodology to tackle uncertainty in complex valuation scenarios. The principle behind it is quite straightforward: by using random sampling, one can simulate a wide array of outcomes, thereby providing a comprehensive view of potential future states. This is particularly pertinent in financial modeling where traditional predictions may fall short. With a dynamic approach to analyzing risks and forecasting potential returns, understanding the fundamental principles of Monte Carlo techniques is imperative for anyone looking to navigate the nuances of today's complex financial landscapes.
Random Sampling Techniques
At the heart of Monte Carlo methods lies the concept of random sampling. This technique involves generating a multitude of random numbers, which correspond to different variables influencing the outcomes of a model. There are various methods for implementing random sampling, such as:
- Simple Random Sampling: This is the most basic approach. Each scenario is equally likely to occur, making it relatively easy to execute.
- Stratified Sampling: In cases where certain outcomes are more likely than others, stratified sampling can enhance outcomes by dividing data into different segments, or strata, ensuring that each strata is appropriately represented.
- Latin Hypercube Sampling: This is a more sophisticated technique that provides more even coverage of the possible outcomes compared to simple random sampling. It helps maintain a balanced representation across multiple dimensions, which can be particularly useful in high-dimensional models.
By utilizing these techniques, practitioners can create a more accurate picture of risks and expected returns. The robustness of random sampling allows users to understand the range of possible outcomes and identify significant risk factors that might otherwise go unnoticed. This process is integral to risk management and decision-making in complex financial environments.
Probability Distributions and Their Importance
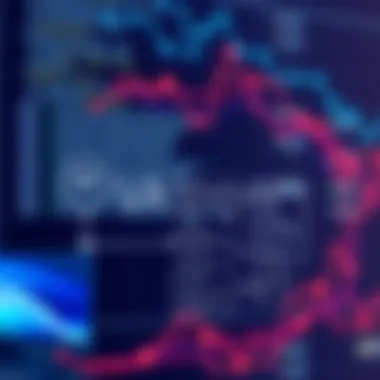
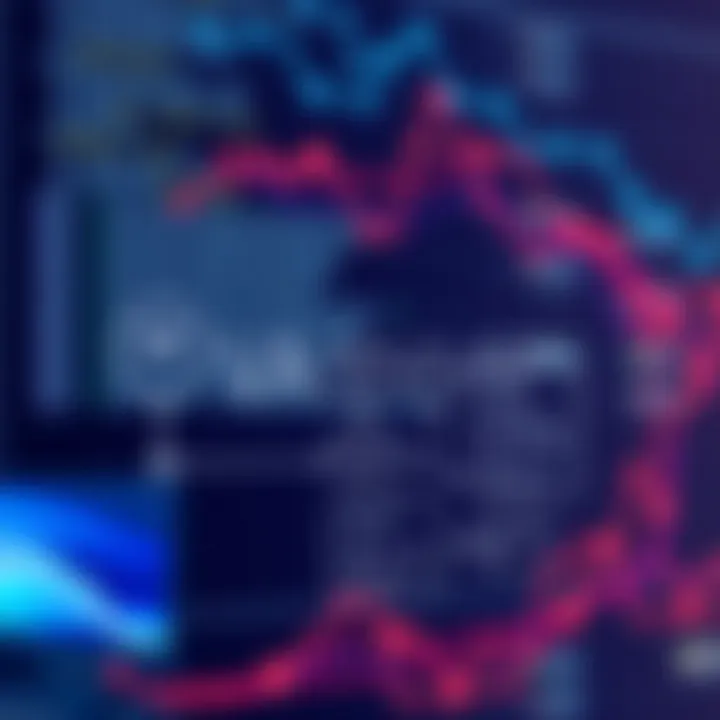
Probability distributions are the backbone of Monte Carlo simulations, influencing how random samples will generate results. Different types of distributions apply depending on the nature of the underlying risks. Here are some common distributions used:
- Normal Distribution: Ideal for modeling many financial variables that cluster around a mean, such as stock returns.
- Log-Normal Distribution: Useful for variables that cannot take negative values, such as asset prices.
- Exponential Distribution: Often used to model time until an event occurs, like default risk.
The choice of distribution significantly impacts the simulation's outputs. For instance, if you inaccurately assess the underlying distribution, it could lead to erroneous conclusions about the risks involved. Thus, understanding the relevance and characteristics of different probability distributions can greatly affect the efficacy of Monte Carlo approaches.
In harnessing the power of these principles, Monte Carlo Simulation provides a nuanced understanding of financial landscapes, aiding in decision-making processes that capitalize on calculated risks.
Applications of Monte Carlo Valuation
The use of Monte Carlo valuation techniques spans across various fields, deeply enriching areas such as finance, risk management, and project valuation. These techniques allow practitioners to model complex systems and to simulate a vast range of outcomes that are tied to uncertain variables. Understanding the applications of Monte Carlo valuation not only highlights its value but also illustrates its versatility in tackling real-world problems that demand nuanced analytical approaches.
Financial Asset Pricing
In finance, the pricing of assets can be a challenging endeavor due to uncertainties in market conditions and investor behaviors. Monte Carlo valuation provides a robust framework for determining the fair value of financial instruments.
Instead of relying solely on static models that could be misleading, Monte Carlo simulation considers a host of future scenarios by generating a multitude of potential outcomes based on stochastic processes. For example, when assessing an option, analysts can establish a range of possible prices at expiration, varying underlying asset prices, interest rates, and volatility. This helps in expressing the value of the option as a distribution, which provides a clearer picture of its potential performance.
Key benefits of employing Monte Carlo for financial asset pricing include:
- Flexibility: It can adjust to incorporate new information, market conditions, and tailored simulations.
- Risk Assessment: By evaluating myriad outcomes, investors gain insights into potential risks and rewards, paving the way for informed decision-making.
- Comprehensive Valuation: Unlike traditional approaches, it captures the inherent risks associated with market dynamics, allowing for a more rigorous valuation framework.
Risk Management Strategies
Robust risk management is vital for any organization navigating uncertain environments. Monte Carlo valuation plays a substantial role in this arena, allowing firms to simulate various risk scenarios and assess their potential impacts.
Using Monte Carlo simulations, businesses can analyze complex models that account for multiple risk factors. For instance, in portfolio management, decision-makers can estimate the potential losses under different market conditions by generating thousands of potential outcomes. This simulation not only aids in visualizing the worst-case scenarios, but it also assists in capital allocation and risk mitigation planning.
Some critical considerations include:
- Scenario Analysis: The ability to develop various economic scenarios leads to better preparedness for potential downturns.
- Regulatory Compliance: Many industries, particularly in finance, are bound by regulations that require effective risk assessment strategies. Monte Carlo aids in satisfying these compliance demands.
- Optimized Decisions: By revealing the probabilities of different outcomes, businesses can fine-tune their strategies to minimize financial exposure.
Project Valuation in Startup Ecosystems
Startups operate in a world filled with uncertainties, making project valuation distinctly challenging. Monte Carlo valuation techniques offer a promising solution to these challenges, allowing entrepreneurs to estimate the value of their projects by examining a series of potential developments.
For example, when raising funds, a startup may utilize this technique to present a range of possible returns to investors, depicting both optimistic and pessimistic scenarios based on various market and operational factors. This transparency creates a narrative that enhances investor confidence, potentially leading to favorable financing terms.
In particular, the advantages in startup ecosystems are:
- Dynamic Projections: Monte Carlo valuations can adjust as new data emerges, ensuring that projections remain relevant.
- Attracting Investors: By illustrating the probabilities of success, startups may appeal more to investors who are drawn to data-driven insights.
- Risk Justification: Entrepreneurs can justify their funding requests by presenting comprehensive simulations that account for both risks and opportunities.
"Monte Carlo valuation is not merely a method; it's an essential pillar for making sense of uncertainty in finance and project management."
In summary, the applications of Monte Carlo valuation are vast and varied, impacting critical aspects of finance, risk management, and project evaluation in startups. Its ability to model uncertainty makes it a treasure for those seeking to navigate the complex landscape of contemporary economics.
Comparison with Other Valuation Methods
In the world of finance and investment analysis, various valuation methods exist, each with its own unique set of advantages and constraints. The comparison of Monte Carlo valuation with other methods is essential for understanding its distinctive value proposition and applicability across different scenarios. By analyzing Monte Carlo alongside established techniques like Discounted Cash Flow (DCF) and Real Options Valuation, we can discern the nuances that make Monte Carlo a preferred choice under specific conditions.
Monte Carlo vs. Discounted Cash Flow (DCF)
At first glance, Discounted Cash Flow (DCF) and Monte Carlo simulation may appear similar; both seek to estimate the present value of future cash flows. However, the methodologies diverge significantly in approach and context.
The DCF method relies heavily on deterministic inputs. It estimates future cash flows based on assumed rates, considering the time value of money through a single discount rate. While DCF provides a straightforward snapshot of a project's potential return, it tends to oversimplify reality. It often assumes a level of predictability that is rarely found in volatile markets or emerging industries.
On the other hand, Monte Carlo simulation injects randomness into the estimation process. By employing thousands of simulations, it captures a range of possible outcomes, allowing for a more comprehensive risk assessment. This method acknowledges that variables such as market conditions and project timelines are inherently uncertain.
For example, if an investor uses DCF to value a tech startup that projects rapid growth, they might input optimistic cash flow estimates without considering potential market disruptions. In contrast, Monte Carlo simulation allows investors to create a probability distribution based on different scenariosâassessing the impact of both favorable and unfavorable events on the startupâs valuation.
"Monte Carlo simulation illustrates the unpredictable dance of variables, while DCF clings to the script with fixed choreography."
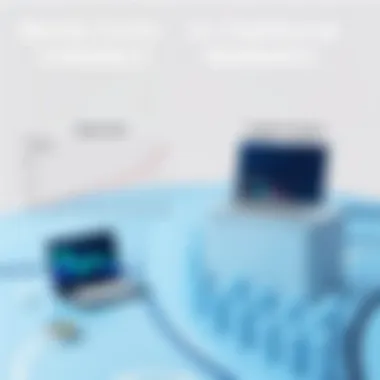
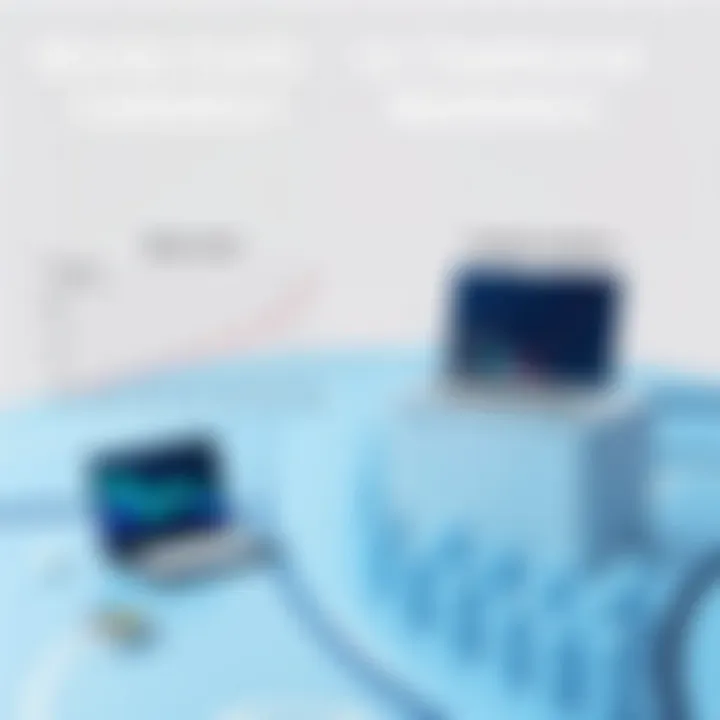
In summary, while DCF can be effective for stable and established businesses, Monte Carlo stands out for projects with high uncertainty or where multiple scenarios need evaluation. It's essentially about picking the right tool for the job.
Monte Carlo vs. Real Options Valuation
Both Monte Carlo and Real Options Valuation (ROV) are advanced methods used to evaluate the potential of investments under uncertainty. Real Options Valuation expounds on traditional discounted cash flow techniques by considering the value of managerial flexibility and the ability to make decisions in response to market changes.
Whereas DCF treats cash flows in a linear fashion, ROV recognizes the right (but not the obligation) to undertake certain business decisionsâsuch as deferring, expanding, or abandoning a project. This dynamic approach is particularly useful in industries characterized by significant investment risks, such as biotechnology or fuel exploration.
Monte Carlo simulation, in contrast, does not focus primarily on managerial decisions. Instead, it provides a statistical understanding of project potential across various scenarios. By integrating simulation, analysts can explore an overwhelming number of outcomes. This diversity of results can aid decision-making by revealing which options have higher probabilities of success and which variables are the most consequential.
In practice, integrating both methods often yields the most insightful results. For instance, in valuing a pharmaceutical project, ROV might identify the option to expand clinical trials as desirable, while Monte Carlo simulation estimates the range of successful outcomes based on varied trial results. The confluence of these analyses can thus paint a clearer picture of both potential returns and associated risks.
Challenges in Implementing Monte Carlo Valuation
Monte Carlo valuation techniques bring a wealth of opportunity for insights and sophisticated analysis, but like any powerful tool, they come with their own set of challenges. These hurdles can be significant for practitioners aiming to leverage this method in their financial modeling and risk management endeavors. Let's delve into the key challenges faced during implementation, demonstrating why overcoming these barriers is crucial for effective usage.
Data Quality and Availability
One of the foremost considerations in any valuation exercise is the availability and quality of data. In the context of Monte Carlo simulations, having accurate and relevant input data directly influences the results. Poor quality data can lead to erroneous probabilities, skewed outcomes, or even a complete misrepresentation of risks. It can be a real conundrum, especially in topics like financial forecasting, where the stakes are high.
- Data integrity is a must: Ensure that the historical data used for simulations is not just plentiful, but also precise. This requires scrutinizing the data sourcesâwhether they be market data, historical financial performance, or estimates of future trends.
- Sampling biases can creep in if the data isn't representative of possible future outcomes. Practitioners must be vigilant about maintaining a diverse dataset that captures a broad array of potential scenarios.
Availability can also be problematic. Not all necessary data might be accessible due to regulatory restrictions or proprietary proprietary concerns. Special attention should also be given to data timelinesâoutdated data can mislead even the most meticulous analysis, as markets and their dynamics can evolve rapidly.
Computational Efficiency
Next up is computational efficiency. Monte Carlo simulations inherently require a lot of computations because they involve running thousands, sometimes millions, of random samples to derive results. This can be taxing on hardware, especially for smaller firms or startups that may lack high-performance computing resources. Here are a few points of consideration:
- Hardware limitations: Organizations need robust infrastructure to support the sheer volume of data processing. Investing in high-performance computing might be justified considering the reliability and speed it can offer.
- Algorithm optimization: Using well-optimized algorithms plays a crucial role in running simulations efficiently. Poorly written code can lead to bottlenecks, when all the complex computations take forever to execute.
- Parallel processing: Some models ease the computational burden by allowing parallel processing to run multiple simulations at once. Understanding how to harness this can minimize execution time significantly.
Alleviating computational inefficiency is vital. A streamlined process not only saves time but also enables a faster interpretation of results, allowing for agile decision-making.
Interpreting Results
Interpreting the results from a Monte Carlo simulation can also be quite the puzzle. Itâs not just about crunching numbersâunderstanding what those numbers mean in terms of risks and opportunities is key. A few aspects are vital:
- Statistical literacy is essential. Practitioners should be comfortable navigating standard deviations, confidence intervals, and other statistical measures that come into play during the interpretation phase. It's far too common for stakeholders to misinterpret these outputs, drawing faulty conclusions from what is essentially probabilistic data.
- Visualization tools can aid significantly. Graphs and charts that illustrate the distribution of possible outcomes or the risk landscape can translate complex outputs into easier-to-understand formats. This helps in communicating reliable insights to teams or clients who may not have a deep statistical background.
- Scenario analysis: Interpretation isnât just about the average outcome, but rather the tail events and what they reveal. A good approach is to perform sensitivity analyses to gauge how different variables affect outcomes. Not every scenario holds equal weight, after all.
In summary, while Monte Carlo valuation techniques possess distinctive strength in forecasting and risk assessment, one must tackle these challenges head-on to harness their potential fully. Without addressing issues like data quality, computational abilities, and interpretation strategies, even the smartest simulations can falter in delivering the clarity and insights that informed decision-making requires.
The Role of Technology in Monte Carlo Valuation
In the ever-evolving landscape of finance, technology acts as an enabler, transforming how we approach valuation techniques like Monte Carlo methods. By harnessing advancements in computing and software, practitioners can perform simulations with remarkable accuracy and efficiency. This section sheds light on key technological elements, their benefits, and considerations that define the modern use of Monte Carlo valuation.
Advancements in Computational Power
The surge in computational power over the last couple of decades cannot be understated. Modern processors and multi-core architectures have significantly reduced the time it takes to run complex simulations.
- Parallel Processing: One of the game-changers in computational power is parallel processing. By allowing multiple computations to occur simultaneously, investment analysts can model thousands or even millions of scenarios in a fraction of the time that was once necessary.
- Graphics Processing Units (GPUs): Initially designed for rendering graphics, GPUs are now widely employed for numerical computations. Their architecture is particularly well-suited for the simultaneous execution of multiple tasks, which is the very essence of Monte Carlo simulations. The transition from CPUs to GPUs in many applications has led to a reduction in computation times from hours to mere minutes.
Such advancements have made it feasible to include more complex variables and scenarios, enriching the insights gained from simulations. As a result, investors can make more informed decisions, ultimately enhancing return on investment.
Software Tools for Monte Carlo Analysis
The next crucial element to consider is the variety of software tools available for Monte Carlo analysis. These tools have made sophisticated techniques accessible, even to those with less mathematical expertise. Here are a few notable options:
- Matlab: Renowned for its powerful numerical computing capabilities, Matlab offers built-in functions for running Monte Carlo simulations. Itâs widely used in finance for risk analysis and portfolio management.
- R and Python: Both programming languages have become essential in the toolkit of data analysts. Libraries like , , and in Python, or and in R, facilitate the creation of custom Monte Carlo models tailored to specific needs.
- Specialized Software: Platforms such as @Risk and Crystal Ball provide user-friendly interfaces designed specifically for risk analysis. These tools allow users to build simulations visually, making it easier to communicate results to stakeholders without deep technical knowledge.
"Technology doesnât just change the way we work; it revolutionizes our understanding of risk and rewards in finance."
Choosing the right tool often depends on the complexity of the problem at hand. However, the integration of software and technology into Monte Carlo valuation techniques has leveled the playing field. It enables not only seasoned analysts but also newcomers in the field to leverage simulation methods effectively.
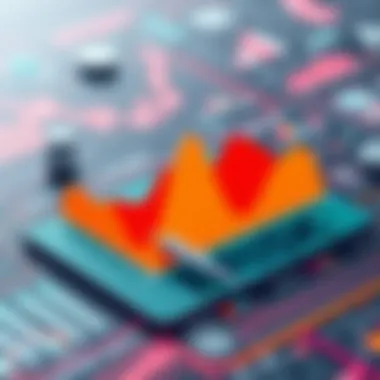
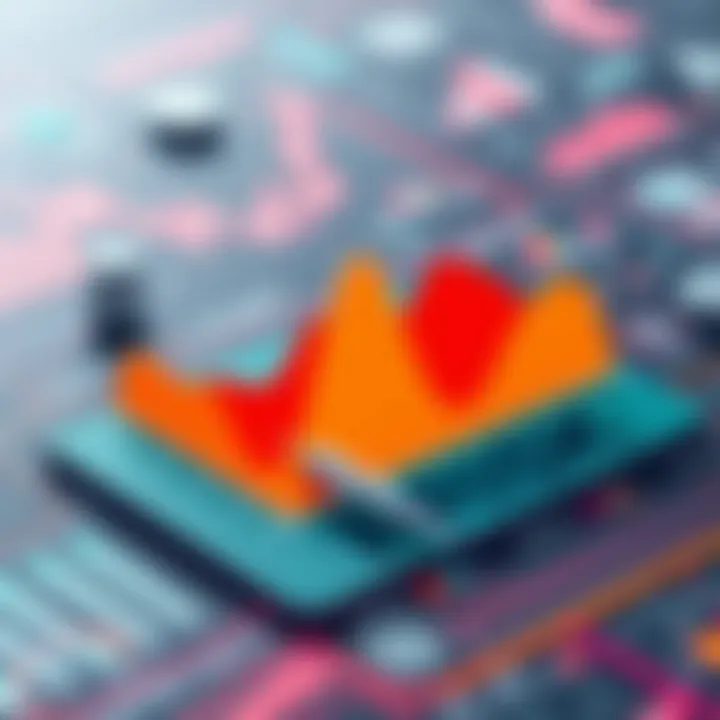
Best Practices for Monte Carlo Valuation
Utilizing Monte Carlo valuation techniques can be a game changer in financial modeling. However, like any powerful tool, their efficacy largely hinges on the right practices during implementation. There are several key points to keep in mind when engaging with these methods. Adhering to best practices not only increases the accuracy and reliability of your results but also enhances your ability to communicate insights derived from the simulations. Here's a deeper dive into what those practices entail.
Setting Appropriate Parameters
When it comes to Monte Carlo simulations, choosing the right parameters can make a world of difference. Parameters, such as the number of trials or the specific distributions used, act as the backbone of your analysis.
- Defining the Scope: Be clear on what exactly you aim to analyze with your simulation. This clarity will guide the selection of both data inputs and boundaries, ensuring you're focused on achieving meaningful results.
- Number of Simulations: There is a fine balance between computational load and result accuracy. While it's tempting to run hundreds of thousands of simulations for more granularity, one must consider the time and resources at hand. A general rule is to run enough trials to converge on stable results, often somewhere around 10,000 to 50,000 runs for many financial models.
Choosing too few simulations can lead to misleading outcomes, like mistaking noise for significant trends. Careful parameter selection sets the stage for your analysis and offers clarity down the line.
Validating Simulation Results
After the simulations wade through the data, validating those results becomes paramount. It's not just about running numbers; it's about making sure those numbers actually mean something in the real world.
- Cross-Validation Techniques: Employ various validation techniques to confirm the integrity of your results. For example, split your dataset into training and testing sets to see if the model reliably performs on unseen data. Cross-validation helps ensure that your findings are replicable.
- Scenario Analysis: Create scenarios to see how your results hold up under different assumptions. This helps identify the robustness of your findings, as certain parameters may skew results drastically.
- Back-Testing Against Historical Data: Compare outcomes from your simulations to historical data to check for consistency and accuracy. While past performance doesn't guarantee future results, it does provide a benchmark for assessing the fidelity of your model.
- Document Everything: As trivial as this might sound, keep a comprehensive record of your simulation process, from parameter selection to result interpretation. This not only aids in transparency but also allows you to retrace your steps if something doesnât add up.
"In finance, what is not documented is often as good as non-existent."
Through careful parameter setting and diligent validation processes, you can unlock the true value of Monte Carlo simulations. The journey doesnât end at obtaining results; it extends into interpreting them effectively for decision-making purposes.
Future Trends in Monte Carlo Valuation
As the financial landscape continues to evolve, the development of Monte Carlo valuation techniques remains a cornerstone for sophisticated analytics in diverse sectors. The future of these methods is invigorating, showcasing a growing influence of emerging technologies, particularly in artificial intelligence and high-tech industries. Understanding these trends can empower practitioners to harness the full potential of Monte Carlo simulations, enhancing decision-making processes and strategic planning.
Integration with Artificial Intelligence
The confluence of Monte Carlo techniques and artificial intelligence (AI) is garnering significant attention. AI can augment Monte Carlo simulations by enhancing the accuracy of input data and refining models. By utilizing machine learning algorithms, practitioners can sift through vast datasets to identify patterns that might otherwise go unnoticed. For example:
- Data Analysis: AI can preprocess data, ensuring higher quality input which leads to more reliable output. Traditional methods often struggle with noisy or incomplete data, but machine learning techniques help clean up these irregularities.
- Predictive Modelling: By integrating AI, simulation models can evolve to generative networks. These networks not only simulate different scenarios but can also predict potential outcomes based on historical trends and real-time data.
"The fusion of artificial intelligence with Monte Carlo simulation represents a shift from traditional perception to predictive insight, offering practitioners a clearer picture of uncertainty and risk."
Overall, the integration of AI into Monte Carlo valuation techniques can help companies gain a competitive edge, enabling them to make strategic decisions based on a blend of predictive analytics and robust risk assessment.
Emerging Applications in High-Tech Industries
High-tech industries are ripe for taking advantage of Monte Carlo valuation techniques, where flexibility and adaptability are paramount. Here are some noteworthy applications:
- Software Development: In agile environments, Monte Carlo simulations assist in project management by predicting timelines and potential pitfalls, helping teams allocate resources more effectively.
- Cryptocurrency and Blockchain: The volatile nature of crypto assets benefits from Monte Carlo simulations as they allow for extensive risk assessments and portfolio optimization, vital in managing exposure to market fluctuations.
- Healthcare Technologies: With the advent of personalized medicine and biotechnology, simulations can help model complex biological systems and predict treatment outcomes based on diverse patient data, guiding investment strategies and policy making.
As we move forward, it becomes increasingly clear that Monte Carlo valuation is not simply a tool of the pastâit's shaping the future of analytics in pioneering sectors. With ongoing advancements and a broadened scope, its role in tech-driven industries is only set to increase, making it indispensable for savvy investors and decision-makers.
Culmination
The conclusion of an article on Monte Carlo valuation techniques wraps up the discussion by highlighting critical insights and future implications. Itâs crucial because it distills complex information into digestible main points, ensuring that the reader walks away with a clear understanding of the concepts presented. Hereâs what we take away from this exploration:
Summarizing Key Insights
Monte Carlo valuation techniques have proven their worth in various financial modeling scenarios, especially in risk analysis and asset pricing. Key insights that emerged from this discussion include:
- Adaptability: These techniques can be tailored for different sectors, accommodating varying levels of risk and uncertainty. This flexibility makes them highly relevant as industries evolve.
- Robustness against Complexity: Unlike traditional methods, Monte Carlo simulations effectively handle complicated, non-linear processes that are common in modern financial landscapes.
- Transparency in Risk Assessment: They provide explicit quantification of potential risks, something that is crucial for stakeholders in any high-stakes environment.
Critically, despite their efficacy, implementing Monte Carlo methods is not without hurdles. Practitioners must ensure robust data quality and comprehend the intricacies of interpreting simulation results. This balancing act can prove challenging but, ultimately, rewarding.
Looking Ahead to Future Developments
As technology continues to evolve, the future of Monte Carlo valuation techniques appears bright. Some anticipated developments include:
- Integration with Artificial Intelligence: As AI becomes more prevalent, we can expect enhanced predictive capabilities. Machine learning algorithms could significantly refine the accuracy of input variables, thus improving simulation fidelity.
- New Applications Across Industries: We may see more industries adopting these methods to tackle uncertainties, from health tech needing to gauge treatment efficacy to renewable energy assessing project viability.
- User-Friendly Software Tools: The growing demand for intuitive interfaces suggests that more accessible software will emerge, democratizing these advanced techniques for smaller firms and individual investors.
In summary, as we peer into the distance, the adaptability and sophistication of Monte Carlo valuation methods will likely elevate their status further in financial analysis and beyond.
"Monte Carlo simulations not only help in making informed decisions but also contribute to building a clearer picture of what the future may hold in financial landscapes."
Thus, keeping an eye on how these valuation techniques evolve will be instrumental for tech-savvy entrepreneurs and investors alike.