Exploring Large Language Models in Banking
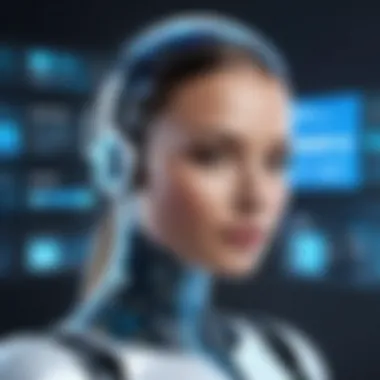
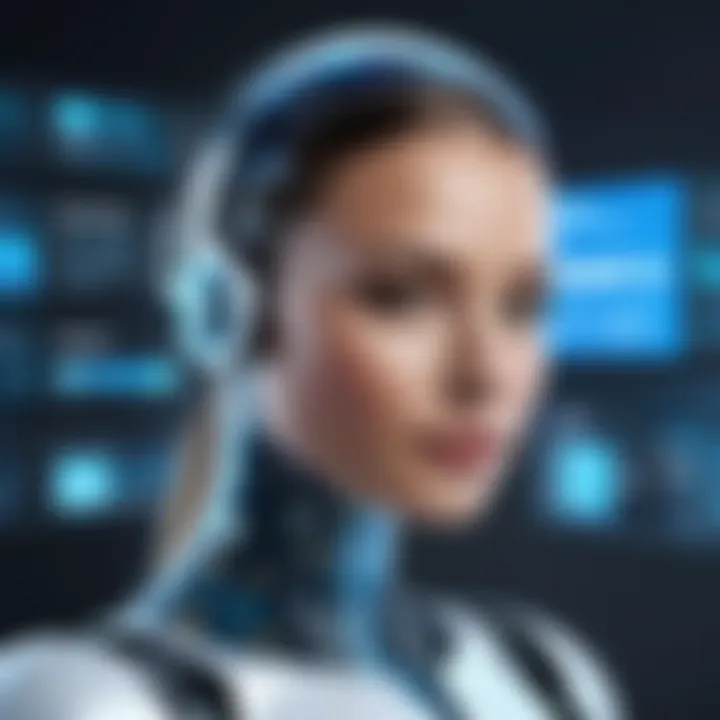
Intro
Large Language Models (LLMs) have started to reshape several sectors, with banking being one of the most notable domains experiencing tangible transformations. In a world where speed, efficiency, and accuracy are paramount, LLMs offer solutions that can streamline operations and improve customer experiences. This article explores the myriad ways in which LLMs are integrated into the banking sector, outlining their real-world applications, benefits, challenges, and ethical considerations.
Today’s competitive landscape in banking demands that institutions leverage innovation. Large Language Models facilitate enhanced data analysis, regulatory compliance, and customer service solutions. Each application drives the evolution of banking by harnessing the power of artificial intelligence. As this technology advances, it brings both opportunities for improvement and challenges that must be carefully managed.
Understanding these dynamics is crucial for bankers, tech enthusiasts, and investors alike. As LLMs continue to develop, their role will likely expand and adapt to meet the changing needs of the financial sector.
Latest Technological Innovations
The advancements in technology relevant to LLMs are broad and impactful. These innovations include increased data processing capabilities, natural language understanding improvements, and more sophisticated machine learning algorithms.
Key recent developments include:
- Transformer Architecture: The basis for most modern LLMs, allowing for improved context understanding.
- Transfer Learning: This technique utilizes pre-trained models to apply knowledge to new but related tasks.
- Reinforcement Learning: This can further enhance the model’s ability to interact intelligently.
Each of these innovations plays a pivotal role in shaping the future of banking operations. Institutions can deploy LLMs to streamline processes that previously required extensive human intervention.
Beyond operational efficiencies, these transformations have broader implications for customer relationship management. Institutions may not only anticipate customer needs but also provide personalized support through chatbots and automated systems, making banking more accessible.
Challenges in Integration
While the merits of LLMs are evident, their integration into established banking systems is not without its complications. Some challenges include:
- Data Security: Handling sensitive customer information requires stringent safeguards.
- Regulatory Compliance: Banking regulations must adapt to the capabilities of AI, ensuring that automation complies with existing laws.
- Ethical Considerations: The potential for bias in decision-making processes raises questions about transparency and fairness in financial services.
These hurdles require careful planning and adaptability from banking institutions to navigate the fast-evolving landscape.
"As we advance in LLM utilization, the ethical implications will be a crucial part of the conversation, affecting public trust in banking technologies."
Future Implications
Looking ahead, LLMs are poised to redefine banking. The convergence of AI with traditional banking functions will likely lead to more innovative customer services and decision-making processes in risk management. Institutions that embrace these changes sooner rather than later will find themselves at the forefront of this digital evolution.
In summary, Large Language Models are not merely a trend; they signify a deeper transformation within the banking industry. Their integration promises enhanced efficiency, better customer service, yet raises significant challenges that must be addressed thoughtfully. As we explore the role of LLMs in banking, we must keep a keen focus on the implications for the sector at large and the ethical considerations that arise.
Preface to Large Language Models
In recent years, the banking sector has witnessed a transformative shift due to the integration of advanced technologies. One of the most pivotal innovations in this area is the emergence of large language models (LLMs). These models utilize complex algorithms to process and generate human-like text, thereby reshaping how banks interact with clients and manage vast amounts of data.
Definition and Overview
Large language models are a subset of artificial intelligence that interpret and produce written language. Unlike traditional models that rely on predefined rules, LLMs learn from extensive datasets, capturing nuances of language, context, and subject matter. Their ability to understand and generate text means they can engage in complex dialogues, answer queries, and provide information efficiently. For example, models like OpenAI’s GPT-3 can offer recommendations based on customer inquiries or provide summaries of lengthy reports, enhancing the overall user experience.
The Evolution of LLMs
The evolution of LLMs reflects significant advancements in machine learning and computational power. Early natural language processing (NLP) focused primarily on rule-based systems with limited flexibility. However, the introduction of deep learning techniques marked a notable departure from these methods.
- Early Models: Initial models, such as n-grams, analyzed fixed sequences of words, struggling to comprehend context.
- Neural Networks: The development of neural networks allowed for more sophisticated text generation, thus improving language comprehension.
- Transformers: Later, the transformer architecture, introduced in the paper "Attention is All You Need," revolutionized NLP by enabling models to focus on relevant parts of the text.
The application of transformers led to the creation of substantial LLMs, which are now pivotal in sectors like finance. Banks harness this technology to streamline operations, ensuring accurate information retrieval and processing.
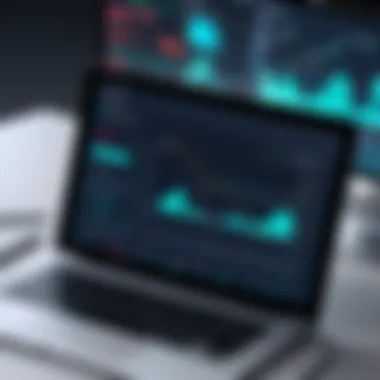
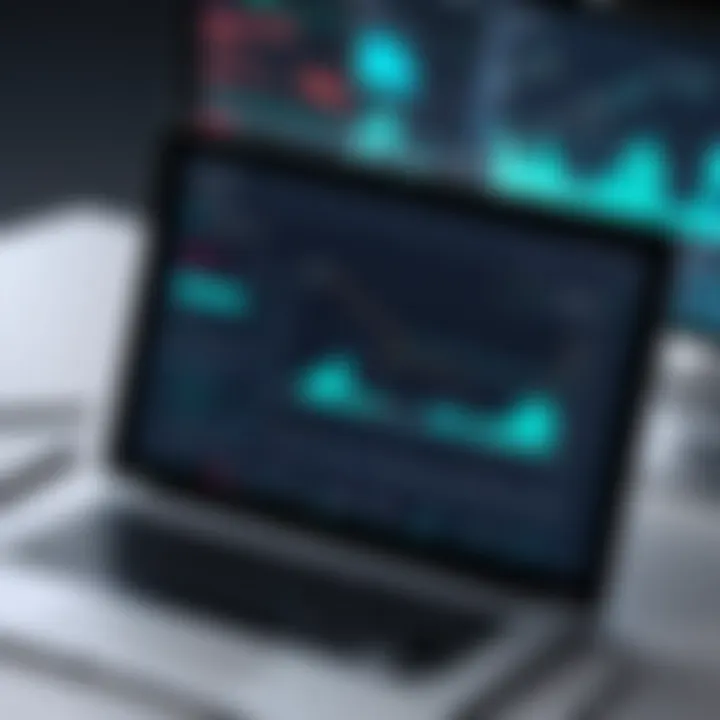
"By leveraging LLMs, financial institutions can achieve unprecedented levels of efficiency and customer satisfaction."
As the capabilities of LLMs continue to advance, their role in banking becomes increasingly indispensable, illustrating the immense potential AI holds within this critical industry.
The Importance of AI in Banking
The integration of artificial intelligence (AI) into the banking sector has become a cornerstone for modern financial services. AI technologies, particularly large language models (LLMs), play an essential role in transforming operations, enhancing customer engagement, and mitigating risks. As banks strive to compete in an increasingly digital landscape, understanding the significance of AI is crucial.
Current State of AI in Banking
Presently, the banking industry is witness to a rapid adoption of AI. Institutions are utilizing AI for various functions ranging from customer service to regulatory compliance. Many banks deploy chatbots for customer inquiries, which enables them to respond to client needs instantly, at any hour. In addition, AI is employed in data analysis, driving insights from large volumes of transactions and customer behaviors.
In recent years, AI algorithms have been trained to detect fraudulent activities by learning from historical data. This practice not only improves security but also enhances trust among users. Despite the advancements, it is vital to recognize that the current implementations of AI vary across institutions. Some banks remain hesitant due to concerns about reliability and the potential for failure in critical areas.
Benefits of AI Integration
The integration of AI in banking presents numerous advantages that cannot be overlooked. Firstly, efficiency is significantly enhanced. AI systems can perform repetitive tasks more quickly than human employees. This allows banks to allocate their resources more effectively, focusing human efforts on strategic decision-making.
Secondly, improved customer experience is evident. Personalization features driven by AI can analyze user data to recommend products tailored to individual needs. Such insights are made possible through continuous learning from interactions and preferences.
Another notable benefit is risk management. AI tools help in predicting market trends and assessing customer creditworthiness, which is invaluable for minimizing financial risks. In addition, regulatory bodies are beginning to recognize the efficiency of AI in ensuring compliance with laws, allowing banks to focus on core business activities instead of spending resources on cumbersome compliance processes.
The benefits of AI integration are substantial, positioning banks to thrive in a competitive environment where customer expectations are continually escalating, and technology is evolving.
"AI is not only a tech tool but a means to redefine the customer experience and operational strategy in banking."
Thus, the importance of AI in banking cannot be overstated. It supports the industry in adapting to new challenges, ensuring that financial services are both efficient and secure.
Applications of LLMs in Banking
Large language models (LLMs) have become pivotal in reshaping various operational aspects of the banking industry. By effectively processing and understanding human language, these models offer a range of applications that enhance efficiency, improve customer experiences, and bolster compliance efforts. In the context of banking, the integration of LLMs not only streamlines daily operations but also addresses some of the pressing challenges faced by the industry.
Customer Support and Chatbots
One of the most immediate applications of LLMs in banking is in customer support through chatbots. Traditional customer support systems often struggle with handling large volumes of inquiries. LLMs can significantly improve this by providing instant and accurate responses to customer queries at any time. These chatbots can interpret language nuances, manage context-based conversations, and even escalate issues when necessary, leading to enhanced customer satisfaction.
"The efficiency of AI-driven chatbots in banking can lead to reduction in operational costs and improvement in response times."
Moreover, LLMs aid in personalizing communication, allowing banks to tailor their messages based on customer preferences. This not only fosters customer trust but also encourages deeper engagement with banking services.
Data Analysis and Reporting
Another significant application is in data analysis and reporting. Banks deal with enormous amounts of data daily. LLMs analyze text data from various sources like emails, reports, and even social media. They can extract trends, sentiments, and key insights, which are invaluable for decision-making processes. For instance, by assessing customer feedback, banks can identify areas of improvement in their services or products.
Furthermore, LLMs simplify the creation of reports by generating human-like text from structured data, aiding in the documentation of critical findings and recommendations. This not only saves time but also enhances clarity in communication among stakeholders.
Fraud Detection and Prevention
Fraud detection is critical for maintaining the integrity of banking operations. LLMs are utilized to enhance fraud detection systems by processing and analyzing vast amounts of transaction data in real-time. Their ability to detect unusual patterns or anomalies in spending behaviors provides bankers with timely alerts about potential fraudulent activities.
Moreover, these models learn continuously from new data, allowing them to adapt to evolving fraud techniques. This proactive approach is essential for minimizing losses and protecting customer assets. LLMs also assist in automating responses to suspected fraud, reducing the lag time in intervention.
Regulatory Compliance
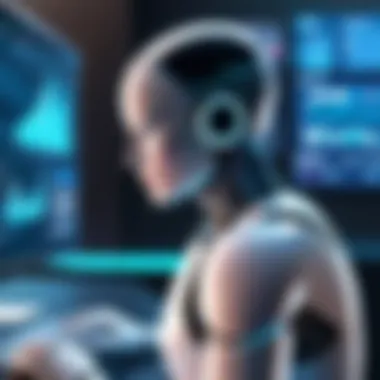
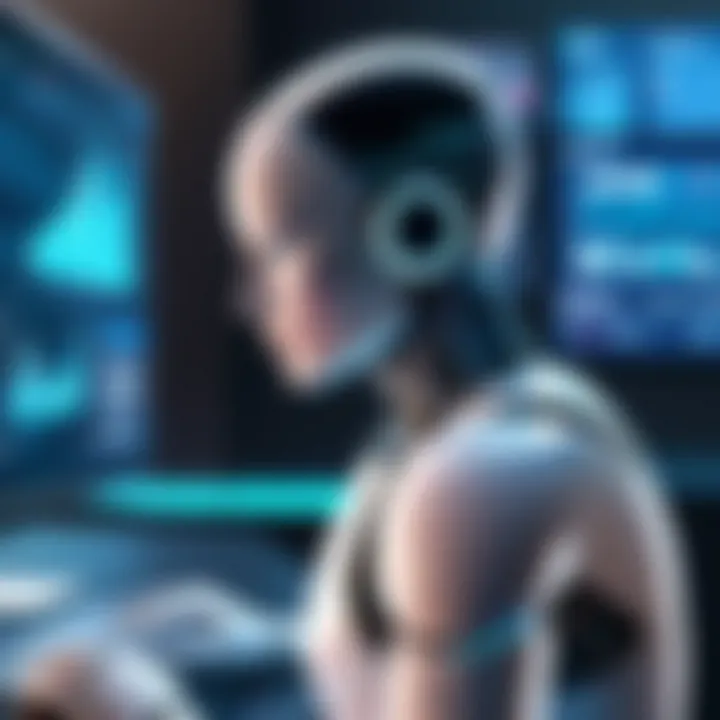
Compliance with regulations remains a cornerstone of banking operations. LLMs significantly contribute to this by assisting in monitoring and managing compliance risks. They can parse through numerous regulations, identifying essential requirements and ensuring that the bank's operations align with them.
Moreover, LLMs help in generating compliance reports, making audits less cumbersome. By keeping track of regulatory changes and summarizing their implications, these models enable banks to respond swiftly to evolving compliance landscapes. This ensures that institutions remain accountable and transparent in their operations, ultimately fostering trust among stakeholders.
Advantages of LLMs in Banking
The integration of large language models (LLMs) in banking presents a pivotal shift in how financial institutions operate and engage with their customers. As banks increasingly lean on Artificial Intelligence (AI), acknowledging the advantages of LLMs becomes crucial. Their potential lies not only in enhancing operational efficiency but also in offering superior customer experiences. Moreover, these models foster improved decision-making and strategic planning, enabling banks to stay competitive in a rapidly evolving financial landscape. Below are some of the key benefits LLMs bring to the banking sector.
Enhanced Customer Experience
Large language models enhance the customer experience in banking by personalizing interactions. They analyze vast amounts of customer data, identifying preferences and behavior patterns. By doing so, they generate recommendations tailored to individual clients. For instance, when customers ask questions about their accounts or seek advice on financial products, LLMs provide timely, accurate, and relevant responses. This ability facilitates seamless engagement, often through chatbots that operate around the clock. The result is higher satisfaction rates and stronger customer relationships.
Moreover, LLMs can assist in emotional analysis by interpreting the tone of a customer's communication. This skill allows banking representatives to adapt their responses appropriately, fostering a human-like interaction despite being an AI system. Such advancements lead to enhanced customer loyalty, a critical element for banks looking to maintain their market position.
Increased Efficiency and Productivity
The efficiency gains from implementing LLMs are significant. By automating routine tasks such as data entry, transaction processing, and report generation, banks can redirect human resources towards higher-value activities. Employees can focus on analytical tasks that require critical thinking rather than mundane operations. This shift not only increases productivity but also reduces the likelihood of human error.
In addition, LLMs streamline internal communications. They assist in document management by generating summaries or extracting key information from large datasets. Consequently, employees spend less time searching for information and more time addressing customer needs or developing new services. Overall, LLMs help organizations operate more efficiently, which is vital in a highly competitive market.
Improved Decision-Making
Large language models also empower banking institutions to make better decisions. By analyzing past data and predicting future trends, LLMs provide valuable insights that inform strategic planning. For example, LLMs can process economic indicators and market data to identify potential financial risks and opportunities, enabling banks to take a proactive approach to risk management.
Additionally, LLMs can enhance compliance efforts by interpreting regulations and assessing risks associated with transactions. They ensure that the institution adheres to legal guidelines without sacrificing operational efficiency. This capability is particularly important in an era where regulatory landscapes constantly evolve.
"The true potential of LLMs in banking lies in their capacity to transform traditional practices into a more efficient and client-centric approach."
In summary, the advantages of LLMs in banking are multifaceted. They enrich customer interactions, drive productivity, and support informed decision-making. As banks continue to harness AI and advanced technologies, the role of LLMs will likely expand, further transforming the industry.
Challenges of Implementing LLMs
The incorporation of large language models (LLMs) in banking offers various advantages, but it is essential to understand the challenges that come with their implementation. This section explores the key obstacles that institutions may face when integrating these advanced technologies. Addressing these challenges not only helps mitigate risks but also ensures a smoother transition and more efficient use of LLMs in banking operations.
Data Privacy Concerns
Data privacy is a critical issue when employing LLMs in banking. With vast amounts of sensitive financial data involved, institutions must consider how they handle and store this information. The risk of data breaches is heightened when LLMs process numerous customer interactions or personal data. This concern is compounded by regulations such as the General Data Protection Regulation (GDPR) in Europe that mandate strict guidelines on personal data use. Banks need to implement robust data governance frameworks to safeguard customer data while ensuring compliance with legal standards. Failure to do so could lead to significant reputational damage and financial penalties.
Algorithmic Bias and Fairness
Algorithmic bias is another substantial challenge. LLMs learn from vast datasets, which may inadvertently carry biases based on historical data. If these biases are not addressed, LLMs can contribute to unfair treatment of certain customer demographics. For instance, if a model is trained on biased data, it may deny loans to qualified applicants based on skewed predictive algorithms. To counteract this, financial institutions must actively work on ensuring fairness in AI models. This may involve regular auditing of algorithms, diverse training datasets, and incorporating fairness metrics in the evaluation stages of LLM deployment.
Technical Limitations
Despite their capabilities, LLMs face technical limitations. They require significant computational power and resources, which can be a barrier for smaller institutions. Additionally, LLMs may struggle with specific requests that need industry-specific knowledge or intuition. Their effectiveness often declines in areas where they encounter ambiguity or nuanced queries. Furthermore, continuous updates and maintenance are necessary to keep models relevant, which can strain IT resources. Combating these limitations requires adopting a hybrid approach, where LLMs work alongside human expertise to enhance overall decision-making processes in banking.
"The successful integration of large language models in banking will depend on understanding and overcoming these challenges."
Overall, while the potential of LLMs in the banking sector is promising, the challenges of data privacy, algorithmic bias, and technical limitations must be carefully navigated. Actionable strategies and best practices are vital for addressing these concerns effectively. As banks move towards greater digital transformation, tackling these issues will ensure that LLMs can be incorporated successfully and ethically.
Ethical Considerations
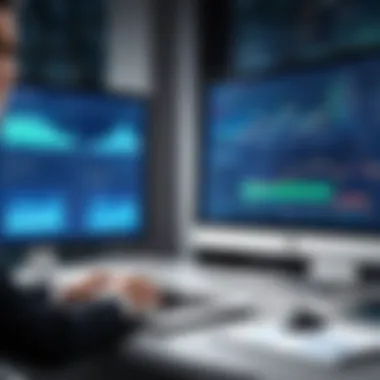
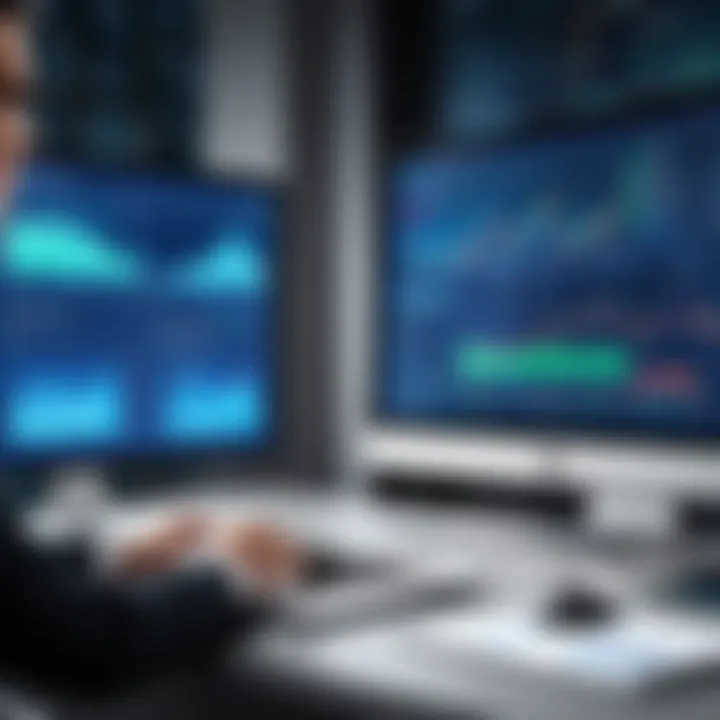
The integration of large language models (LLMs) in banking raises several important ethical inquiries that must be addressed. The financial industry operates under strict regulations and high expectations regarding trust and accountability. As such, ethical considerations become paramount in ensuring that the deployment of LLMs aligns with the values and standards of the banking sector. By exploring these considerations, we gain insight into the potential risks and challenges that accompany the implementation of such advanced technologies.
Transparency in AI Use
Transparency in AI use is a crucial aspect of maintaining trust with customers and regulatory bodies. Banks utilizing LLMs must ensure that their operations are clear and understandable. Customers deserve to know how their data is being processed and the decision-making processes behind AI-driven recommendations or actions. This clarity helps demystify AI systems, reducing anxiety and skepticism.
Effective transparency involves several key elements:
- Clear Communication: Financial institutions should provide accessible information about how LLMs function in customer interactions and data analysis.
- Open Algorithms: Where possible, banks can share the methodologies and algorithms used in LLMs, which allows for greater scrutiny and trust.
- User Controls: Empowering users with options to understand or influence AI interactions fosters a sense of control.
By promoting transparency, banks not only strengthen their customer relationships but also ensure adherence to legal compliance frameworks, such as GDPR.
Accountability and Oversight
The deployment of LLMs warrants robust accountability and oversight mechanisms. As machine learning models can produce outcomes that are not always predictable, it becomes essential to monitor their performance continuously. Financial institutions must establish clear lines of accountability to address any negative outcomes or misuse of the technology.
Key factors in promoting accountability include:
- Regular Audits: Conduct frequent audits of LLM outputs to assess their impact and performance, adjusting as necessary to improve outcomes.
- Human Oversight: LLMs should function under human decision-making frameworks, particularly in sensitive areas, to ensure balanced and fair outcomes.
- Ethical Guidelines: Establishing ethical guidelines for the development and implementation of LLMs can help in defining acceptable use cases and limitations.
Implementing these accountability measures safeguards against potential abuses while also reinforcing the integrity of the banking institution's AI initiatives.
Ethical practices promote trust and ensure the sustainability of AI technologies in finance.
By focusing on these ethical considerations, banks can navigate the complexities of AI while enhancing customer trust and regulatory compliance. Such diligence not only reflects a commitment to ethical standards but also positions banking institutions favorably in an increasingly AI-driven market.
Future Implications for Employment
The integration of large language models (LLMs) within the banking sector carries significant implications for employment. As technology continues to advance, understanding these implications is essential for both the workforce and employers. The conversation around LLMs often focuses on efficiency and automation, but it is critical to also consider the broader impact on job markets and skill requirements.
Job Displacement vs. Job Creation
One of the most discussed aspects of LLMs is job displacement. The automation of various tasks can lead to the reduction of certain roles, particularly those involved in routine and repetitive activities. For example, functions like data entry, basic customer support, and document processing may decline as LLMs take over these tasks. However, it is essential to analyze this from a broader perspective. While some jobs may be lost, others may very well be created. LLMs can enhance existing positions by taking over mundane tasks, allowing employees to focus on more complex and strategic challenges.
- New Roles Emerging: Roles that require a combination of technical understanding and soft skills are likely to rise. Positions such as AI trainers or machine learning specialists will become critical as banks attempt to optimize and maintain LLM implementations.
- Adaptation in Existing Roles: Many current roles in customer service, compliance, and risk management will evolve. Employees will need to work alongside LLMs, leveraging their capabilities to provide better service and informed decisions.
This dual narrative of job displacement and creation underscores the necessity for proactive transition strategies by banks and training institutions.
Skills Required in an AI-Driven Environment
As the bankng industry shifts toward more AI-centric operations, the skill sets required for employees will inevitably change. The intersection of advanced technology and human expertise will define the future workforce. Understanding the skills needed is vital for workers to remain relevant in this new landscape.
- Technical Skills: Proficient knowledge in AI and machine learning concepts is becoming critical. Familiarity with programming languages such as Python or R can be advantageous for tasks related to data analysis and model development.
- Analytical Skills: Employees will need to harness analytical skills to interpret data-driven insights generated by LLMs. The ability to question and critically assess AI outputs will be an asset in making informed decisions.
- Soft Skills: Empathy and interpersonal skills will not lose their value. As LLMs take over transactional roles, the human element in customer interaction becomes more important in high-stakes scenarios like financial advice and conflict resolution.
- Continuous Learning: The landscape is constantly evolving. Employees must embrace lifelong learning to keep abreast of emerging trends and technologies. Organizations should support training initiatives to ensure their workforce can adapt effectively.
The future landscape of work in banking will require adaptability, technical acuity, and a keen understanding of the human-computer relationship. The challenge and opportunity lie in how workers and institutions respond to these changes.
Culmination
The integration of large language models in the banking sector signifies a transformative shift, reshaping operations and client interactions. This conclusion synthesizes critical elements discussed throughout the article, emphasizing the importance of LLMs in modern banking.
Summary of Key Points
- Applications of LLMs: LLMs improve customer support through chatbots, enhance data analysis for better decision-making, aid in fraud detection, and ensure regulatory compliance.
- Advantages: With LLMs, banks can provide personalized services, optimize efficiency, and make informed decisions based on comprehensive data analysis.
- Challenges: Despite their potential, challenges such as data privacy concerns, algorithmic bias, and technical limitations must be addressed to ensure ethical implementation.
- Ethical Considerations: Ensuring transparency in AI usage and establishing accountability is vital to maintaining trust and compliance in banking practices.
- Future of Employment: The role of LLMs could lead to both job displacement and the creation of new job opportunities, necessitating a shift in required skills within the industry.
Looking Ahead: The Future of Banking with LLMs
As we move forward, the future of banking will likely see more sophisticated applications of LLMs. The potential for enhanced analytics capabilities will continue to grow, allowing banks to better understand customer needs and market trends. Furthermore, the proliferation of AI-driven tools could augment financial advisory services, tailoring advice to individual client situations.
Lessons learned from implementing LLMs will inform best practices and mitigate risks associated with AI deployment. Education and training for banking professionals will become essential as new tools emerge and traditional roles evolve. Thus, the continued collaboration between humans and AI forms a new frontier in the banking landscape.