Unlocking Innovation: The Impact of Open Source DataOps Tools on High-Tech and Startups
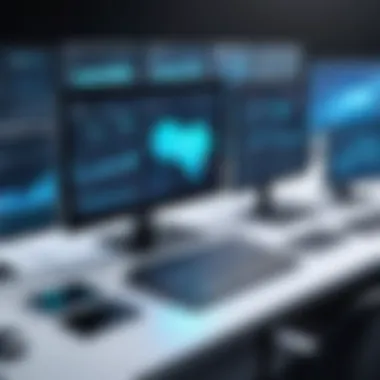
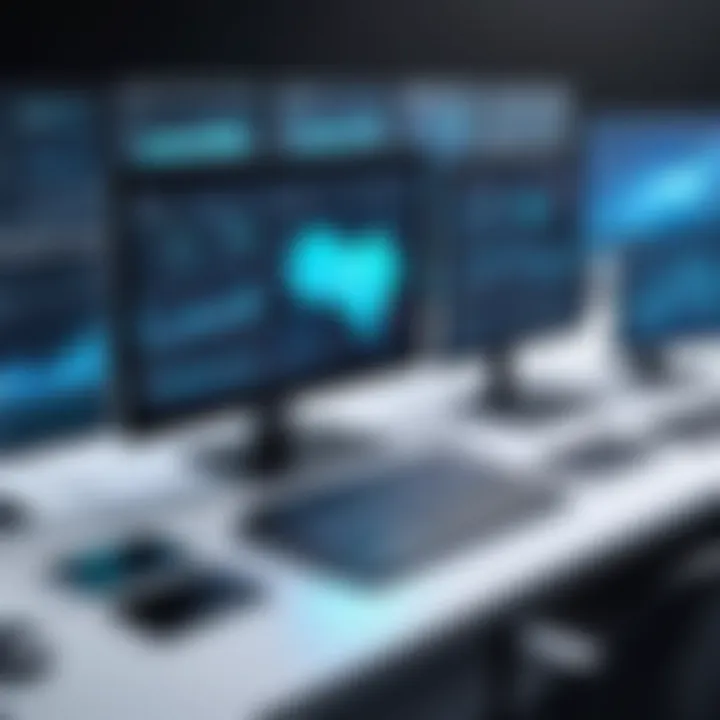
Latest Technological Innovations
Open-source Data Ops tools have swiftly emerged as a game-changer in the high-tech and startup domains. These tools represent the zenith of technological advancement, revolutionizing the way data is managed and optimized. By dissecting complex data operations into streamlined processes, these tools have resoundedly boosted productivity and efficiency for companies embracing them. The ramifications of incorporating open-source DataOps tools in organizations are profound, showcasing a paradigm shift towards innovation and accelerated growth.
Startup Spotlight
In the bustling landscape of startups, one company stands out as a beacon of success through the judicious application of open-source Data Ops tools. The featured startup, represented through its fascinating profile, exemplifies how leveraging these tools can pave the way for monumental achievements. From humble beginnings to scaling obstacles, the startup's journey encapsulates the challenges faced by many in the competitive industry. By navigating the complexities of data management with open-source tools, this startup triumphed over adversities, firmly establishing its position in the high-tech realm.
Tech Industry Trends
A holistic examination of current trends dominating the high-tech sphere reveals a tapestry of innovation and competition. Various companies' divergent approaches to technological advancements underscore the dynamic nature of the industry. The juxtaposition of these strategies offers valuable insights into successfully navigating the swiftly evolving tech landscape. Moreover, predictions on future trends propound intriguing possibilities, shaping the future trajectory of the high-tech domain and its myriad stakeholders.
Investment Opportunities
Delving into investment prospects within high-tech and startup companies uncovers a spectrum of opportunities for discerning investors. Analyzing key factors that define sound investment practices aids in strategizing for optimal returns in this ever-evolving market. Shedding light on promising startups seeking funding illuminates a pathway for potential investors to partake in groundbreaking ventures, fostering mutual growth and innovation within the tech ecosystem.
Entrepreneurship Insights
Navigating the labyrinth of launching and scaling a high-tech business demands a blend of tenacity, creativity, and resilience. Unveiling strategies tailored for aspiring entrepreneurs embarks on a journey of enlightenment on how to navigate the intricate nuances of the fast-paced tech industry. By distilling invaluable tips and insights, this section acts as a beacon for entrepreneurs seeking to carve a niche in the competitive high-tech milieu.
Introduction
In the fast-paced realms of high-tech and startups, the utilization of open-source Data Ops tools has emerged as a transformative force, reshaping traditional data management paradigms. This article embarks on a compelling journey to uncover the profound implications of incorporating these agile tools within such dynamic environments. By delving into the intricate interplay between DataOps methodologies and the ever-evolving tech landscape, we aim to unveil the multifaceted dimensions that underpin the pivotal role of these tools in propelling operational efficiencies and catalyzing innovation.
The significance of this exploration lies in its capacity to unearth a trove of possibilities that lie at the intersection of open-source technologies and data-driven decision-making processes. From exalting the virtues of streamlined data operations to illuminating the nuanced benefits that these tools engender, each facet of this discourse is imbued with tangible implications for stakeholders across diverse industry verticals, particularly those vested in the high-tech and startup sectors.
At the crux of this narrative, one finds a compelling exposition on how open-source Data Ops tools serve as catalysts for organizational metamorphosis, transcending mere digitization to orchestrate a symphony of data orchestration that harmonizes seamlessly with strategic objectives. By elucidating the symbiotic relationship between open-source ethos and digital transformation imperatives, this article endeavors to serve as a north star for digital pioneers navigating the uncharted waters of contemporary technological landscapes. The unfolding sections will meticulously dissect the operative dynamics of DataOps tools, offering a granular understanding of their transformative potential and the strategic advantages they confer.
Understanding DataOps
Data Ops forms a critical aspect of navigating the dynamic landscape of data management within high-tech and startup sectors, proving instrumental in optimizing operational efficiencies and fostering a culture of innovation. By comprehensively understanding DataOps, organizations can streamline their data processes, drive informed decision-making, and achieve unprecedented growth and scalability. The key elements of DataOps revolve around automation, collaboration, and agility, offering multifaceted advantages that cater to the evolving needs of modern businesses.
Defining DataOps
Data Ops, a fusion of data and operations, encapsulates a methodology aimed at streamlining and optimizing data analytics and deployment processes throughout an organization's data lifecycle. It emphasizes collaboration and communication between data engineers, data scientists, and other stakeholders to ensure seamless data operations. Defined by its emphasis on continuous integration and deployment, DataOps seeks to eliminate silos and enhance cross-functional teamwork and communication, ultimately driving actionable insights and innovation within enterprises.
Principles of DataOps
Automation
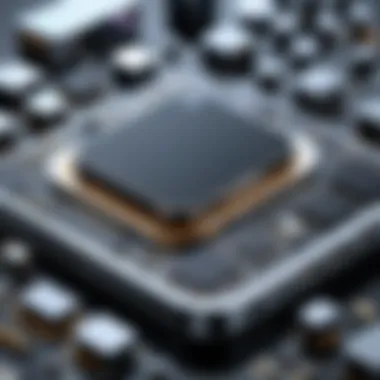
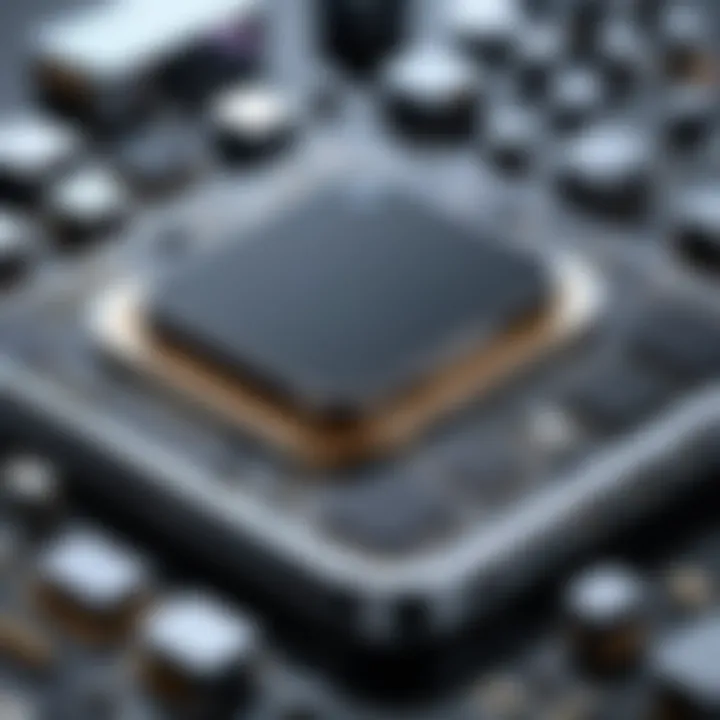
Automation in Data Ops revolves around the integration of technologies and processes to automate repetitive tasks, reduce manual intervention, and accelerate the pace of data operations. This key characteristic of automation ensures consistency, reduces error rates, and enhances operational efficiency within data management workflows. The unique feature of automation lies in its ability to orchestrate complex data pipelines, schedule tasks, and monitor workflows in real-time, enabling organizations to streamline their data operations effectively. While automation offers unparalleled benefits in terms of speed and efficiency, challenges such as algorithm biases and overreliance on automated processes must be carefully considered within the context of DataOps initiatives.
Collaboration
Collaboration serves as a fundamental principle of Data Ops, fostering a culture of knowledge sharing, innovation, and cross-functional collaboration within organizations. This key characteristic of collaboration emphasizes the importance of breaking down departmental silos, encouraging open communication, and promoting interdisciplinary teamwork to drive organizational success. The unique feature of collaboration lies in its ability to synchronize efforts between data teams, IT departments, and business stakeholders, ensuring alignment towards common goals and objectives. While collaboration enhances transparency, creativity, and problem-solving capacities, challenges such as communication barriers and conflicting priorities may hinder collaborative efforts in DataOps implementations.
Agility
Agility within Data Ops pertains to the ability to adapt quickly to changing data landscapes, market trends, and business requirements, enabling organizations to pivot, innovate, and respond effectively in dynamic environments. This key characteristic of agility emphasizes flexibility, responsiveness, and iterative development cycles, empowering teams to iterate on data processes rapidly and incorporate feedback seamlessly. The unique feature of agility lies in its capacity to drive continuous improvement, accelerate time-to-market, and enhance decision-making processes by enabling organizations to adjust strategies in real-time based on evolving data insights. While agility offers advantages in terms of adaptability and competitiveness, challenges such as resistance to change and scalability constraints must be navigated strategically within DataOps frameworks.
Benefits of DataOps
Improved Data Quality
Improved data quality serves as a cornerstone benefit of Data Ops, enriching data insights, decision-making processes, and overall operational efficiency within organizations. This specific aspect of improved data quality underscores the importance of data cleanliness, consistency, and accuracy in driving actionable insights and strategic outcomes. The key characteristic of improved data quality lies in its role in enhancing trust, reliability, and relevancy of data assets, ensuring that data-driven decisions align with organizational goals and objectives. The unique feature of improved data quality centers on data governance, data cleansing, and data enrichment strategies, mitigating risks associated with poor data quality and empowering organizations with reliable data assets for informed decision-making. While improved data quality offers advantages in terms of reliability and decision accuracy, challenges such as data integration complexities and data standardization issues must be addressed proactively within DataOps frameworks.
Faster Insights
Faster insights represent a pivotal advantage of Data Ops, empowering organizations to derive actionable insights from data rapidly and make informed decisions in real-time. This specific aspect of faster insights highlights the importance of speed, agility, and responsiveness in extracting value from data assets and leveraging data-driven strategies effectively. The key characteristic of faster insights lies in its capacity to reduce time-to-insight, enable real-time analytics, and support rapid decision-making processes, enhancing organizational agility and competitiveness. The unique feature of faster insights focuses on data processing optimizations, real-time analytics tools, and predictive modeling techniques, facilitating organizations in capitalizing on opportunities, mitigating risks, and optimizing operational performance with timely insights. While faster insights offer benefits in terms of responsiveness and competitive advantage, challenges such as data latency and data accuracy issues must be monitored and rectified within DataOps environments.
Scalability
Scalability serves as a critical benefit of Data Ops, enabling organizations to expand, adapt, and evolve their data operations seamlessly in response to growing business demands and industry transformations. This specific aspect of scalability emphasizes the capacity to accommodate increasing data volumes, user loads, and analytical complexities without compromising performance or efficiency. The key characteristic of scalability lies in its ability to support elastic data architectures, distributed computing frameworks, and cloud-native infrastructure, facilitating organizations in scaling their data processes horizontally and vertically based on evolving requirements. The unique feature of scalability encompasses auto-scaling mechanisms, data partitioning strategies, and load balancing techniques, empowering organizations to achieve operational excellence, cost efficiencies, and performance optimization while maintaining data integrity and reliability. While scalability offers advantages in terms of flexibility and resource optimization, challenges such as data migration complexities and operational overheads must be managed diligently to ensure seamless scalability within DataOps implementations.
Role of Data
Ops in High-Tech Sector
In the fast-paced realm of high-tech, the role of Data Ops holds paramount importance. High-tech companies, driven by data-driven decision-making, rely heavily on efficient data management practices. DataOps, within this sector, acts as a linchpin by facilitating streamlined data operations, optimizing processes, and enhancing overall efficiency. The seamless integration of DataOps ensures that high-tech enterprises can unlock the full potential of their data assets. By leveraging DataOps principles like automation, collaboration, and agility, these companies can stay ahead in the competitive landscape, responding rapidly to market demands and implementing innovative solutions.
Enhancing Data Management
Enhancing data management within high-tech industries is a critical aspect to ensure operational success. Data Ops methodologies play a pivotal role in fortifying data management practices by enabling real-time data processing, active monitoring, and robust data governance frameworks. By centralizing data management processes and implementing automated workflows, high-tech firms can experience heightened data accuracy, reduced processing times, and improved accessibility to relevant information. DataOps not only streamlines data pipelines but also enhances data quality assurance protocols, making data management a seamless and efficient process within high-tech organizations.
Driving Innovation
Innovation stands at the core of high-tech sectors, driving breakthrough solutions and technological advancements continuously. Data Ops fuels this spirit of innovation by providing a structured framework for experimentation, data analysis, and iterative improvements. By embracing DataOps practices, high-tech companies create an environment conducive to collaboration, knowledge sharing, and rapid prototyping. This approach accelerates the innovation cycle, allowing enterprises to transform data insights into actionable strategies swiftly. Moreover, DataOps methodologies empower teams to test new ideas, validate hypotheses, and scale innovation initiatives efficiently, fostering a culture of continuous innovation within the high-tech landscape.
Ensuring Data Security
Data security remains a top priority in the high-tech sector due to the sensitive nature of proprietary information and customer data. Data Ops plays a crucial role in ensuring robust data security measures by implementing encryption standards, access controls, and data protection mechanisms throughout the data lifecycle. By integrating security protocols within data pipelines, high-tech organizations can safeguard against data breaches, unauthorized access, and potential cyber threats. DataOps not only enhances data security but also enforces compliance with industry regulations, building trust among stakeholders and maintaining the integrity of critical data assets within the high-tech ecosystem.
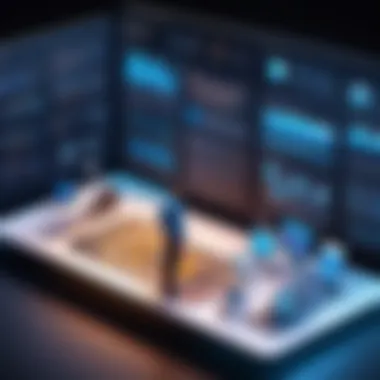
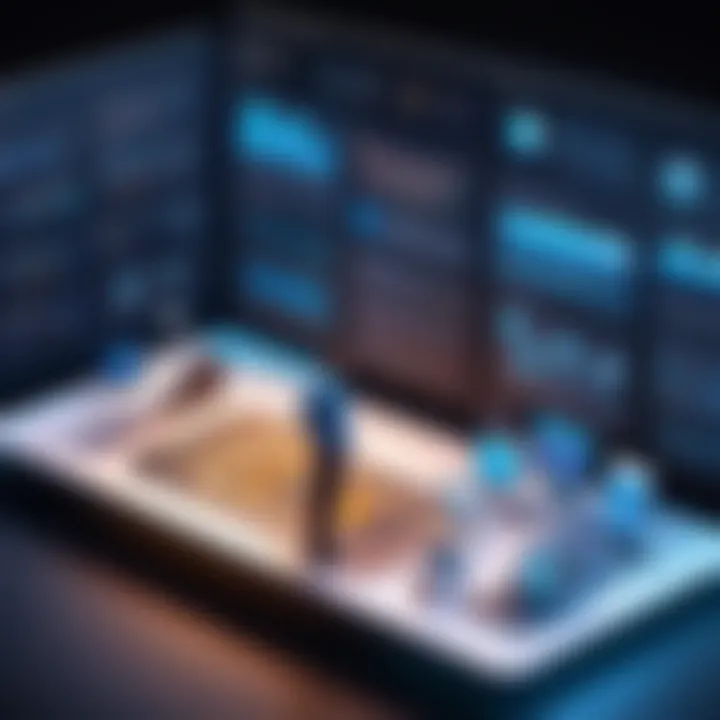
Open Source DataOps Tools
Open Source Data Ops tools play a pivotal role in revolutionizing data management processes within the high-tech and startup sectors. These tools act as a catalyst for enhancing operational efficiency, promoting collaboration, and fostering agility. By embracing Open Source DataOps tools, organizations can streamline their data operations, ensure higher data quality, gain quicker insights, and seamlessly scale their infrastructure while keeping costs in check.
Overview of Open Source Tools
Open Source Data Ops tools encompass an array of software solutions designed to optimize data workflows in a collaborative and automated environment. These tools leverage open-source frameworks and methodologies to offer cost-effective and customizable solutions tailored to meet the diverse needs of modern businesses. They empower enterprises to automate repetitive tasks, create data pipelines, and facilitate seamless data integration across different platforms and systems.
Popular Open Source Data
Ops Tools
Apache Airflow
Apache Airflow stands out as a prominent Open Source Data Ops tool renowned for its workflow automation capabilities. Its key strength lies in orchestrating complex data workflows in a scalable and programmable manner. This tool streamlines the process of scheduling, monitoring, and managing data pipelines, enabling users to achieve greater operational efficiency and flexibility. However, its learning curve and initial setup complexity may pose challenges for novice users.
DVC, another popular Open Source Data Ops tool, focuses on versioning and managing machine learning models and datasets. Its primary advantage lies in facilitating the reproducibility and traceability of experiments, ensuring that data scientists can track changes and collaborate effectively. DVC excels in simplifying the process of data versioning and integration within ML workflows, enhancing data quality control and experiment management. Yet, users should be aware of potential limitations in scalability for large datasets.
Prefect
Prefect emerges as a reliable Open Source Data Ops tool distinguished for its emphasis on pipeline orchestration and monitoring. It excels in providing a robust infrastructure for building, executing, and monitoring data workflows at scale, allowing users to streamline complex data processes and ensure reliable task execution. Prefect's intuitive design and extensive library of integrations make it a preferable choice for organizations seeking a flexible and scalable DataOps solution. However, users may encounter constraints related to the learning curve associated with advanced functionalities.
Comparison of Open Source vs. Proprietary Tools
When comparing Open Source Data Ops tools to proprietary ones, businesses face a critical decision-making process. While Open Source tools offer flexibility, transparency, and a thriving community for support and development, proprietary tools provide advanced features, dedicated support, and oftentimes, better integration capabilities. It's essential for organizations to weigh the pros and cons of each type of tool carefully, considering factors such as cost, customizability, security, and long-term scalability, to make an informed choice that aligns with their unique business requirements and objectives.
Integration of Data
Ops Tools in Startups
In the realm of high-tech and startup sectors, the integration of Data Ops tools plays a pivotal role in optimizing data operations, fostering efficiency, and driving innovation. Startups, in particular, can benefit immensely from incorporating these tools into their operations. By seamlessly integrating DataOps tools into their workflows, startups can streamline their data processes, enhance collaboration among team members, and accelerate the speed at which data is processed and analyzed.
This integration facilitates the automation of data pipelines, enabling startups to automate repetitive tasks, streamline data collection, and ensure data quality. By automating manual tasks, startups can significantly reduce the risk of human error and improve the efficiency and accuracy of their data operations. Moreover, the implementation of Data Ops tools allows startups to scale their data infrastructure as their business grows, ensuring that they can handle increasing volumes of data without compromising performance.
Streamlining Data Processes
Streamlining data processes is a critical aspect of integrating Data Ops tools in startups. By streamlining data processes, startups can establish efficient workflows that minimize bottlenecks, reduce processing times, and enhance data quality. This streamlined approach to data processing enables startups to access and analyze data more quickly, empowering decision-makers to make informed choices based on up-to-date and reliable information.

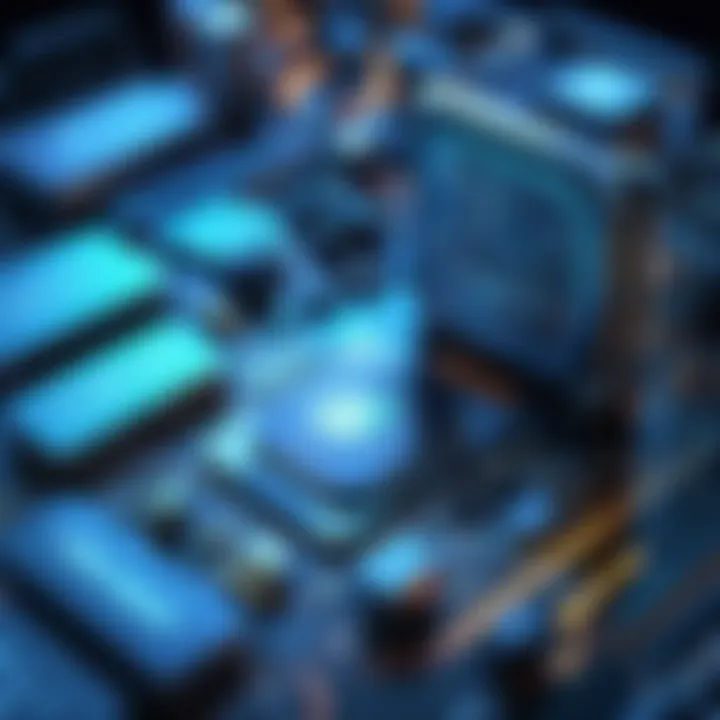
Implementing Data Ops tools for streamlining data processes involves optimizing data workflows, defining clear data pipelines, and removing any unnecessary steps in the data management process. By streamlining data processes, startups can improve their operational efficiency, reduce costs associated with manual data processing, and gain a competitive edge in their industry.
Enabling Data-Driven Decision Making
Enabling data-driven decision-making is another key benefit of integrating Data Ops tools in startups. By leveraging these tools, startups can harness the power of data to drive strategic decision-making processes. DataOps tools empower startups to collect, analyze, and interpret data more effectively, enabling them to identify trends, patterns, and insights that can inform critical business decisions.
Data-driven decision-making allows startups to make informed choices based on data-driven insights rather than intuition or guesswork. By enabling data-driven decision-making, Data Ops tools help startups mitigate risks, seize opportunities, and optimize their business processes for maximum efficiency and effectiveness.
Facilitating Growth and Scalability
Facilitating growth and scalability is a crucial aspect of integrating Data Ops tools in startups. As startups expand and evolve, they must be able to scale their data operations to accommodate growing data volumes and increasing analytical demands. DataOps tools provide startups with the scalability they need to grow their data infrastructure in a flexible and efficient manner.
By facilitating growth and scalability, Data Ops tools enable startups to expand their data capabilities without incurring significant costs or delays. These tools allow startups to scale their data operations seamlessly, ensuring that they can analyze and utilize data effectively as their business expands. Moreover, the scalability offered by DataOps tools positions startups for long-term success and sustainability in an increasingly data-driven business landscape.
Challenges and Considerations
In the ever-evolving landscape of high-tech and startup sectors, understanding and addressing challenges and considerations within Data Ops are paramount. By delving into the complexities of data privacy and compliance, resource constraints, and skill gaps in DataOps, organizations can proactively navigate obstacles and foster sustainable growth and innovation.
When we contemplate the realm of data privacy and compliance, a critical facet emerges. Ensuring that data handling aligns with regulatory frameworks and industry standards is not just a legal necessity but a foundational element of building trust with customers and partners. As high-tech and startups deal with vast amounts of sensitive information, such as user data and intellectual property, fostering a culture of data security and compliance becomes non-negotiable. Implementing robust data protection measures and staying abreast of evolving regulations both safeguard organizational integrity and mitigate the risks of data breaches and legal entanglements.
Conversely, the specter of resource constraints looms large in the realm of Data Ops. High-tech firms and startups are often racing against time and competition, striving to maximize output with limited resources. From financial constraints to manpower limitations, organizations face the challenge of optimizing data operations without overburdening existing infrastructures. Shrewd resource allocation, leveraging cost-effective solutions, and effectively scaling operations become imperative strategies for organizations looking to thrive in a resource-constrained environment. Balancing efficiency with expenditure, and innovation with pragmatism, becomes a delicate yet crucial dance for entities operating within these dynamic sectors.
Moreover, the pressing issue of skill gap in Data Ops requires deliberate attention and action. As technologies advance at breakneck speeds, the demand for skilled professionals well-versed in data management, analytics, and innovative technologies continues to outstrip supply. High-tech organizations and startups grapple with the challenge of sourcing, retaining, and upskilling talent proficient in DataOps. Investing in comprehensive training programs, fostering a culture of continuous learning, and creating pathways for skill development not only enhance organizational capabilities but also future-proof teams against the rapid evolution of technology. Bridging the skill gap in DataOps is not merely a matter of competitiveness but a strategic imperative for organizations seeking to stay ahead in the technological race.
Future Outlook
In the realm of utilizing open-source Data Ops tools within the high-tech and startup sectors, the future outlook is teeming with possibilities and advancements waiting to be harnessed. As technology continues to evolve at a rapid pace, the integration of cutting-edge tools like Apache Airflow, DVC, and Prefect is expected to revolutionize data operations further. The trajectory of DataOps in these sectors is deeply intertwined with the surge of data-driven decision-making and the constant quest for operational excellence.
Looking ahead, a key element that will shape the future outlook of Data Ops tools is their adaptability to emerging technologies. With artificial intelligence, machine learning, and big data analytics driving the industry forward, open-source DataOps tools must align with these trends to remain relevant and competitive. Moreover, the scalability and flexibility of these tools will be paramount as organizations strive to manage increasingly complex and vast datasets in real-time.
The benefits of embracing a forward-looking approach to Data Ops tools are manifold. Enhanced collaboration, streamlined workflows, and improved data governance are just a few aspects that will propel high-tech companies and startups towards greater operational efficiency and innovation. By staying abreast of the latest advancements in the field and adopting a future-oriented mindset, businesses can gain a significant edge in an increasingly data-centric landscape.
However, while the future of open-source Data Ops tools holds immense promise, certain considerations must be taken into account. As the volume and variety of data continue to expand exponentially, ensuring data security and compliance with regulatory standards will remain a critical concern. Addressing the skill gap in DataOps will also be crucial, necessitating continuous training and upskilling initiatives to empower employees with the necessary expertise to leverage these advanced tools effectively.
Ops tools in the high-tech and startup sectors is exceptionally bright, paving the way for unparalleled efficiency, innovation, and growth. By embracing the evolving landscape of data technologies and proactively addressing challenges, organizations can position themselves at the forefront of the digital revolution, driving success and sustainable development in the competitive global market.
Conclusion
The significance of the Conclusion section in this article on The Impact of Open Source Data Ops Tools in High-Tech and Startup Sectors cannot be overstated. It serves as the culmination of the intricate exploration of how the adoption of open-source DataOps tools revolutionizes data operations in these dynamic industries. By delving deep into the key elements, benefits, and considerations of DataOps tools, this conclusion encapsulates the essence of leveraging cutting-edge technology for enhancing operational efficiency and driving innovation.
One of the paramount elements underscored throughout this article is the transformative power of adopting open-source Data Ops tools in high-tech and startup sectors. These tools play a pivotal role in optimizing data management processes, streamlining operations, and facilitating agile decision-making. By emphasizing the importance of automation, collaboration, and agility, DataOps tools pave the way for organizations to achieve unprecedented levels of efficiency and productivity.
Furthermore, the benefits elucidated in the preceding sections underscore the immense value that open-source Data Ops tools bring to the table. From improving data quality and accelerating insights to enabling scalability and growth, these tools equip high-tech companies and startups with the competitive edge needed to thrive in a rapidly evolving landscape. The seamless integration of Apache Airflow, DVC, and Prefect showcases how innovative technologies can empower organizations to unlock their full potential.
When it comes to considerations, addressing challenges such as data privacy, compliance, resource constraints, and the skill gap in Data Ops is imperative for organizations aiming to harness the full potential of these tools. By strategically navigating these hurdles, companies can ensure a secure and compliant data environment while nurturing a skilled workforce capable of leveraging DataOps tools effectively.
In essence, the Conclusion section serves as a call to action for high-tech professionals, entrepreneurs, and investors to embrace the transformative capabilities of open-source Data Ops tools. By staying abreast of emerging trends, leveraging the right tools, and proactively addressing challenges, organizations can chart a course towards sustained growth, innovation, and success in the competitive landscape of high-tech and startup sectors.