Unveiling the Real AI Applications: A Comprehensive Analysis
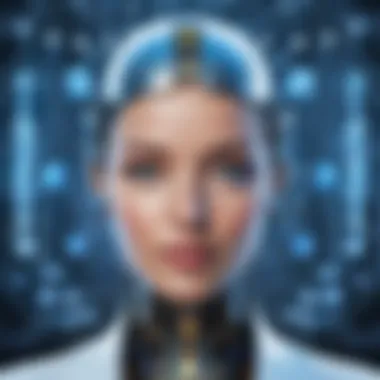
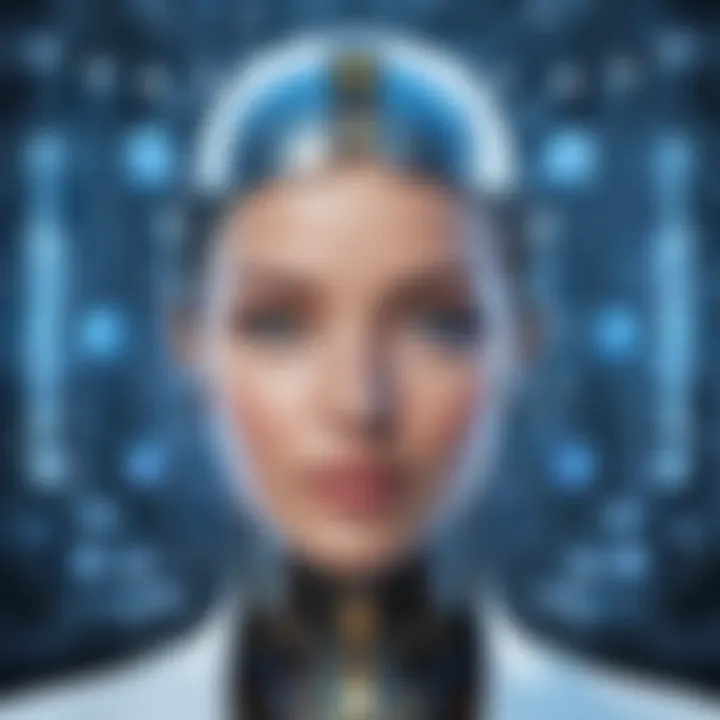
Latest Technological Innovations
In the realm of real AI applications, keeping abreast of the latest technological innovations is paramount to staying competitive. The landscape is ever-evolving, with continuous advancements reshaping industries. From state-of-the-art machine learning algorithms to cutting-edge deep neural networks, staying informed about these developments is vital. The dynamic nature of technological progress necessitates a deep dive into the intricacies of these innovations.
Startup Spotlight
Featured in this comprehensive guide is a startup that exemplifies the potential and challenges within the real AI application domain. This startup has navigated the intricate landscape of high-tech innovations, offering a unique perspective on success and resilience. The journey of this startup sheds light on the trials and triumphs that accompany venturing into the world of AI applications. By understanding their path to success and the obstacles they overcame, valuable insights emerge for both seasoned entrepreneurs and newcomers in the industry.
Tech Industry Trends
Unveiling the current trends in the tech industry is crucial for grasping the trajectory of real AI applications. By examining the trends shaping the high-tech sector, a comprehensive view of industry dynamics is revealed. Comparative analyses of various companies' approaches to these trends further enhance understanding. Moreover, predicting future trends and their potential impacts offers strategic foresight for stakeholders navigating the rapidly evolving tech landscape.
Investment Opportunities
Exploring investment prospects in high-tech and startup companies unveils a realm of opportunities for savvy investors. Understanding the key factors influencing investment decisions in the tech industry is instrumental in making informed choices. Delving into viable startups seeking funding provides a glimpse into the potential for growth and innovation. By analyzing these opportunities, investors can position themselves advantageously in the dynamic world of real AI applications.
Entrepreneurship Insights
Navigating the challenges of starting a high-tech business requires strategic insights and entrepreneurial acumen. Success in the fast-paced tech industry hinges on effective strategies tailored to the demands of innovation and competition. Aspiring entrepreneurs can benefit from valuable tips for thriving in this dynamic sector. By synthesizing firsthand experiences and expert advice, this section equips entrepreneurs with the knowledge essential for embarking on their high-tech ventures successfully.
Introduction
In this article, we embark on a thorough exploration of real AI applications, unraveling their intricate workings, advantages, and impacts on various industries. The realm of technology is constantly evolving, and grasping the essence of real AI apps is vital for understanding the future landscape of innovation and automation. By dissecting machine learning algorithms, deep neural networks, and natural language processing, we aim to provide a detailed account of the potential these applications hold.
Defining Real AI App
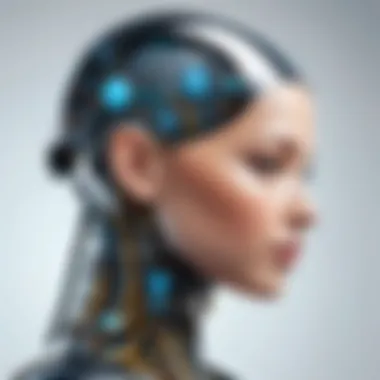
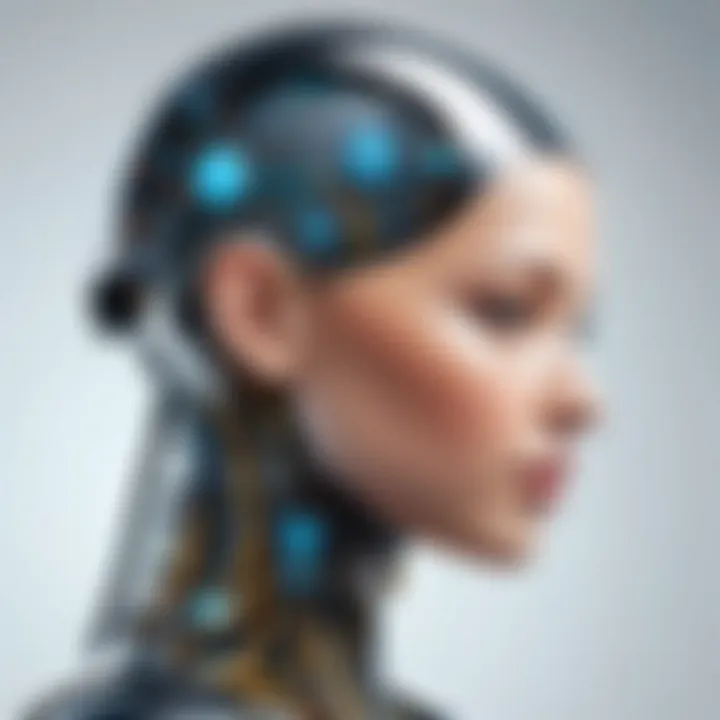
When we refer to real AI applications, we are delving into the practical implementation of artificial intelligence in various contexts. These applications are designed to simulate human intelligence and perform tasks that typically require human cognition. Real AI apps go beyond theoretical concepts and aim to provide tangible solutions to real-world problems, ushering in a new era of technological advancement and efficiency.
Significance of Real AI Applications
The significance of real AI applications cannot be overstated in today's digital landscape. These advanced technologies have revolutionized industries by streamlining processes, enhancing decision-making capabilities, and optimizing performance. Real AI applications offer unparalleled opportunities for innovation, efficiency, and competitiveness, shaping the way organizations operate and interact with data. Understanding the significance of these applications is crucial for staying ahead in the rapidly evolving tech-driven world.
Overview of Artificial Intelligence
Artificial intelligence serves as the foundation for real AI applications, driving innovation and transformation across various sectors. By mimicking human cognitive functions, AI systems can analyze vast amounts of data, identify patterns, and make informed decisions. This overview provides a glimpse into the diverse subsets of AI, including machine learning, deep learning, and neural networks, illustrating the breadth and depth of capabilities that underpin real AI applications.
Fundamentals of Real AI Apps
In this section, we delve into the pivotal aspects of Fundamentals of Real AI Apps. Understanding the core principles and functionalities of real AI applications is paramount in gaining a comprehensive grasp of this innovative technology landscape. Fundamentals of Real AI Apps serve as the building blocks for all advanced AI systems, encompassing a wide array of machine learning algorithms, deep neural networks, and natural language processing techniques. The significance of this topic lies in its role as the foundation upon which the practical applications of AI thrive.
Machine Learning Technologies
Supervised Learning
Supervised Learning plays a crucial role in the realm of AI applications. It involves training a model on a labelled dataset, where the algorithm learns to map input data to the correct output. The key characteristic of Supervised Learning is the presence of a supervisor or teacher who provides the algorithm with correct output for given inputs. This approach is advantageous as it allows for precise predictions based on historical data. However, its dependence on labelled data and the risk of overfitting are notable considerations in this article.
Unsupervised Learning
Unsupervised Learning stands out for its ability to uncover hidden patterns in data without the need for labelled outputs. This approach is beneficial for discovering inherent structures within datasets and detecting anomalies or outliers. The unique feature of Unsupervised Learning lies in its adaptability to unlabelled data, offering flexibility in exploring uncharted territories. Despite its advantages in data exploration, the challenge of interpreting results without clear labels poses a significant consideration in this article.
Reinforcement Learning
Reinforcement Learning introduces a different paradigm by enabling an agent to learn through the consequences of its actions. The key characteristic of Reinforcement Learning is the continuous feedback loop between the agent and its environment, where rewards and punishments shape the learning process. This method is advantageous for tasks requiring decision-making and sequential actions. However, the trade-off between exploration and exploitation poses a complex challenge in this article.
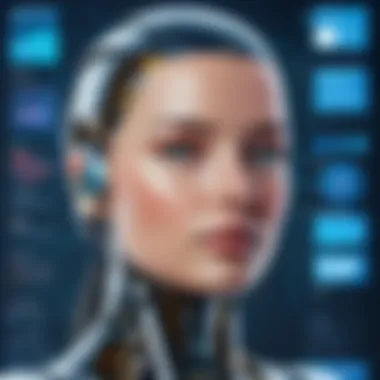
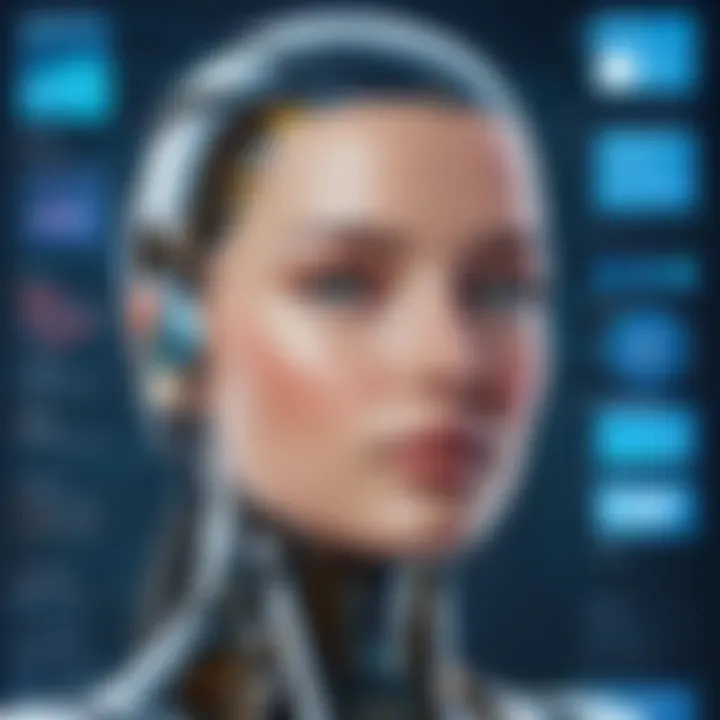
Deep Neural Networks
Convolutional Neural Networks
Convolutional Neural Networks are essential in various AI applications, particularly in image and pattern recognition tasks. The key characteristic of CNNs is their ability to automatically extract features from input data, making them popular choices for computer vision applications. The unique feature of Convolutional Neural Networks lies in their hierarchical structure, allowing for the detection of complex patterns. Despite their efficiency in image processing tasks, CNNs may face challenges in handling spatial variance and high computational requirements.
Recurrent Neural Networks
Recurrent Neural Networks are prominent for tasks involving sequential data, such as time series analysis and natural language processing. The key characteristic of RNNs is their recurrent connections that enable information persistence over time. This feature makes them beneficial for processing sequences of data with contextual dependencies. However, issues like vanishing gradients and difficulty in capturing long-range dependencies are considerations in this article.
Generative Adversarial Networks
Generative Adversarial Networks are innovative models that consist of two neural networks, the generator, and the discriminator, engaged in a unique adversarial training process. The key characteristic of GANs is their capability to generate synthetic data that resembles real data distribution. This approach is beneficial for tasks like image generation and data augmentation. However, challenges related to training stability and mode collapse are significant considerations in this article.
Applications of Real AI Apps
In this article, we delve into the crucial realm of real AI applications, unveiling their immense significance and impact across various industries. Real AI applications represent cutting-edge technology that leverages machine learning algorithms, deep neural networks, and natural language processing to revolutionize different sectors. Understanding the applications of real AI apps is paramount in comprehending how technology is reshaping our world today. By exploring the functionalities and benefits of real AI applications, we gain invaluable insights into the future of artificial intelligence.
Healthcare Industry
Diagnosis Assistance
Delving into the intricacies of diagnosis assistance within the healthcare industry uncovers a pivotal aspect of real AI applications. Diagnosis assistance utilizes advanced algorithms to analyze medical data, assisting healthcare professionals in accurate and timely diagnoses. One key characteristic of diagnosis assistance is its ability to process large volumes of information swiftly, leading to faster and more precise medical assessments. The unique feature of diagnosis assistance lies in its potential to reduce human error and provide consistent diagnostic results. While offering significant advantages in enhancing healthcare outcomes, challenges such as data privacy concerns and algorithmic biases must be carefully addressed in the integration of diagnosis assistance in the healthcare sector.
Treatment Personalization
The personalized treatment approach facilitated by AI technologies marks a revolutionary advancement in healthcare. Treatment personalization tailors medical interventions to individual patient profiles, optimizing therapeutic outcomes and patient experiences. A significant characteristic of treatment personalization is its adaptability to cater to specific patient needs, ensuring customized care plans. This tailored approach fosters improved patient adherence and enhances treatment effectiveness. However, considerations about data security and ethical implications arise with the integration of personalized treatment solutions in healthcare settings.
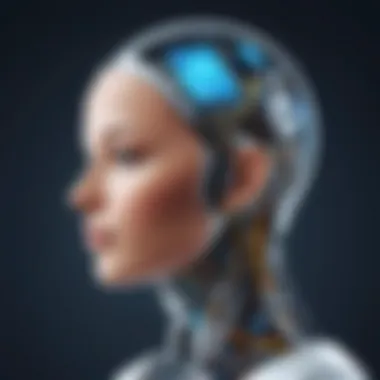
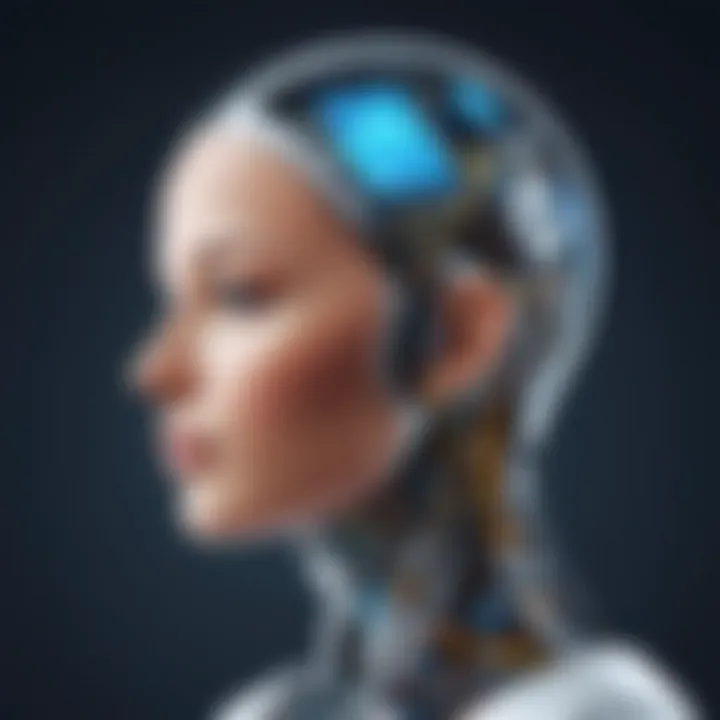
Drug Discovery
The realm of drug discovery is being reshaped by AI innovations, streamlining the identification and development of novel pharmaceuticals. By leveraging deep neural networks and machine learning algorithms, AI enables accelerated drug discovery processes, potentially leading to groundbreaking treatments. The key characteristic of AI-driven drug discovery is its ability to analyze vast amounts of chemical and biological data to identify promising drug candidates efficiently. The unique feature of this approach lies in its potential to reduce time and resource expenses traditionally associated with pharmaceutical research. While offering immense advantages in advancing healthcare treatments, ensuring regulatory compliance and maintaining ethical standards are crucial considerations in adopting AI for drug discovery.
Challenges and Ethical Considerations
In this segment of the article, we delve into the crucial facet of Challenges and Ethical Considerations surrounding real AI applications. It is imperative to address these aspects as AI technologies become more prevalent in various industries. One key element to consider is the potential impact on data privacy. As AI systems rely on vast amounts of data for training and decision-making, there arises a significant concern regarding the protection of sensitive information. Ensuring robust data privacy measures is essential to maintain trust and compliance with regulations. Additionally, Algorithmic Bias poses a substantial challenge in AI applications. The inherent biases within training data or design frameworks can lead to discriminatory outcomes, which undermines the ethical integrity of the technology. Mitigating algorithmic bias requires careful monitoring, and corrective actions to promote fairness and inclusivity. Moreover, Transparency in AI Decision-making emerges as a pivotal consideration. The opaqueness of AI algorithms and decision processes raises questions about accountability and trustworthiness. By enhancing transparency in AI systems, stakeholders can better understand and scrutinize the reasoning behind automated decisions, fostering ethical and accountable AI practices.
Data Privacy Concerns
Data privacy concerns represent a critical component within real AI applications. With the proliferation of AI technologies utilizing personal data for analysis and decision-making, safeguarding individuals' privacy is paramount. The collection, storage, and utilization of sensitive information raise ethical dilemmas regarding consent, transparency, and control over data. Implementing robust data encryption, access controls, and anonymization techniques are essential safeguards to protect privacy rights. Organizations must adhere to data protection regulations and industry best practices to prevent unauthorized access or misuse of confidential data.
Algorithmic Bias
The issue of Algorithmic Bias looms large over the landscape of AI applications. Biases inherent in training data or algorithmic decision-making processes can perpetuate discrimination and inequality in outcomes. Addressing algorithmic bias requires proactive measures such as diversity in dataset collection, bias detection tools, and fairness metrics integration. By identifying and mitigating biases at every stage of AI development, stakeholders can promote fairness, equity, and social responsibility within AI applications.
Transparency in AI Decision-making
Transparency plays a pivotal role in fostering trust and accountability in AI decision-making processes. Providing transparent explanations of how AI systems reach conclusions or recommendations is essential for users to understand the rationale behind automated decisions. Implementing interpretability techniques, such as model visualization or explanation mechanisms, can enhance the transparency of AI algorithms. By promoting openness and clarity in AI decision-making, organizations can build credibility and user acceptance, driving ethical and responsible AI practices.
Future Prospects of Real AI Apps
Advancements in AI Research
As we navigate the intricate world of artificial intelligence, the advancements in AI research stand out as a beacon of innovation and progress. This subsection delves into the cutting-edge developments and breakthroughs that are propelling the field of AI forward. From novel algorithms to enhanced computing capabilities, AI research is at the forefront of driving technological innovation. By exploring the latest trends and discoveries in AI research, we gain a comprehensive understanding of the evolving landscape of artificial intelligence.
Integration of AI in Everyday Life
The integration of AI in everyday life marks a significant paradigm shift in how we engage with technology on a daily basis. From virtual assistants to personalized recommendations, AI has seamlessly woven itself into the fabric of our modern world. This subsection explores the various ways in which AI is integrated into our daily lives, transforming industries, products, and services. By examining the impact of AI integration on different facets of society, we can appreciate the transformative power of artificial intelligence.
Socio-economic Implications
The socio-economic implications of AI reverberate across various sectors, shaping the way we work, communicate, and live. This subsection delves into the multifaceted impact of AI on society, economy, and employment. From job automation to economic growth, AI's influence extends far and wide, presenting both opportunities and challenges. By unraveling the socio-economic implications of AI, we can navigate the complex interplay between technology and society, paving the way for a more informed and sustainable future.