Unveiling the Impact of Open Source Knowledge Graphs in the Digital Era
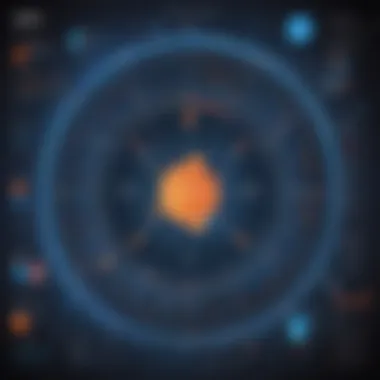
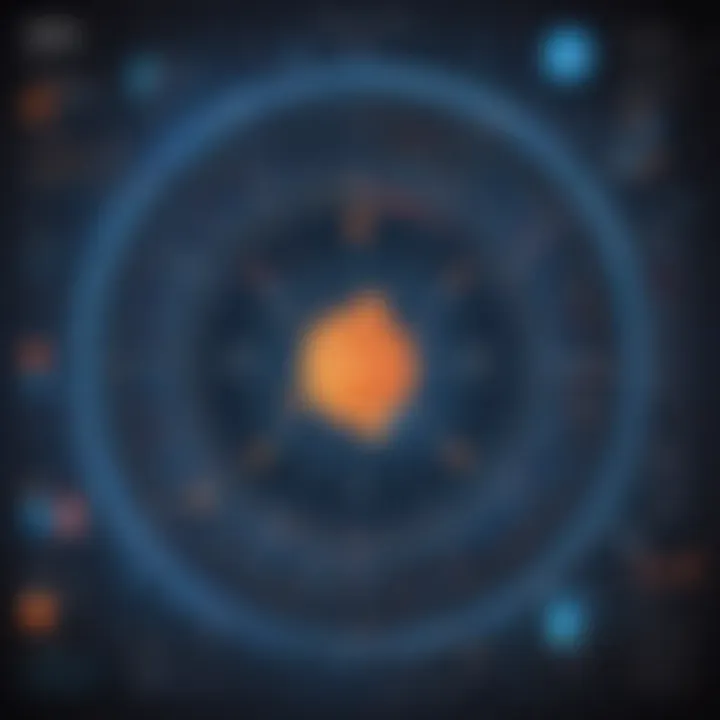
Latest Technological Innovations
In the realm of cutting-edge technology, the domain of open source knowledge graphs stands tall as a beacon of progress and innovation. By delving into the intricacies of knowledge graphs and examining the advantages inherent in an open source approach, one gets a glimpse into how these revolutionary technologies are reshaping the landscape of information organization and retrieval. The dynamic nature of open source platforms allows for collaborative contributions from diverse sources, fostering a robust ecosystem that enhances the quality and accessibility of knowledge. This evolution in information structuring is catalyzing a paradigm shift in how we interact with and leverage data, paving the way for more efficient and effective information retrieval mechanisms.
Startup Spotlight
Within the dynamic milieu of startups, a spotlight shines on companies venturing into the realm of open source knowledge graphs. These innovative startups are charting new territories by harnessing the power of collaborative knowledge sharing and leveraging open source technologies to drive their growth trajectory. Through their journey, these startups encounter challenges that test their resilience and creativity, propelling them to develop novel solutions and strategies. By overcoming obstacles and seizing opportunities, these startups carve a niche for themselves in the competitive landscape of high-tech entrepreneurship. Their success stories serve as beacons of inspiration for aspiring entrepreneurs, illustrating the transformative potential of open source knowledge graph technologies.
Tech Industry Trends
As the tech industry continues its rapid evolution, current trends indicate a robust inclination towards the adoption of open source knowledge graphs as integral components of information architecture. Companies across diverse sectors are embracing this disruptive technology, recognizing its potential to enhance operational efficiency and drive strategic decision-making. By analyzing different companies' approaches to integrating knowledge graphs into their workflows, we gain insights into the varied applications and implications of this technology. Moreover, the emergence of AI-driven solutions and the convergence of big data analytics with knowledge graphs signal a new era of data-driven innovation, poised to revolutionize how businesses leverage information for competitive advantage.
Investment Opportunities
Amidst the burgeoning landscape of high-tech and startup ventures, investment opportunities in the realm of open source knowledge graphs present a unique proposition for discerning investors. By evaluating key factors such as market potential, technological scalability, and competitive differentiation, investors can identify promising startups poised for exponential growth. The allure of backing innovative companies at the forefront of knowledge graph development offers investors a chance to partake in the digital transformation sweeping across industries. Through strategic investments and partnerships, stakeholders can contribute to and benefit from the rapid expansion of open source knowledge graph ecosystems, driving both financial returns and technological advancements.
Entrepreneurship Insights
For aspiring entrepreneurs navigating the fast-paced tech industry, insights gleaned from successful startup journeys in the realm of open source knowledge graphs provide valuable lessons in resilience and strategic foresight. By dissecting the strategies employed by entrepreneurs to overcome challenges and capitalize on opportunities, aspiring business leaders can glean actionable insights to steer their ventures towards success. Tips on team building, market positioning, and technology adoption offer a roadmap for entrepreneurs seeking to establish their presence in the digital economy. The dynamic landscape of open source knowledge graphs presents a fertile ground for entrepreneurial endeavors, catalyzing innovation and collaboration on a global scale.
Introduction
In delving into the realm of an open source knowledge graph, one must grasp the essential role it plays in the modern, digitally-driven landscape. The concept of a knowledge graph serves as a pivotal tool for organizing and retrieving vast amounts of information efficiently and effectively. By embracing an open source approach, the transparency and accessibility of knowledge graphs are heightened, giving rise to a new era of collaborative knowledge management. This article aims to shed light on the significance of open source knowledge graphs in revolutionizing how information is structured and accessed.
Defining Knowledge Graphs
Structured Data Representation
When we talk about structured data representation within a knowledge graph, we are referring to the meticulous organization and categorization of data points in a format that is easily navigable and comprehensible. This method of structuring information enhances the overall clarity and coherence of the knowledge graph, making it a popular choice for those seeking a systematic approach to data management. The unique feature of structured data representation lies in its ability to establish clear relationships between different pieces of data, enabling more advanced data analytics and interpretation within the context of the knowledge graph.
Graph-Based Information Model
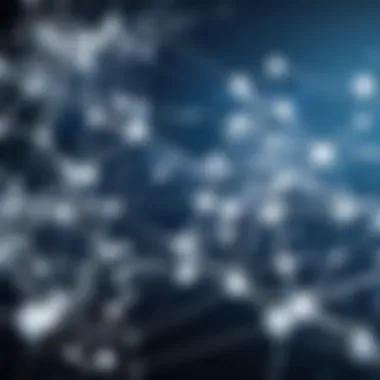
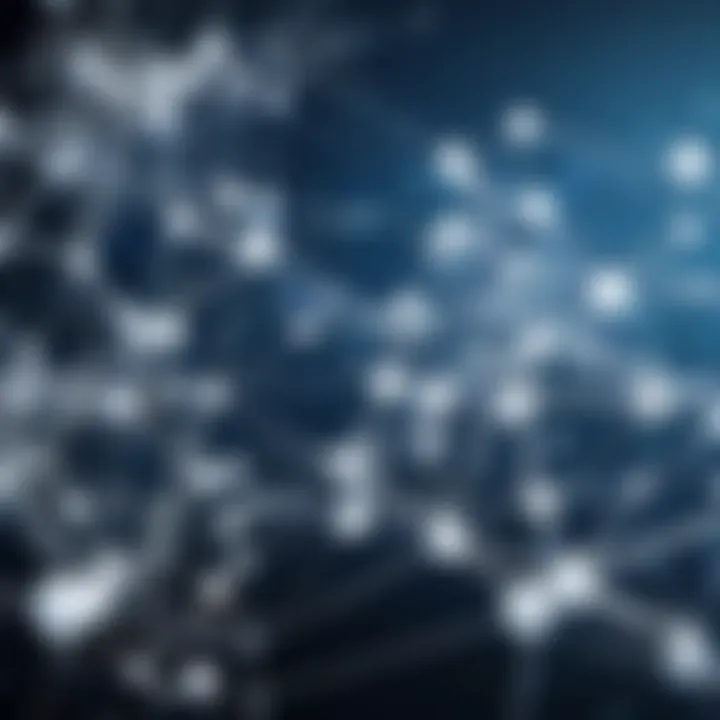
Within the realm of knowledge graphs, the graph-based information model stands out as a cornerstone for representing relationships between entities. This model highlights the interconnected nature of information, focusing on the links and dependencies that exist between various data points. By adopting a graph-based approach, knowledge graphs can capture complex relationships more effectively, offering a more nuanced understanding of the data landscape. While the advantages of a graph-based information model are substantial in terms of relationship mapping and data connectivity, challenges may arise regarding scalability and computational intensity.
The Rise of Open Source
Open Source Philosophy
At the core of the rise of open source knowledge graphs lies the fundamental principle of open source philosophy - the belief in the collective power of shared knowledge and collaborative development. This philosophy fosters a culture of transparency, inclusivity, and community-driven innovation, aligning perfectly with the democratization of information that open source technologies epitomize. The unique feature of open source philosophy lies in its ability to harness the collective ingenuity of a global community, facilitating rapid advancements in knowledge graph development while ensuring accountability and accessibility.
Collaborative Development Model
In tandem with open source philosophy, the collaborative development model embodies a paradigm shift in how knowledge graphs are created and maintained. By leveraging the expertise and diverse perspectives of contributors worldwide, this model promotes continuous learning, refinement, and innovation. The collaborative nature of knowledge graph development allows for accelerated growth and iteration, leading to more robust and adaptable solutions. However, challenges may arise concerning governance, quality control, and aligning divergent viewpoints in the development process.
Understanding Open Source Knowledge Graphs
Benefits of Open Source Knowledge Graphs
Enhanced Accessibility
Enhanced Accessibility is a critical aspect of open source knowledge graphs. It focuses on the ease of access and utilization of knowledge graph resources by a wide range of users. The key characteristic of Enhanced Accessibility lies in its inclusive nature, allowing both technical and non-technical individuals to interact with and benefit from knowledge graph data effortlessly. This accessibility promotes greater engagement with the technology and fosters a more collaborative and innovative environment. However, while Enhanced Accessibility enhances usability, it may also lead to potential data security risks if not appropriately managed within the open source framework.
Community-driven Innovation
Community-driven Innovation is a cornerstone of open source knowledge graphs, emphasizing the power of collective intelligence and collaboration in driving technological advancements. This concept underscores the active participation of diverse community members, including developers, researchers, and end-users, in innovating and evolving knowledge graph capabilities. The key characteristic of Community-driven Innovation lies in its ability to harness the collective expertise and creativity of a community towards common goals, accelerating the pace of innovation and problem-solving. While community-driven innovation fuels rapid development and idea generation, it may also introduce challenges related to coordinating diverse perspectives and achieving consensus within decentralized communities.
Cost-Effectiveness
Cost-Effectiveness is a key advantage of open source knowledge graphs, highlighting the economic benefits and affordability of adopting and maintaining such systems. The primary benefit of Cost-Effectiveness is its ability to significantly reduce the financial barriers associated with implementing knowledge graph solutions, making advanced technology more accessible to organizations of varying sizes and resource capacities. The unique feature of Cost-Effectiveness lies in the cost-saving potential achieved through shared development efforts, free access to open source tools, and reduced vendor lock-in. While cost-effectiveness promotes broader adoption and innovation, it may also pose challenges related to ongoing support, customization, and maintenance costs in the long run.
Challenges and Limitations
Quality Control Concerns
Quality Control Concerns represent a critical challenge within open source knowledge graphs, focusing on ensuring the accuracy, integrity, and reliability of information stored and retrieved within these systems. The key characteristic of Quality Control Concerns is the need for robust processes and mechanisms to verify and maintain data quality throughout the knowledge graph lifecycle. While quality control measures are essential for preserving the credibility and utility of knowledge graphs, they can also introduce complexities in terms of standardization, consistency, and scalability. The unique feature of Quality Control Concerns is their direct impact on decision-making, analysis, and knowledge discovery, underscoring the importance of continuous quality assurance practices within open source environments.
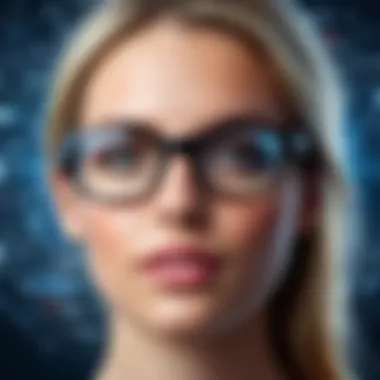
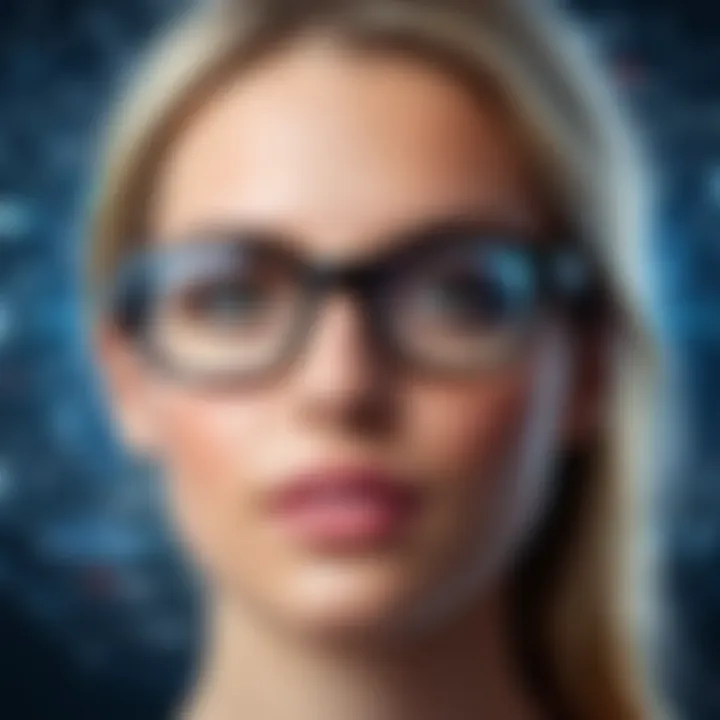
Integration Complexity
Integration Complexity emerges as a significant limitation in open source knowledge graphs, emphasizing the challenges and complexities associated with integrating diverse data sources, formats, and structures within a unified graph framework. The key characteristic of Integration Complexity lies in the intricacies of mapping, harmonizing, and connecting disparate data elements to create a coherent knowledge graph ecosystem. While integration complexity is essential for achieving comprehensive insights and holistic views of complex information landscapes, it may hinder the seamless integration of new data sources, inhibit interoperability, and increase maintenance efforts. The unique feature of Integration Complexity is its role in shaping the scalability, adaptability, and performance of knowledge graph implementations, requiring strategic planning and technical expertise to overcome integration hurdles.
Implementing Open Source Knowledge Graphs
Implementing Open Source Knowledge Graphs is a critical aspect of this discourse on the open-source knowledge graph landscape. It serves as the bridge between the conceptual framework and the practical application of knowledge graph technology. In this section, we will delve into the significance of leveraging open source methodologies for constructing and managing knowledge graphs. By adopting open-source practices, organizations can tap into a vast pool of collaborative intelligence and groundbreaking innovations. The emphasis will be on how implementing open source knowledge graphs can enhance data accessibility, streamline information retrieval, and foster a culture of transparent knowledge sharing.
Tools and Technologies
Graph Databases
Graph Databases play a pivotal role in the realm of knowledge graphs by providing a robust infrastructure for storing and querying interconnected data entities. One of the key attributes of Graph Databases is their ability to model relationships between disparate data points, offering a more intuitive representation of complex information structures. This feature is particularly advantageous for knowledge graph applications as it facilitates the traversal of interconnected data nodes, enabling faster and more efficient access to valuable insights. Despite their efficiency in handling connected data, Graph Databases may pose challenges in scalability and performance when dealing with extensive datasets. Organizations embracing knowledge graphs must weigh these pros and cons to make informed decisions regarding the adoption of Graph Databases.
Linked Data Standards
Linked Data Standards serve as a cornerstone for ensuring interoperability and semantic consistency across disparate data sources within a knowledge graph ecosystem. These standards govern the way in which data entities are interconnected and define protocols for sharing and querying linked data sets. By adhering to Linked Data Standards, organizations can foster data harmonization and enable seamless integration of heterogeneous data silos. The unique feature of Linked Data Standards lies in their capacity to foster data interconnectivity in a decentralized environment, promoting data transparency and accessibility. However, the adoption of Linked Data Standards may require significant efforts in data standardization and metadata enrichment, posing challenges in maintaining data quality and consistency.
Best Practices
Data Modeling Strategies
Effective Data Modeling Strategies are paramount in realizing the full potential of a knowledge graph implementation. These strategies encompass methodologies for defining data schemas, relationship types, and ontology structures within a knowledge graph framework. The key characteristic of Data Modeling Strategies lies in their ability to capture the semantic associations between data entities, thereby enriching the information retrieval capabilities of knowledge graphs. By leveraging sound data modeling principles, organizations can ensure data integrity, schema flexibility, and query efficiency within their knowledge graph environments. However, data modeling complexity and the need for domain-specific expertise may present hurdles for organizations seeking to implement optimal data modeling strategies.
Collaborative Development Guidelines
Collaborative Development Guidelines establish a framework for collective engagement and contribution within the open-source knowledge graph community. These guidelines outline best practices for collaborative data integration, ontology alignment, and version control mechanisms across distributed knowledge graph repositories. The key characteristic of Collaborative Development Guidelines is their role in fostering a culture of knowledge sharing, peer review, and continuous improvement within the knowledge graph ecosystem. By following these guidelines, organizations can harness the collective intelligence of a diverse community to enhance the quality and relevance of their knowledge graphs. Nevertheless, challenges related to governance, data stewardship, and community coordination may arise, requiring adept governance strategies to mitigate potential conflicts and ensure sustained collaboration.
Case Studies and Success Stories
Real-World Applications
Healthcare Industry
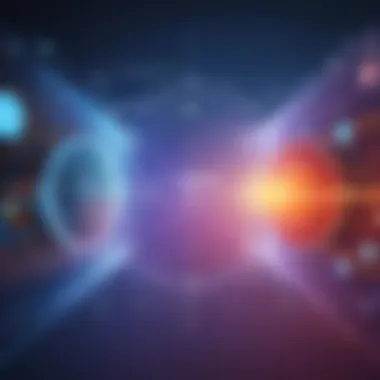
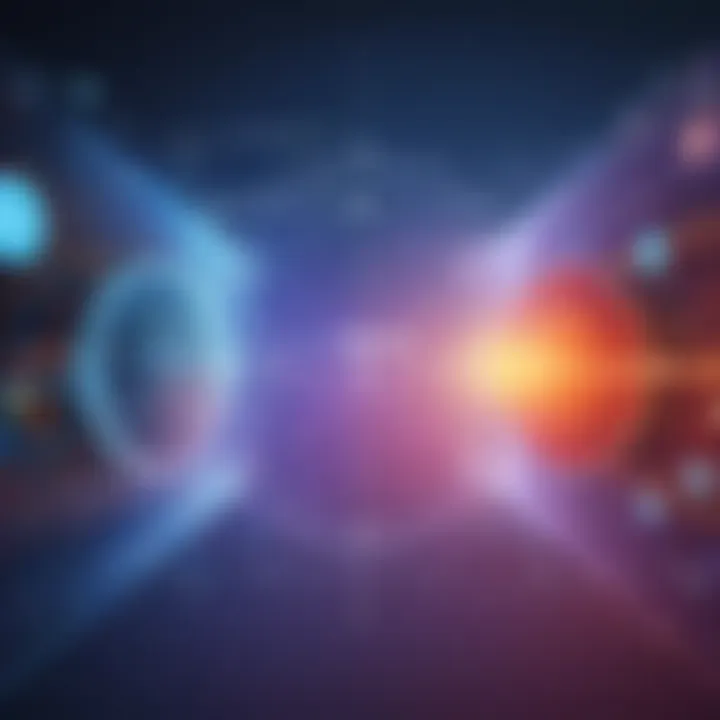
Discussing the application of open source knowledge graphs in the healthcare industry sheds light on specific use cases and their contributions to the broader technological landscape. The healthcare sector benefits significantly from knowledge graphs by facilitating data integration, improving patient outcomes, and enabling personalized treatment plans. The key characteristic of leveraging knowledge graphs in healthcare is the ability to connect disparate sources of medical information to generate holistic patient profiles. This approach enhances diagnostic accuracy, treatment efficacy, and research capabilities, making it a popular choice for healthcare organizations seeking to optimize their operations. Despite these advantages, challenges such as data privacy concerns and regulatory compliance issues need to be addressed to harness the full potential of knowledge graphs in the healthcare setting.
E-commerce Platforms
Exploring the integration of open source knowledge graphs in e-commerce platforms reveals unique opportunities for enhancing customer experiences and driving business growth. Knowledge graphs play a crucial role in improving product recommendations, optimizing search functionalities, and personalizing user interactions on e-commerce websites. The key characteristic of applying knowledge graphs in e-commerce lies in creating interconnected product databases that enhance browsing efficiency and purchasing decisions for consumers. This approach has become a popular choice for online retailers looking to stay ahead in a competitive market landscape. However, challenges such as data scalability and algorithm complexity should be carefully managed to ensure the seamless implementation of knowledge graphs in e-commerce settings.
Innovative Use Cases
Semantic Search
Examining the innovative use of semantic search within the context of open source knowledge graphs highlights its importance in information retrieval and knowledge discovery. Semantic search leverages natural language processing and AI algorithms to interpret user queries effectively, generating more relevant search results. The key characteristic of semantic search is its ability to understand context, intent, and relationships between data points, enabling more precise search outcomes. This feature makes semantic search a preferred choice for applications requiring complex search functionalities and content categorization. Despite its advantages in enhancing user experience and content discovery, challenges such as semantic ambiguity and query understanding complexity must be addressed to leverage the full potential of semantic search in knowledge graph implementations.
Personalized Recommendations
Exploring the utilization of personalized recommendations in conjunction with open source knowledge graphs uncovers new avenues for delivering tailored user experiences and increasing customer engagement. Personalized recommendations leverage user data and behavioral patterns to suggest relevant products, services, or content based on individual preferences. The key characteristic of personalized recommendations is their ability to enhance user satisfaction, drive conversions, and foster brand loyalty through targeted marketing strategies. This approach is a beneficial choice for businesses looking to optimize customer engagement and retention metrics. However, challenges such as data privacy concerns and algorithm transparency need to be carefully managed to ensure ethical and effective implementation of personalized recommendation systems in knowledge graph frameworks.
Future Trends and Possibilities
In the realm of open source knowledge graphs, the anticipation and examination of future trends and possibilities hold paramount significance. As technology continues to evolve at a rapid pace, the intersection of knowledge graphs with emerging trends such as artificial intelligence (AI) integration and cross-domain knowledge sharing becomes increasingly essential. The dynamic nature of knowledge graphs necessitates continual adaptation to stay relevant in a landscape characterized by innovation and change. By staying attuned to future trends and possibilities, organizations can strategically position themselves to harness the full potential of open source knowledge graphs.
Evolution of Knowledge Graphs
AI Integration
Delving into the specifics of AI integration within the context of knowledge graphs, it becomes evident that the integration of artificial intelligence technologies plays a pivotal role in enhancing the capabilities and functionalities of knowledge graphs. AI integration empowers knowledge graphs to delve deeper into data analysis, pattern recognition, and predictive modeling, thereby augmenting decision-making processes and insights derivation. The key characteristic of AI integration lies in its ability to autonomously learn and adapt to data patterns, leading to more accurate and efficient outcomes. This feature makes AI integration a popular and beneficial choice within the realm of knowledge graphs, propelling organizations towards data-driven decision-making and innovation. Despite its advantages, challenges of AI integration in knowledge graphs include algorithmic biases and data privacy concerns, requiring careful consideration and mitigation strategies.
Cross-Domain Knowledge Sharing
Another significant aspect within the evolution of knowledge graphs is cross-domain knowledge sharing, which promotes the seamless exchange of information across diverse domains or disciplines. Cross-domain knowledge sharing enhances the interoperability and connectivity of knowledge graphs, enabling the amalgamation of insights and data from various sources. The key characteristic of cross-domain knowledge sharing is its capacity to break silos and foster collaboration among disparate domains, resulting in a holistic understanding of interconnected information. This characteristic renders cross-domain knowledge sharing a valuable and popular choice for organizations seeking to leverage diverse data assets for comprehensive insights generation. While advantageous in facilitating knowledge transfer and integrative analysis, challenges of cross-domain knowledge sharing include data standardization issues and compatibility complexities that need to be addressed for seamless integration.
Emerging Opportunities
Industry Disruption
Within the landscape of open source knowledge graphs, the concept of industry disruption emerges as a significant opportunity for organizations to innovate and transform traditional practices. Industry disruption entails the revolutionary impact of knowledge graphs on existing industry frameworks, leading to paradigm shifts in data management, decision-making processes, and customer interactions. The key characteristic of industry disruption lies in its potential to challenge conventional norms and catalyze organizational evolution towards more agile and data-centric operations. This characteristic positions industry disruption as a beneficial and popular choice for enterprises seeking competitive differentiation and market leadership through disruptive innovation. While advantageous in fostering organizational agility and competitive edge, challenges of industry disruption may include resistance to change and operational realignment requirements that necessitate strategic change management approaches.
Data Ecosystem Expansion
Expanding the data ecosystem through the integration of open source knowledge graphs offers organizations a transformative opportunity to enrich their data landscapes and foster enhanced collaboration. Data ecosystem expansion involves the seamless integration of knowledge graphs with existing data repositories, platforms, and systems to facilitate comprehensive data utilization and interconnected insights discovery. The key characteristic of data ecosystem expansion is its ability to create a unified data fabric that enables cross-functional data access, analysis, and utilization, driving synergies and knowledge discovery across organizational boundaries. This characteristic establishes data ecosystem expansion as a beneficial and popular choice for enterprises aiming to break data silos and augment data-driven decision-making capabilities. While advantageous in promoting data democratization and organizational transparency, challenges of data ecosystem expansion may include data governance complexities and cybersecurity risks that demand robust data management frameworks and security measures.