Exploring Multi-Plot Mapping in Data Visualization
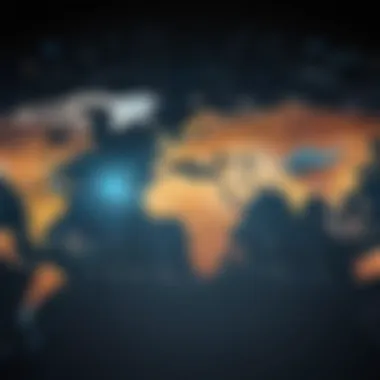
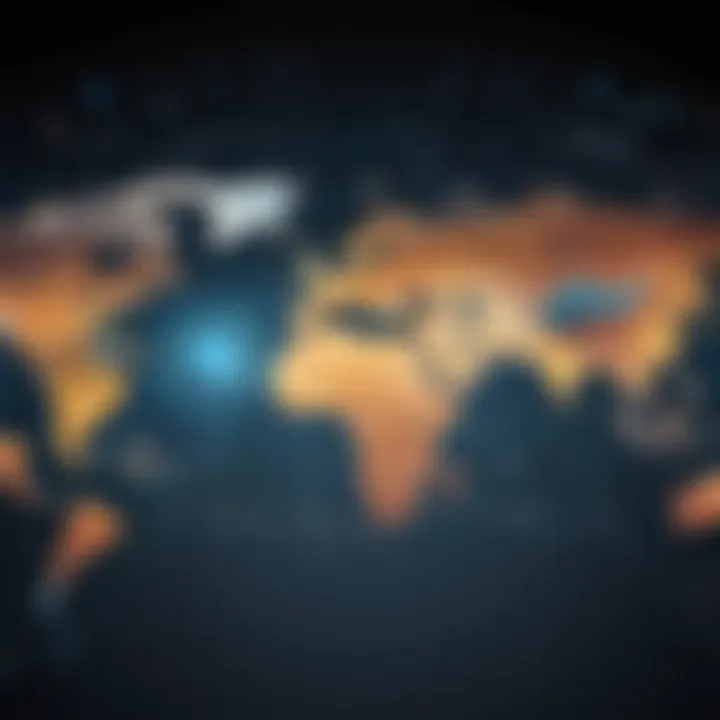
Intro
In the evolving landscape of data visualization, the way we interpret and present complex data sets has shifted drastically. One of the most groundbreaking techniques emerging from this revolution is multi-plot mapping. This approach allows data analysts to unveil layers of information simultaneously through various visual formats. From scatter plots to heat maps, the essence of multi-plot mapping lies in its ability to encapsulate multifaceted data in a digestible form, making it easier for individuals and organizations to draw meaningful insights.
Multi-plot mapping has become essential for tech enthusiasts, entrepreneurs, and data analysts alike. The necessity to sift through mountains of data and extract actionable intelligence has led to a demand for methodologies that do not merely present visuals but also foster comprehensive understanding. As we explore this innovative technique, we will delve into its pivotal role in enhancing analytical capabilities and facilitating informed decision-making.
In this article, we will provide an in-depth examination of how multi-plot maps serve varied applications across different industries, reflect on effective practices for ensuring clarity in visual communication, and paint a comprehensive picture of this fascinating subject.
Understanding Multi-Plot Mapping
Multi-plot mapping is a pivotal technique in data visualization, allowing observers to dissect and interpret complex datasets swiftly. This approach is akin to having multiple lenses through which to see the same landscape—each angle revealing unique details and insights. Embracing multi-plot mapping elevates one's analytical prowess, significantly enhancing the capacity to unveil relationships and trends buried deep within data.
One of the core elements of understanding multi-plot mapping is acknowledging its inherent value in data analysis. By presenting numerous plots simultaneously, it fosters a richer context for viewers, who may otherwise drown in a sea of numerical information. The benefits are manifold:
- Enhanced Clarity: Instead of sifting through extensive tables or individual graphs, a multi-plot format clusters related information, facilitating easier comparisons and insights.
- Contextualization: It places different data variables side by side, enabling viewers to see correlations that would remain obscure in single-plot representations.
- Space Efficiency: With the ability to cover more ground in a compact visual area, this mapping technique is a savior for presentations and reports that demand clarity without scale.
However, understanding multi-plot mapping is not without its caveats. It's crucial to consider potential pitfalls, such as misinformation arising from clutter. Too many plots might overwhelm an audience, causing important details to fade into the background. Thus, the artistry of multi-plot mapping lies in balancing information density with visual clarity.
The Fundamentals of Multi-Plot Mapping
Before venturing into how multi-plot mapping operates, it’s key to grasp its fundamental components. At its essence, multi-plot mapping comprises several visual representations grouped together based on a common theme or dataset.
Common types include:
- Scatter plots exhibiting relationships between two variables across multiple datasets,
- Histograms displaying distributions to compare populations,
- Line graphs tracking changes over time in several categories.
Each type serves distinct purposes yet shares the common goal of elucidating complex data connections. Properly utilized, they help forge a cohesive narrative around data, transforming raw figures into compelling stories.
Historical Context and Evolution
The journey of data visualization has deep roots, dating back centuries. While early civilizations utilized primitive visuals to communicate information—think of cave paintings or early maps—it was only in the 18th century, with figures like William Playfair, that the modern concept of graphing began to take shape. Playfair's work in creating line and bar graphs set the stage for future developments.
As time marched on, the increasing complexity of data driven by advancements in technology necessitated a profound evolution in visualization methods. Multi-plot mapping emerged as a response to the growing demand for clarity amid an explosion of information.
As computing power accelerated, tools became available that allowed data analysts to implement multi-plot maps with ease. Software like Tableau and Microsoft Power BI transformed how users interact with data, enabling a more sophisticated deployment of visual techniques. This evolution highlights not only the adaptability of visualization methods but also their role in informed decision-making processes across diverse fields.
"Data visualization is not just about display; it’s about establishing a clear connection between the observer and the data."
In summary, understanding multi-plot mapping is not just an academic pursuit; it’s a practical skill essential for navigating today’s data-rich landscape. With tools and techniques evolving, staying ahead in multi-plot mapping can be a game changer for tech professionals and entrepreneurs alike.
The Role of Data Visualization in Interpretation
Data visualization serves as more than just a means to present information; it acts as a bridge between raw data and meaningful insight. Through visualization, the complexities of large and intricate datasets can be distilled into digestible formats, allowing us to interpret signs and trends that might otherwise go unnoticed. In an age where data-driven decision-making reigns supreme, the significance of multi-plot mapping becomes particularly critical, as it offers an organized aesthetic for analyzing relationships through various lenses.
Why Visualization Matters
Understanding why visualization holds paramount importance in today's data ecosystem involves delving into its multifold benefits:
- Simplification of Complex Data: Visualizations break down intricate datasets into simpler, more understandable forms. For instance, a multi-plot map can clarify the dynamics between different variables, such as sales versus advertising spend across multiple platforms.
- Enhanced Insight Discovery: An eye-catching plot can reveal patterns, outliers, or trends that raw data may obscure. Charts, heat maps, and clustering can expose correlations that prompt further insights, aiding analysts in deriving conclusions quickly.
- Facilitating Better Communication: Visual aids make it easier for stakeholders to grasp the message behind the data. The proverbial saying, “a picture is worth a thousand words,” rings particularly accurate in data visualization. A well-designed map can tell a story about the data that can be grasped in seconds, fostering discussions and decisions.
- Aiding Memory Retention: Information presented visually is retained longer than text-based data alone. This aspect is crucial in presentation scenarios where key takeaways must linger long after the information session concludes.
Key Principles of Effective Data Visualization
Designing effective data visualizations, especially in the context of multi-plot mapping, is guided by several principles that ensure clarity and utility:
- Clarity Over Complexity: Always prioritize clarity when presenting data. Aim for straightforward visuals that guide the viewer's attention to the most important details without overwhelming them.
- Consistency in Design: A coherent design across multiple plots helps viewers to quickly adapt and understand the various facets presented. It ensures that colors, scales, and formatting are consistent, leading to less confusion.
- Interactive Features: Incorporating interactive elements into multi-plot maps can dramatically enhance engagement. Features like tooltips or drill-down menus allow users to explore dimensions of the data dynamically, making the visualization more compelling.
- Contextual Relevance: Always include context for the data being presented. This can involve annotations or brief explanations to prevent misinterpretation of the visualized data. Understanding the
Features of Multi-Plot Mapping
Understanding the features of multi-plot mapping is essential for comprehending its significance in data visualization. These features not only enhance analytical capabilities but also allow for a richer narrative when dissecting complex datasets. Multi-plot mapping enables users to represent various dimensions of data simultaneously, providing a panorama that individual plots simply cannot deliver.
Types of Multi-Plot Maps
There are several types of multi-plot maps that serve different purposes and can be tailored to specific analytical needs. Here’s a look at some common types:
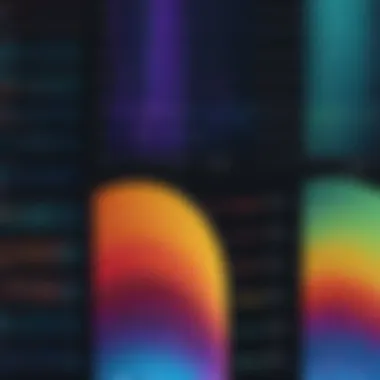
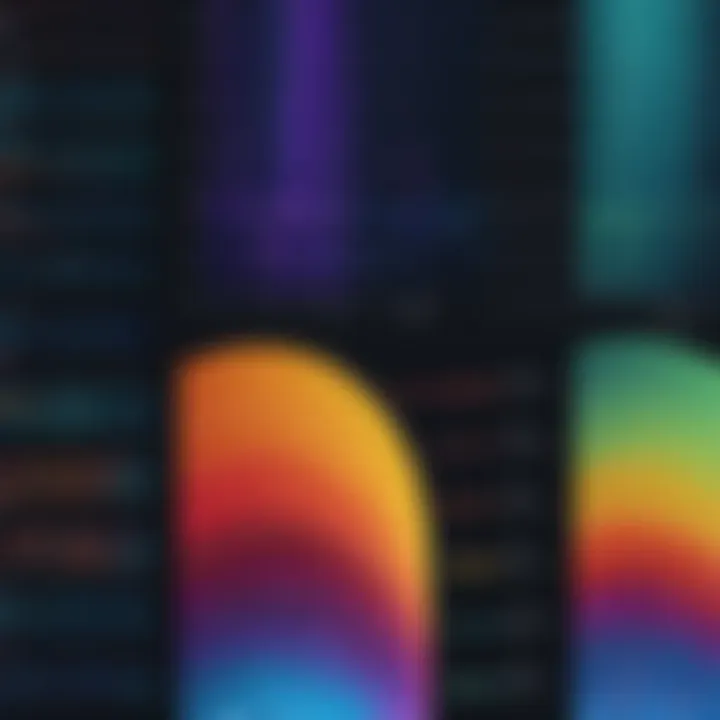
- Scatterplot Matrix: This series of scatterplots displays the relationships between multiple variables in a compact form. It serves as a valuable tool for assessing correlations and spotting patterns. Placing many scatterplots side-by-side can quickly highlight where data points cluster, making it apparent where relationships are strong or weak.
- Faceted Plots: These are essentially individual plots divided by one categorical variable. They are incredibly useful for discerning how a particular variable impacts others across different groups. For example, if you were examining sales data, facets can show sales patterns among different regions, allowing decision-makers to adapt strategies accordingly.
- Heatmaps: While not strictly a multi-plot format, heatmaps can display multiple variables where colors represent density or intensity. They are excellent for revealing trends in large datasets, such as website traffic across various hours of the day or year.
Choosing the right multi-plot type hinges on the specific analytical question at hand.
Capabilities and Flexibility
The capabilities and flexibility of multi-plot maps make them indispensable in modern data analysis. They offer several advantages:
- Overlapping Data Representation: Multi-plot mapping shines where overlapping data representation is vital. For instance, in epidemiological studies where multiple variables such as age, gender, and geographic location intersect, layering these variables in a multi-plot format can reveal intricate interactions that might go unnoticed in single-variable mappings.
- Increased Interpretability: When data is presented in multiple plots, it becomes easier to draw conclusions from different perspectives. This multi-faceted approach allows for a more comprehensive understanding of the data landscape. A single visualization might obscure critical insights, but with multi-plot mapping, analysts can piece together narratives from various angles.
- Customization and Interaction: Many software tools today allow customization of these maps to fit unique reporting needs. Users can choose colors, shapes, and layouts, enhancing readability and appeal. Interactive capabilities make it even more useful: stakeholders can explore data on their own terms, drilling down into specific areas of interest within the overall dataset.
Overall, the features of multi-plot mapping significantly enhance the ability to interpret and visualize complex datasets, making them a vital tool for tech enthusiasts and entrepreneurs aiming to harness data insightfully.
"In today's data-saturated environment, the ability to visualize complex relationships is not just a luxury; it’s a necessity for informed decision-making."
Utilizing these maps in various domains, be it healthcare, finance, or social sciences, underscores their broad applicability and relevance in contemporary analysis.
Methods for Creating Multi-Plot Maps
Creating multi-plot maps requires a thoughtful approach that takes into account various elements such as data structure, visualization objectives, and user interaction. This section lays the groundwork for producing effective multi-plot maps, emphasizing the significance of choosing the right tools and methodologies.
Software Tools and Platforms
To launch into the world of multi-plot mapping, familiarity with various software tools is essential. These tools serve as the backbone for your visualizations, allowing you to manipulate datasets and translate them into insightful graphics. The selection of a tool can dictate the ease of the workflow and the effectiveness of the resulting map.
Popular Tools Include:
- Tableau: It shines in creating interactive dashboards. Users can drag and drop elements to create complex visualizations, which is great for those who prefer a graphical interface.
- R with ggplot2: For those more inclined towards programming, ggplot2 is a formidable choice. It offers high customization for detailed plots, making it an excellent fit for researchers needing precise visual outputs.
- Python’s Matplotlib: A versatile tool that works well for automated plotting, particularly useful for data analysis processes that require reproducibility.
- Excel: Surprisingly effective for simpler multi-plot maps, especially in a corporate setting. Its accessibility enables quick mapping, even for those less familiar with data visualization.
These tools each come with unique strengths, so choosing one may depend on the specific needs of the project, such as the desired complexity of the mappings or the interaction level required.
Workflow for Implementing Multi-Plot Maps
Now that the tools are set, understanding the workflow for implementing multi-plot maps is crucial. It involves several steps that guide you from raw data through to the polished visual.
- Data Preparation: Ensure your data is clean and structured appropriately. It's akin to preparing the ingredients before you cook. Flawed data leads to misleading maps.
- Choosing Appropriate Visualizations: Not all data fits all visual modes. Depending on the nature of the data—be it categorical, continuous, or time-series—you need to select the right plotting techniques.
- Integration and Design: Here, you'll bring together different plots into a cohesive map. This often means determining how elements relate and adjusting visual parameters. A cohesive design caters not just to aesthetics but also to clarity; users should glean insights easily.
- Testing and Feedback: Once your map is created, it's wise to test it. Gather feedback from potential users. Their insights can be invaluable. Iterate based on their responses to refine clarity and interaction.
- Deployment: Finally, your multi-plot map is ready for sharing. Whether it’s internal presentations or public platforms like Reddit or Facebook, ensure it is accessible and serves its intended purpose.
"Design is not just what it looks like and feels like. Design is how it works." — Steve Jobs
Supporting these steps with a robust workflow not only enhances efficiency but also increases the likelihood of creating an impactful visual.
With proper methods in place, multi-plot mapping can transform complex datasets into actionable insights, paving the way for data-driven decision making in various industries.
Applications Across Various Industries
In the realm of data visualization, multi-plot mapping emerges as a powerful tool that transcends industry boundaries. Understanding its application across various sectors can paint a clearer picture of its significance. By utilizing this technique, businesses and organizations can discern patterns and relationships in complex datasets that, at first glance, might seem tangled like a ball of yarn.
Data visualization, which should never be relegated to mere aesthetics, serves as the backbone of analytical insights. Multi-plot maps allow stakeholders to visualize multifaceted dimensions of their data, which is especially critical in fields where precision and clarity can make or break decisions. Below, we delve into specific applications of multi-plot mapping within key industries.
Healthcare and Epidemiology
In healthcare, the stakes are high, making accurate data representation crucial. Multi-plot mapping plays a vital role in epidemiological studies, where understanding disease outbreaks can save lives. By layering various data points, such as infection rates, demographic information, and environmental factors, health analysts can uncover correlations that may not be immediately obvious.
For instance, during the COVID-19 pandemic, researchers employed multi-plot maps to visualize the spread of the virus across regions, breaking down cases by age group and pre-existing conditions. This granular approach helped public health officials allocate resources more effectively and target interventions to vulnerable populations.
Moreover, multi-plot maps can facilitate longitudinal studies, guiding healthcare providers in tracking the effectiveness of treatments or interventions over time. Here, complex datasets become easier to digest, allowing for informed decision-making based on a comprehensive view of patient outcomes.
Finance and Market Analysis
In the fast-paced world of finance, the ability to make quick yet informed decisions based on data visualization can yield substantial returns. Multi-plot mapping serves astute investors by presenting intricate relationships between financial indicators, such as stock prices, trading volumes, and market sentiment.
Consider a financial analyst using multi-plot maps to visualize the intricate web of correlations among sectors during an economic downturn. By analyzing how these sectors interrelate, they can anticipate potential market shifts and adjust their investment strategies accordingly.
Additionally, multi-plot mapping facilitates risk assessment. For example, investors can visualize potential risks across multiple assets, enabling them to better diversify their portfolio. The ability to overlay various financial metrics makes it possible to gain deeper insights into market behavior, turning data into actionable strategies.
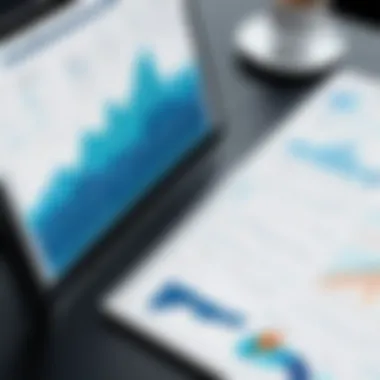
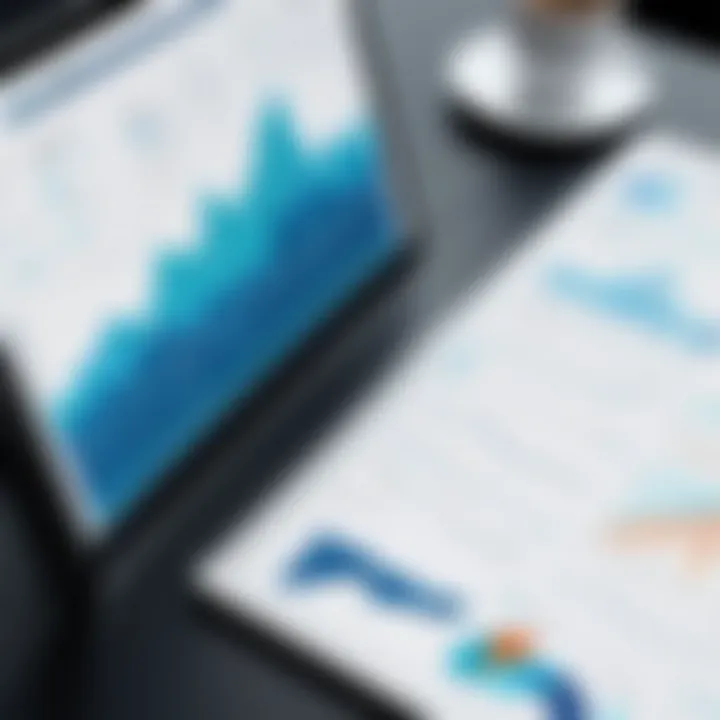
Social Sciences and Demography
In the social sciences, where understanding societal trends is paramount, multi-plot mapping provides researchers with a versatile framework to analyze and communicate data. By integrating various data sets—such as socioeconomic status, education levels, and geographic locations—scholars can craft a more nuanced picture of social dynamics.
For instance, using a multi-plot map, researchers can visualize the clustering of poverty in urban areas against education levels. Such insights can be pivotal in formulating policies aimed at community development and resource allocation.
Moreover, public policy makers often rely on these visualizations to understand demographic changes over time. Having a clear visual representation of data allows them to communicate findings to stakeholders, making a compelling case for initiatives that address inequalities or target specific populations.
In summary, applications of multi-plot mapping across these varied industries underscore its value. Whether in healthcare, finance, or social sciences, this technique not only enhances data comprehension but also drives informed decision-making—transforming raw data into actionable intelligence that impacts lives and livelihoods.
Challenges in Multi-Plot Mapping
Multi-plot mapping serves as a robust tool in data visualization, offering an intricate look into the relationships within vast data sets. However, with great power comes notable challenges that must be addressed to harness the full potential of this technique. Understanding these challenges not only sharpens the effectiveness of visualization efforts but also enhances analytical accuracy.
This section aims to shed light on specific elements of multi-plot mapping that, if not handled judiciously, can lead to confusion rather than clarity. By navigating these nuanced considerations, data analysts, tech enthusiasts, and entrepreneurs can create visualizations that are not just eye-catching but truly informative.
Data Overload and Clutter
One of the predominant issues in multi-plot mapping is the danger of data overload. When a map displays an excessive amount of information, readers can easily become overwhelmed. This clutter may obscure significant patterns or insights that the visualization is meant to reveal.
Multiple dimensions often result in busy graphics that, while rich in detail, can make it hard for users to draw actionable conclusions. Often, analysts might be tempted to throw every available data point onto one plot, thinking it adds depth. In reality, this approach can backfire, leading to visual chaos. An effective practice is to limit the number of data variables presented at one time.
For example, consider a mapping exercise that includes sales data across various regions, customer demographics, and product types. If all these elements are simultaneously plotted, it could resemble a spaghetti mess rather than a coherent data story. The goal should be clarity over quantity.
Tips for Avoiding Overload:
- Select Key Variables: Focus on the most relevant data points that contribute to the analysis.
- Utilize Interactive Elements: Implement features that allow users to filter or drill down into specific aspects of the data.
- Color and Size Coding: Use distinct colors and sizes to represent different categories rather than relying on mere density.
Maintaining Clarity and Usability
Even if a multi-plot map contains relevant data, its usability is paramount. A visually appealing plot that fails to convey its message is bupkis. Usability pertains not only to the ease of interpreting the data but also to the navigation through the visualization. An effective multi-plot should guide users effortlessly through the narrative it tells.
Another pitfall often encountered is the inconsistency of design. This can range from varying scales and axes across plots or mixed symbols and color schemes. Maintaining a uniform scale and consistent labeling simplifies the cognitive load on users, allowing them to focus on the data rather than decoding the visualization itself.
"In the world of visual data, simplicity is often the first casualty. Yet it is simplicity that can unlock true understanding."
Best Practices for Enhancing Clarity:
- Standardize Formats: Ensure that all plots adhere to a common design guideline.
- Incorporate Legends and Annotations: Adding annotations or legends helps users contextualize the data.
- Test for Usability: Solicit feedback from potential users to identify confusing elements before finalizing the plots.
By addressing these challenges head-on, those venturing into the realm of multi-plot mapping can maximize clarity and utility. This lays a solid foundation for data-driven decision-making, enabling stakeholders to embrace insights readily and effectively.
Best Practices for Effective Multi-Plot Mapping
Effective multi-plot mapping is not just about pushing data visuals onto a screen, rather it encompasses a thoughtful approach to data presentation. It’s crucial in ensuring that the data communicates clearly and impactfully. The right practices not only enhance interpretability but also significantly contribute to informed decision-making across various sectors. Let’s delve into two fundamental aspects that shape best practices: design principles and iterative feedback.
Design Principles
Design principles form the bedrock of any compelling visualization, especially in multi-plot mapping where myriad elements can lead to chaos if not handled properly.
A few essential design principles include:
- Simplicity is Key: The visual representation should stick to the essentials. Overloading a plot with too many elements can confuse rather than clarify. Limiting color palettes and trying to adhere to a uniform structure can help keep the audience focused.
- Logical Layout: The arrangement of plots should follow a logical flow that aids the viewer in drawing connections. For instance, placing related plots side by side can make patterns more apparent.
- Consistent Symbols and Colors: Using similar symbols or color themes across plots helps viewers form associations between different data sets. It's about creating a visual language that the audience can decode easily.
- Accessibility Considerations: It’s important to be conscious of color blindness and other accessibility issues. Using color combinations that contrast well or adding patterns can make a significant difference in readability for diverse audiences.
"Good design is about making complex things simple and simple things beautiful."
These principles, when practiced well, enable the viewer to engage with the data more intuitively. When a narrative is apparent through visuals, the risk of misinterpretation decreases significantly.
Iterating Feedback and Refinement
The process of creating effective multi-plot maps isn't static; it thrives on an iterative process of feedback and refinement. Gathering initial subject feedback can lead to useful insights that transform a mediocre plot into a powerful communication tool.
Here are some strategies to incorporate feedback effectively:
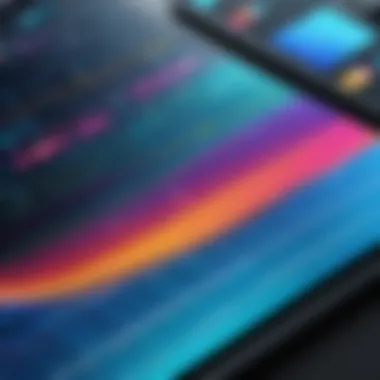
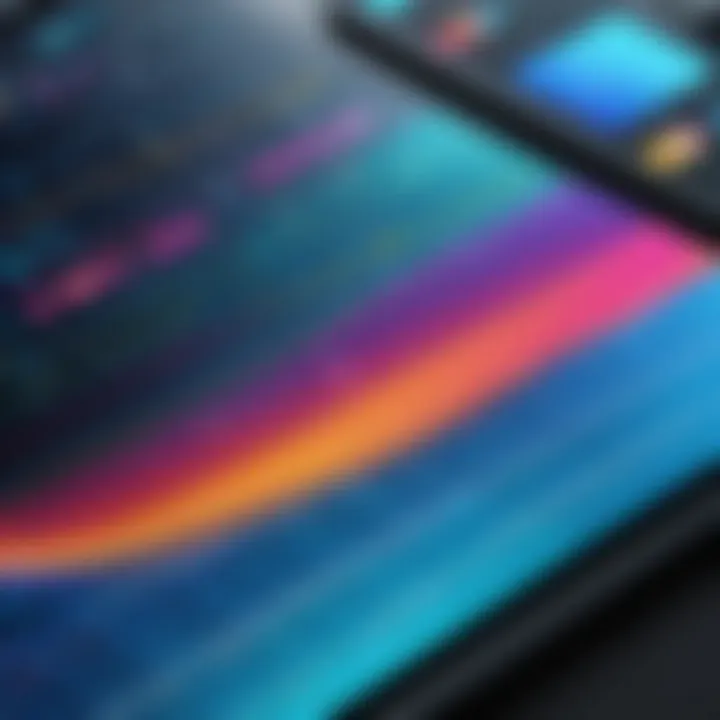
- Involve Stakeholders Early On: By involving key stakeholders in the early phases of the design, you ensure that their needs and preferences are taken into account. It’s about creating a visual narrative that resonates with the audience directly.
- Iterative Prototyping: Creating preliminary versions of your visualizations allows for exploration. Share these prototypes with your audience, and encourage candid critiques. Each round of input should aim to capture how well the visual communicates its intended message.
- Test for Clarity: Conduct informal tests with people unfamiliar with the data. Their reactions can reveal if the intended messages are communicated clearly. If they misinterpret data, it’s time to revise.
- Be Open to Changes: The willingness to reassess and modify your visualizations based on feedback is what turns a good presentation into a great one. Get comfortable with evolving your plots until they truly resonate with the viewer.
By prioritizing design principles and embedding a feedback loop into your workflow, crafting effective multi-plot maps can become a less daunting endeavor. Each cycle of creation, feedback, and refinement fosters clarity and intentionality in communicating complex data.
Future Trends in Data Visualization
Data visualization is not a stagnant field; it is constantly evolving and adapting to the rapid pace of technological advancement. Understanding future trends in this area is crucial for anyone involved in data analysis, whether they are tech enthusiasts, startup aficionados, entrepreneurs, or investors. Embracing these trends can lead to better decision-making, more effective communication of data insights, and ultimately, a competitive edge.
Technological Advancements
The rise of new tools and technologies shapes how we visualize data. Technologies like high-speed internet and powerful cloud computing capabilities are enabling the storage and processing of vast amounts of information that would have been unthinkable just a decade ago. Tools such as Tableau, Power BI, and D3.js are stirring the pot, making complex visualizations easier to create and share.
One key advancement is the shift towards real-time data visualization. Capturing data live allows organizations to react quickly to changes in the market or consumer behavior. For instance, if a retail company uses real-time sales data to adjust prices instantly, this can significantly boost revenue. Similarly, industries like healthcare are using real-time data to monitor patient health indicators, greatly improving patient response times.
Another aspect worth noting is the increasing sophistication of interactive visualizations. Users can now engage with data more intuitively, asking "what if" questions and immediately seeing the potential outcomes. This leads to deeper insights and a better understanding of complex data relationships.
"The future of data visualization lies not just in presenting numbers but in telling stories that resonate with the audience."
Integrating AI and Machine Learning
Artificial intelligence and machine learning aren't just buzzwords; they’re redefining the landscape of data visualization. These technologies can analyze vast datasets and uncover patterns that might go unnoticed through traditional means. When integrated into visualization tools, AI can help automate and improve the process of data discovery.
Machine learning algorithms can learn from the data, helping to create predictive visualizations that can forecast future trends. For example, a financial analyst might use machine learning to predict stock prices based on historical performance and current market conditions. Such insights transform stakeholders' strategic planning and investment decisions.
Additionally, AI-powered tools can enhance personal experiences by adapting visualizations to an individual’s preferences or previous interactions. This adds a layer of customization that can make the data more relevant and accessible, thereby driving greater engagement.
Case Studies in Multi-Plot Mapping
The realm of multi-plot mapping is rich with real-life applications that showcase its strength in conveying complex data. Case studies serve as a practical means to understand how these mappings can fully realize the potential of visual data representation. They not only highlight successful implementations but also provide cautionary tales from failures, offering an invaluable learning experience for tech enthusiasts, entrepreneurs, and data analysts alike. In this section, we will explore two essential aspects: notable success stories and the lessons gleaned from unsuccessful attempts.
Success Stories in Data-Driven Decision Making
The impact of multi-plot mapping has been vividly illustrated through several case studies across industries. For example, let’s take a closer look at how a healthcare company utilized multi-plot mapping to address disease outbreaks. This organization implemented a cholera risk map using geographic data overlays, comparing environmental conditions with reported cases. The result? They could pinpoint high-risk areas swiftly and allocate medical resources effectively.
Another vivid success story comes from a major financial institution that adopted multi-plot mapping for market analysis. By layering various data points—such as stock performance, economic indicators, and sector-specific trends—analysts visualized correlations that were previously hidden in spreadsheets. This not only streamlined internal reporting but also empowered decision-makers to act proactively rather than reactively.
"Visual representation of data isn't just about beauty; it transforms the way decisions are made."
— Analyst at XYZ Financial Corp.
These success stories exemplify how multi-plot mapping transforms raw data into meaningful insights. By combining datasets and offering visual clarity, organizations can make quicker, more informed decisions that ultimately enhance operational efficiency and drive results.
Lessons Learned from Failed Implementations
While the successes are inspiring, the failures are equally informative. Let’s consider the case of a tech startup that aimed to employ multi-plot mapping for user engagement analysis. Unfortunately, they opted for overly complex visuals. The result was confusion rather than clarity, as users struggled to interpret the data. Instead of enhancing decision-making, the convoluted plots caused more harm than good, leading to misinformed strategies and resource misallocation.
Another example involved a governmental agency trying to utilize multi-plot mapping for urban planning without proper context. They created maps that were visually appealing but disparate from the real-world implications. As a result, local community preferences and needs were overlooked, leading to public pushback against proposed initiatives.
These lessons stress the importance of keeping user experience in mind when implementing multi-plot mapping strategies. Clarity and relevance to the audience should always be prioritized. The failures remind us that visualizations must be user-friendly, emphasizing the proper communication of data rather than just sophisticated graphics.
Through these case studies, both successful and failed, we can grasp the full range of potential that multi-plot mapping holds in data visualization, equipping us with valuable insights to drive future applications.
Epilogue and Future Directions
The convergence of data visualization techniques, particularly multi-plot mapping, marks a pivotal moment in how we understand and interact with complex data. By synthesizing the discussions from this article, we can appreciate that multi-plot mapping is more than just a visual tool; it is a crucial element in the analytic toolbox of today's tech-savvy entrepreneurs and data practitioners. The benefits it offers are manifold, instrumental in turning raw data into actionable insights.
Summary of Key Insights
Throughout this exploration, several core insights unfurl:
- Holistic Perspective: Multi-plot mapping provides a comprehensive view of data relationships, enabling users to see broader patterns that individual charts may miss. This approach allows an analyst to glean insights across multiple dimensions, enhancing analytical depth.
- Enhanced Decision-Making: With clearer visual representation, decision-makers can act swiftly and accurately. It’s demonstrated that visual tools play an integral role in frameworks for strategic planning across industries.
- Adapting to Change: As the data landscape evolves, so too must our methods of understanding it. The flexibility of multi-plot mapping caters to this dynamic nature, allowing for adaptiveness in visual presentation that is crucial in real-time analysis scenarios.
In essence, embracing multi-plot mapping can transform how stakeholders conceive their data environments, making it a necessary approach in modern analytics.
Encouraging Further Exploration
This discussion serves as a springboard into the vast realm of data visualization. As multi-plot mapping holds promise for the future of analytical strategies, it becomes pertinent for data enthusiasts and industry players to dive deeper. Here are a few suggested avenues for exploration:
- Experimentation with Tools: Engage with various software platforms that facilitate multi-plot mapping. Tools like Tableau and R's ggplot2 can offer valuable hands-on experience.
- Interdisciplinary Learning: Benefit from the cross-pollination of ideas among different fields. How techniques from ecology, market research, or social science might inform multi-plot mapping practices can yield innovative approaches.
- Community Engagement: Join forums or communities on platforms like Reddit or Facebook where data visualization practitioners gather. Sharing insights and challenges can enrich the learning experience, providing practical knowledge from a diverse range of professionals.
Multi-plot mapping stands at a crossroads of analytical prowess and storytelling, offering a unique lens through which data can be appreciated and leveraged. As practitioners continue to refine methods and approaches, the possibilities only grow, inviting ongoing exploration and innovation in data visualization.